Self-selective memristor-enabled in-memory search for highly efficient data mining
INFOMAT(2023)
摘要
Similarity search, that is, finding similar items in massive data, is a fundamental computing problem in many fields such as data mining and information retrieval. However, for large-scale and high-dimension data, it suffers from high computational complexity, requiring tremendous computation resources. Here, based on the low-power self-selective memristors, for the first time, we propose an in-memory search (IMS) system with two innovative designs. First, by exploiting the natural distribution law of the devices resistance, a hardware locality sensitive hashing encoder has been designed to transform the real-valued vectors into more efficient binary codes. Second, a compact memristive ternary content addressable memory is developed to calculate the Hamming distances between the binary codes in parallel. Our IMS system demonstrated a 168x energy efficiency improvement over all-transistors counterparts in clustering and classification tasks, while achieving a software-comparable accuracy, thus providing a low-complexity and low-power solution for in-memory data mining applications.
更多查看译文
关键词
in-memory search,self-selective memristor,similarity search,ternary content addressable memory
AI 理解论文
溯源树
样例
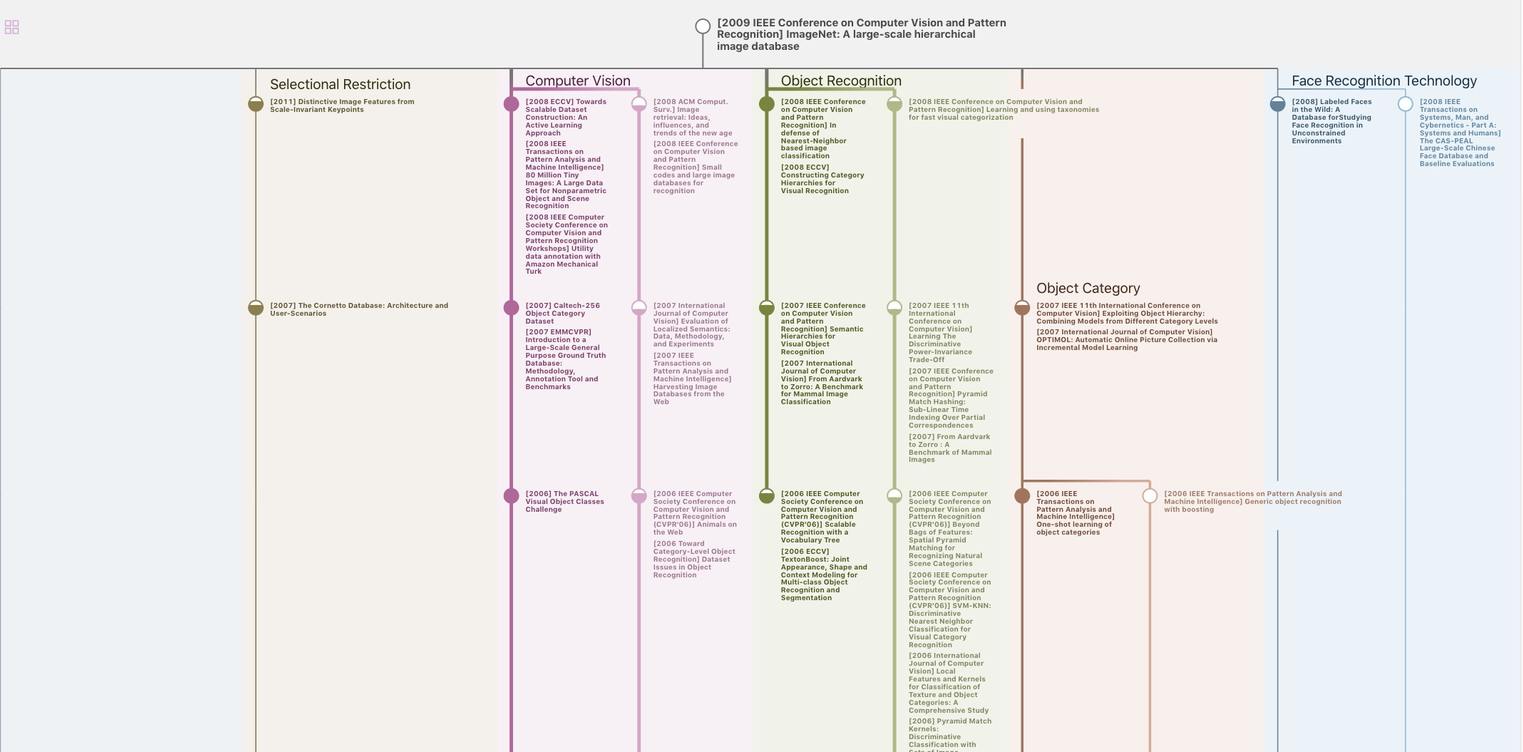
生成溯源树,研究论文发展脉络
Chat Paper
正在生成论文摘要