Cortex-inspired ensemble based network intrusion detection system
NEURAL COMPUTING & APPLICATIONS(2023)
摘要
With the invention of modern network technologies in recent decades, the exponential growth in wireless devices and their ease in wireless connectivity, network traffic is significantly increasing. Unluckily, this ease of connectivity is increasing the risk of network intrusion and exploitation of information. This vulnerability also provides opportunities for attackers to perform malicious activities, resulting in serious security threats such as DDoS, DoS, Brute Force, SQL Injection, Malware, and Phishing attack, thus creating the demand for an effective network intrusion detection system. The traditional approaches for identifying the attacks performed by an intruder are mostly packet analysis-based and thus cannot achieve good performance. Unluckily, inspection of a packet is not possible in the case of a high-speed and encrypted packet. Additionally, a packet is a small chunk of information as compared to flow thereby, extracting the nature (attack) of traffic for which a packet is being used is constrained. Considering the power of information processing in the human brain’s visual cortex, we propose a cortex-inspired ensemble-based network intrusion detection system (CI-EnsID) capable of detecting attacks or intrusions in a network flow. The proposed system consists of a multilayer feed-forward network that processes the information like an ensemble classification in addition to its cortex-like information processing. Moreover, the proposed system hybridizes contemporary unsupervised and supervised learning techniques. This system is to be installed in a cascade to analyze the network flow to identify malicious traffic resulting from network intruders. To evaluate the proposed system, it was tested on standard and publicly available KDDCup99 and CICIDS2017 datasets. The experimental results show that the proposed CI-EnsID outperforms the Naive Bayes, artificial neural network, logistic regression, decision tree, and random forest-based contemporary classification techniques.
更多查看译文
关键词
Machine learning,Neural network,Intrusion detection system (IDS),Computer network,Algorithms,Brain's visual cortex
AI 理解论文
溯源树
样例
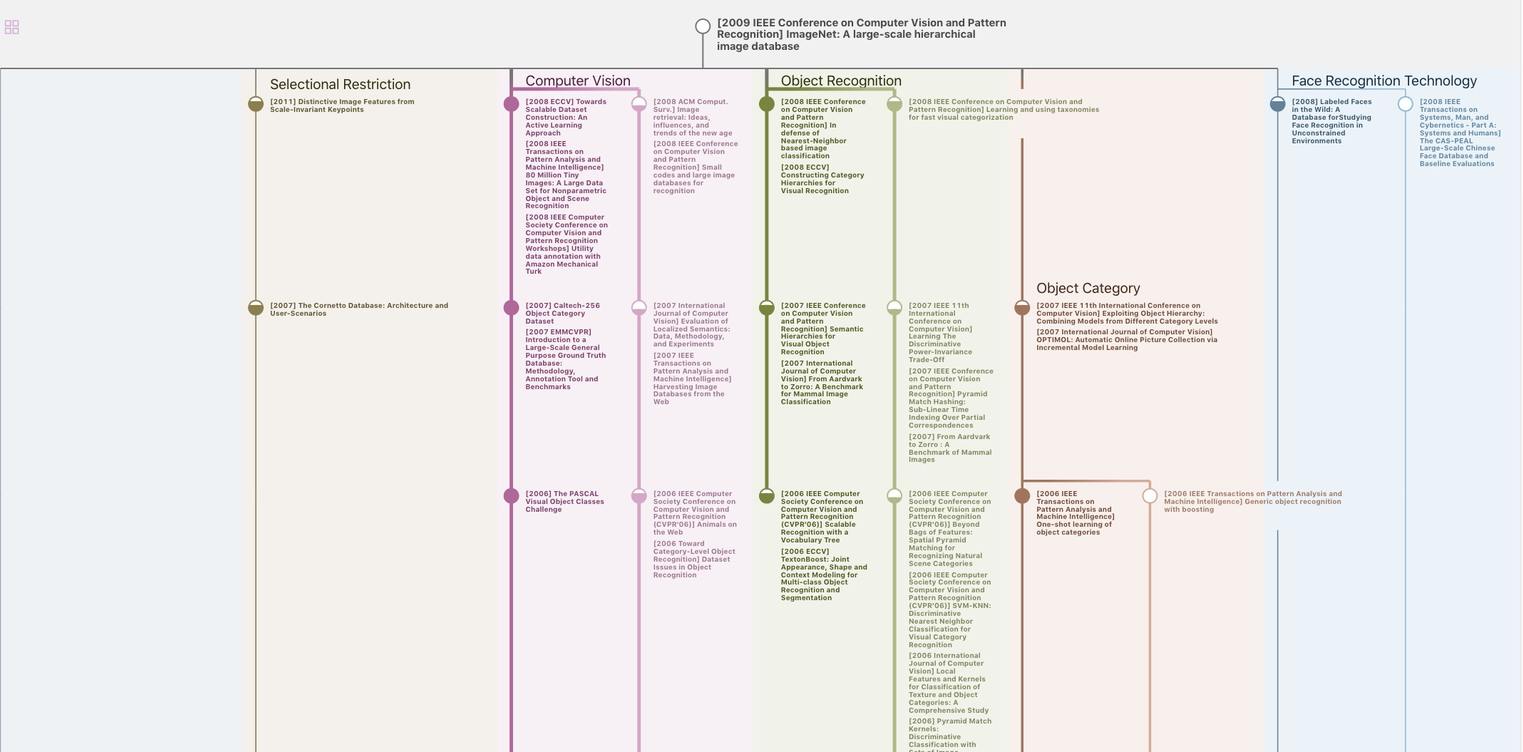
生成溯源树,研究论文发展脉络
Chat Paper
正在生成论文摘要