Adaptive traffic signal control using deep Q-learning: case study on optimal implementations
CANADIAN JOURNAL OF CIVIL ENGINEERING(2023)
摘要
Deep reinforcement learning has found great successes in addressing many challenging control problems; however, realworld implementations are still scarce if not non-existent. This is primarily due to three main challenges pertaining to the implementation, the stability, the optimal settings, and a lack of knowledge on methods that can be applied to field settings. This research attempts to address these issues with an adaptive simulation-based control framework proposed specifically for the training and evaluation. The control framework has implemented simulation models to conduct an extensive sensitivity analysis on the effects of key design variables, including rewarding schemes, state spaces, and model training parameters. The feasibility of transfer learning as a training strategy is also studied on scenarios with different layouts and different driver behavior models. Complex scenarios are also evaluated and used as test cases, including multiphase ring-and-barrier control and multi-intersection control. The research has contributed a significant amount of evidence on several critical design and implementation-related questions such as input representation, data (technology) requirements, training methods, and model transferability.Key words: deep reinforcement learning, traffic signal control, optimal control
更多查看译文
关键词
deep reinforcement learning,traffic signal control,optimal control
AI 理解论文
溯源树
样例
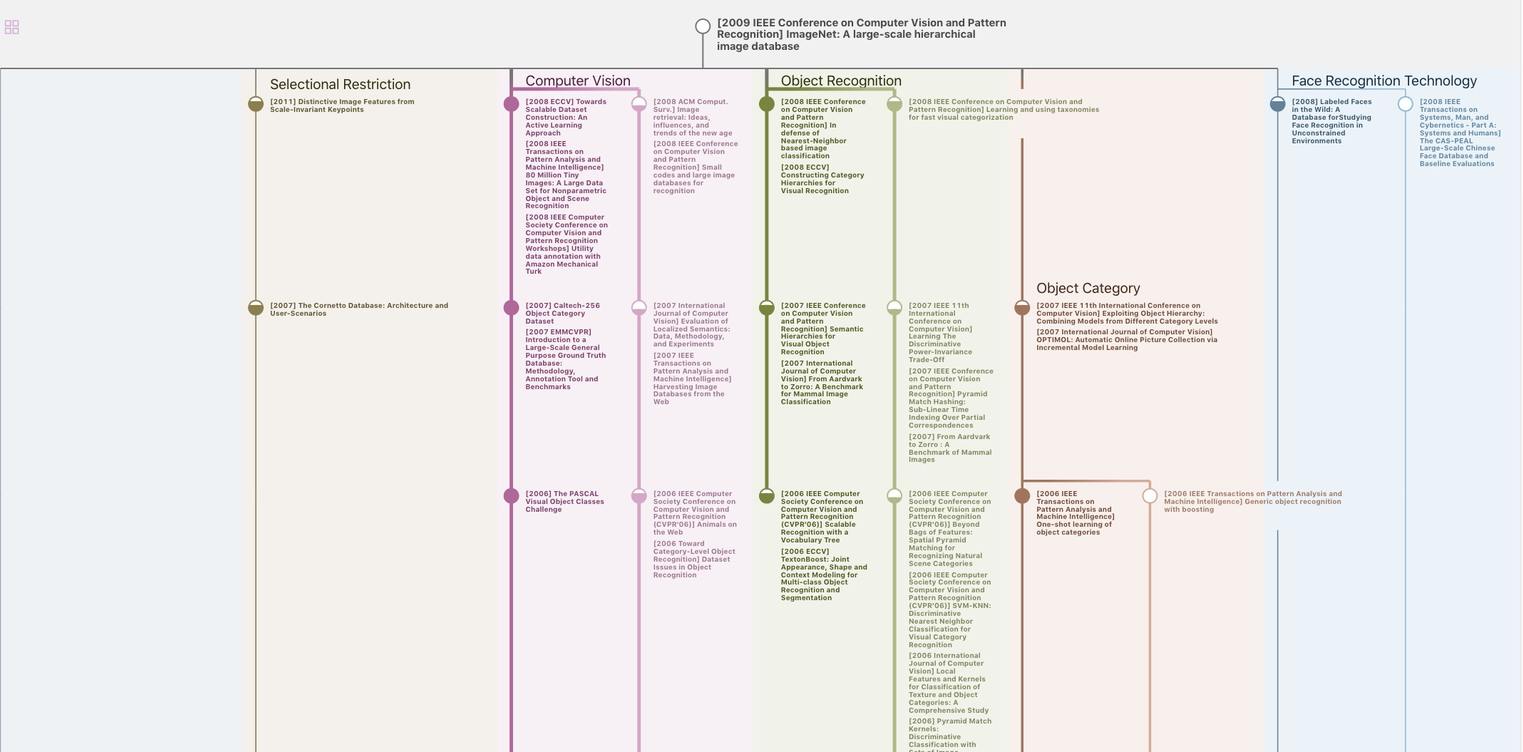
生成溯源树,研究论文发展脉络
Chat Paper
正在生成论文摘要