Meta-learning based instance manipulation for implicit discourse relation recognition
KNOWLEDGE-BASED SYSTEMS(2023)
摘要
Without discourse connectives, implicit discourse relations recognition (IDRR) remains a challenging task and has attracted increasing attention. However, most studies ignore the issues of class imbalance and data noise. To alleviate these two problems, in this paper, we propose to improve the robustness of IDRR models with two novel meta-learning based instance manipulation networks: Meta-Weight-Net (MW-Net) and Meta-Label-Net (ML-Net), which adaptively control the effect of training instances and smooth the label distributions during model training, respectively. Specifically, we use MW-Net to adaptively learn an instance weight function directly from data and integrates the instance weights into the objective function of IDRR. Meanwhile, we adopt ML-Net to dynamically refine the label distributions, leading to the smaller variance in updating gradients. We conduct experiments on the PDTB 2.0 corpus. Experimental results and in-depth analysis empirically demonstrate the effectiveness of our networks.(c) 2023 Elsevier B.V. All rights reserved.
更多查看译文
关键词
Implicit discourse relation recognition,Meta learning,Class imbalance,Instance weight
AI 理解论文
溯源树
样例
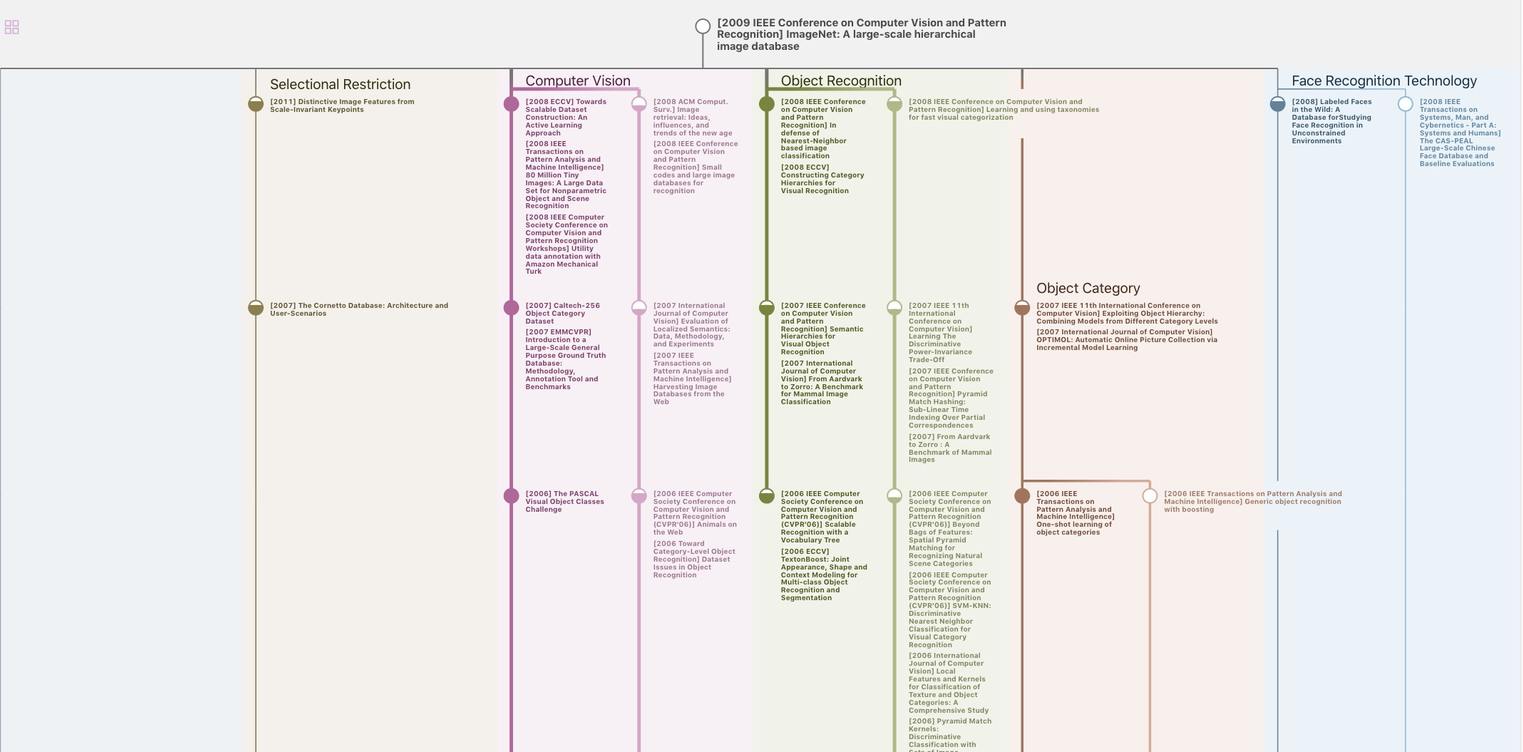
生成溯源树,研究论文发展脉络
Chat Paper
正在生成论文摘要