Graph-Based Change-Point Analysis
ANNUAL REVIEW OF STATISTICS AND ITS APPLICATION(2023)
摘要
Recent technological advances allow for the collection of massive data in the study of complex phenomena over time and/or space in various fields. Many of these data involve sequences of high-dimensional or non-Euclidean measurements, where change-point analysis is a crucial early step in understanding the data. Segmentation, or offline change-point analysis, divides data into homogeneous temporal or spatial segments, making subsequent analysis easier; its online counterpart detects changes in sequentially observed data, allowing for real-time anomaly detection. This article reviews a nonparametric change-point analysis framework that utilizes graphs representing the similarity between observations. This framework can be applied to data as long as a reasonable dissimilarity distance among the observations can be defined. Thus, this framework can be applied to a wide range of applications, from high-dimensional data to non-Euclidean data, such as imaging data or network data. In addition, analytic formulas can be derived to control the false discoveries, making them easy off-the-shelf data analysis tools.
更多查看译文
关键词
nonparametrics,graph-based tests,scan statistic,tail probability,high-dimensional data,network data,non-Euclidean data
AI 理解论文
溯源树
样例
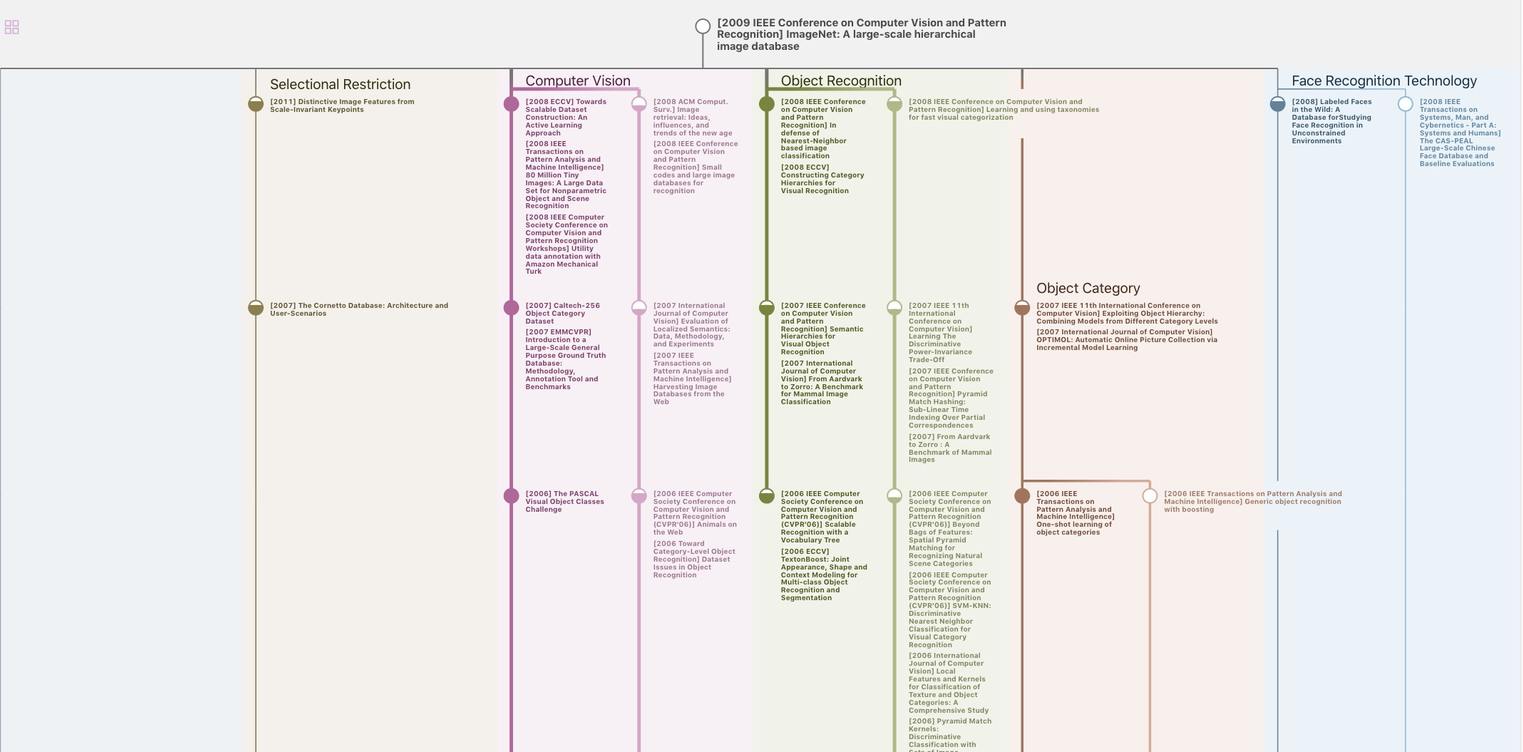
生成溯源树,研究论文发展脉络
Chat Paper
正在生成论文摘要