Predicting vehicle trajectory via combination of model-based and data-driven methods using Kalman filter
PROCEEDINGS OF THE INSTITUTION OF MECHANICAL ENGINEERS PART D-JOURNAL OF AUTOMOBILE ENGINEERING(2023)
摘要
Predicting future trajectories of surrounding vehicles accurately benefits the decision-making and motion-planning of autonomous vehicles (AVs). Physical-model-based prediction methods are generally accurate in short horizon prediction, but often fail in long-horizon prediction owing to the simplicity of the models and the complexity of the environment. Data-driven prediction methods can achieve promising performance in complex traffic scenarios and long-horizon prediction tasks. However, they might not be reliable on some occasions compared with model-based approaches, mainly owing to their uncertainty, poor interpretability and unsatisfying ability to learn the physical constraints of vehicles. In this paper, a novel cooperative vehicle trajectory prediction strategy exploiting the advantages of the two categories of prediction methods is proposed. The prediction results of the data-driven and kinematic models together with their uncertainties are described by the bivariate Gaussian model, and subsequently combined with an innovatively designed Kalman filter. The fusion strategy provides both physical and environmental constraints on the two types of methods for better prediction performance in both short and long horizons. Experiments applying different data-driven methods were carried out on the public BEV High-D dataset, as well as our specially-collected first-point-of-view highway dataset. The results indicate that the proposed method outperforms both the sub-models adopted in terms of prediction accuracy.
更多查看译文
关键词
Autonomous vehicles,vehicle trajectory prediction,kinematic model,deep neural network,Kalman filter
AI 理解论文
溯源树
样例
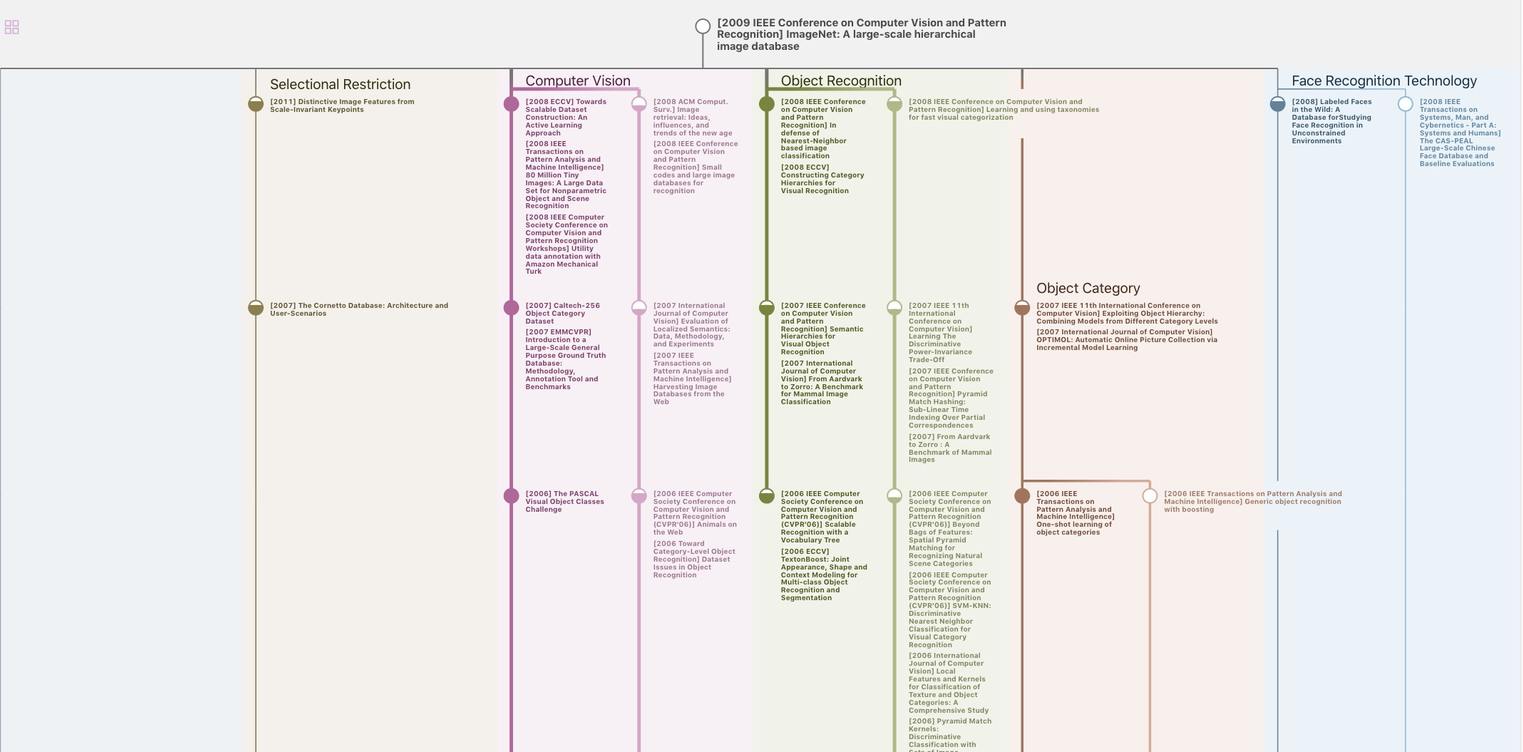
生成溯源树,研究论文发展脉络
Chat Paper
正在生成论文摘要