Artificial-intelligence-based molecular classification of diffuse gliomas using rapid, label-free optical imaging
NATURE MEDICINE(2023)
摘要
DeepGlioma, a multimodal deep learning approach for intraoperative diagnostic screening of diffuse glioma, trained on stimulated Raman histology and large-scale public genomic data, can predict molecular alterations for diffuse glioma diagnosis with high accuracy. Molecular classification has transformed the management of brain tumors by enabling more accurate prognostication and personalized treatment. However, timely molecular diagnostic testing for patients with brain tumors is limited, complicating surgical and adjuvant treatment and obstructing clinical trial enrollment. In this study, we developed DeepGlioma, a rapid (<90 seconds), artificial-intelligence-based diagnostic screening system to streamline the molecular diagnosis of diffuse gliomas. DeepGlioma is trained using a multimodal dataset that includes stimulated Raman histology (SRH); a rapid, label-free, non-consumptive, optical imaging method; and large-scale, public genomic data. In a prospective, multicenter, international testing cohort of patients with diffuse glioma (n = 153) who underwent real-time SRH imaging, we demonstrate that DeepGlioma can predict the molecular alterations used by the World Health Organization to define the adult-type diffuse glioma taxonomy (IDH mutation, 1p19q co-deletion and ATRX mutation), achieving a mean molecular classification accuracy of 93.3 +/- 1.6%. Our results represent how artificial intelligence and optical histology can be used to provide a rapid and scalable adjunct to wet lab methods for the molecular screening of patients with diffuse glioma.
更多查看译文
AI 理解论文
溯源树
样例
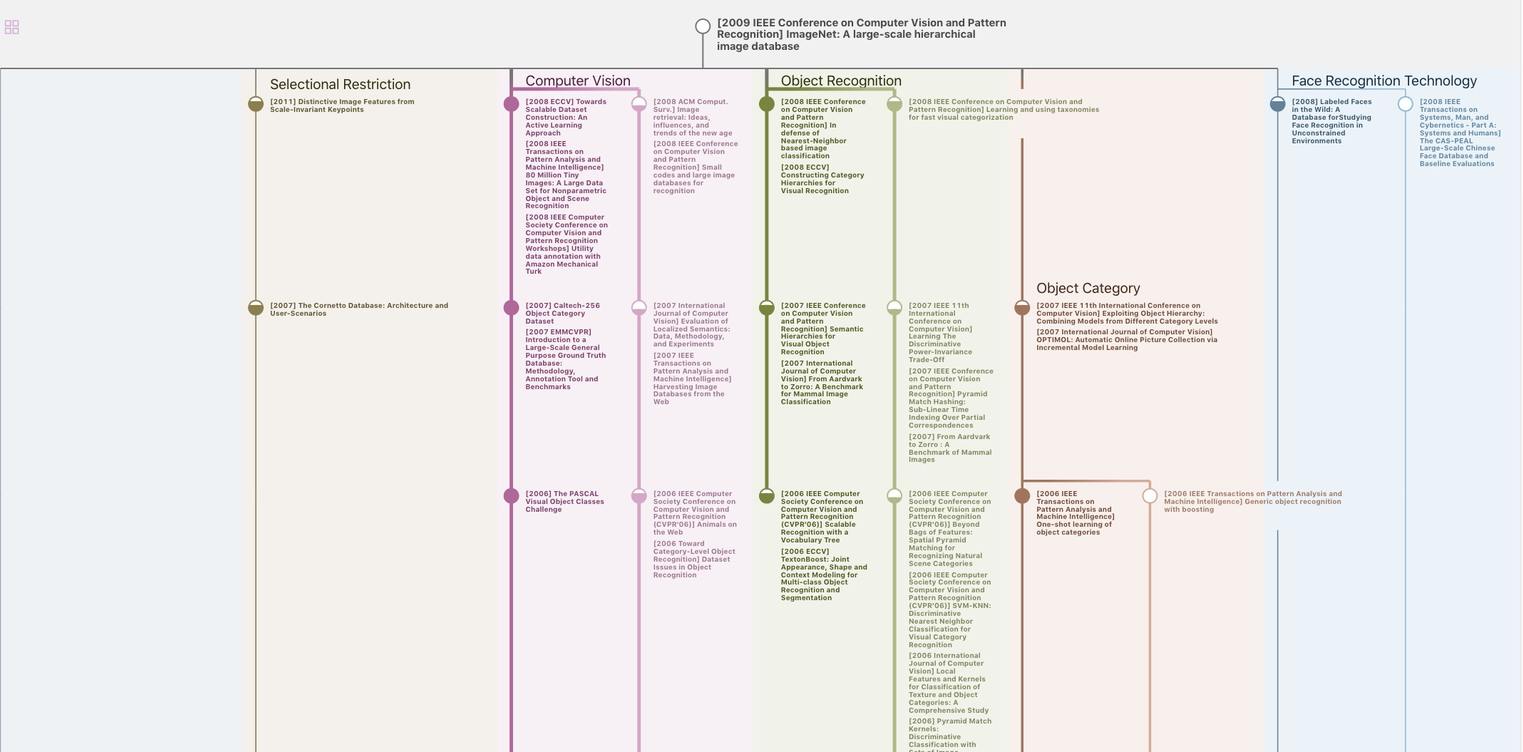
生成溯源树,研究论文发展脉络
Chat Paper
正在生成论文摘要