Intelligent forecasting model of stock price using neighborhood rough set and multivariate empirical mode decomposition
ENGINEERING APPLICATIONS OF ARTIFICIAL INTELLIGENCE(2023)
摘要
Intelligent forecasting model of stock price is an effective way to obtain ideal investment returns. Due to the impact of quantitative transactions, traditional forecasting methods face challenges and pressures. How to find a reliable forecasting model and improve the forecasting accuracy will be a scientific problem worthy of further discussion. This paper proposes an intelligent forecasting model of stock price based on neighborhood rough set (NRS) and multivariate empirical mode decomposition (MEMD) by means of the ideal of "granular computing"and "decomposition ensemble". Firstly, the multiscale permutation entropy (MPE) method is conducted to determine decision features, which can improve the stability of permutation entropy. Then, a new feature selection method, fusing mutual information (MI) and the NRS, is proposed to automatically capture and select the condition features from the stock market. Subsequently, aiming on revealing the more detailed feature information and maintaining relevance between features, the MEMD is utilized to simultaneously decompose all features. Finally, the decomposed features are inputted into the long short-term memory (LSTM) network to train the intelligent forecasting model and provide the forecasting results of stock price. The validity of our proposed model is assessed through the stocks of Shanghai and Shenzhen markets. The results show that our proposed intelligent forecasting model of stock price makes a beneficial attempt and discussion for the integration of "granular computing"and "decomposition ensemble". And it will provide scientific support and reference for investors' actual investment decisions.
更多查看译文
关键词
Stock price forecasting,Neighborhood rough set,Multivariate empirical mode decomposition
AI 理解论文
溯源树
样例
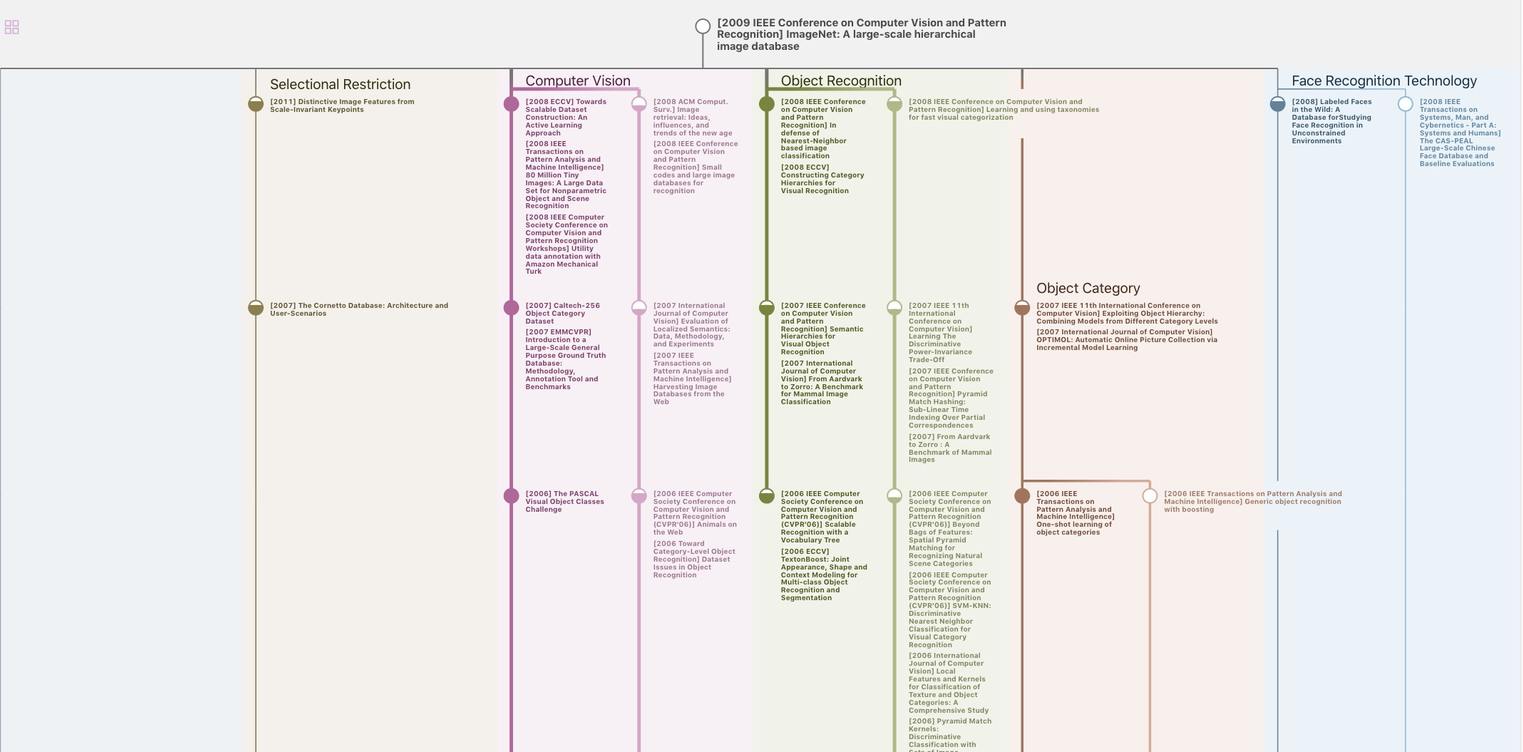
生成溯源树,研究论文发展脉络
Chat Paper
正在生成论文摘要