Transfer Learning to Detect Neonatal Seizure from Electroencephalography Signals
Neural computing & applications(2021)
Abstract
This paper offers a solution to the problem of detecting neonatal seizures via a transfer learning technique that judiciously reconstructs pre-trained deep convolution neural networks (p-DCNN), including alexnet, resnet18, googlenet, densenet, and resnet50. Multichannel electroencephalography (EEG) signals are converted to colour images for feeding them as an input for the p-DCNN. A deep neural network (DNN) such as a convolution neural network (CNN) may be directly used instead of transfer learning-based networks. However, a DNN requires too much training data, too much training time, and a computer with high-performance computational capability. The DNN also has several user-supplied hyper-parameters that must be tuned to obtain desirable classification success. To prevent these drawbacks, we propose a transfer learning technique to solve the neonatal seizures detection problem. Results of simulations and the statistical analysis enable us to devise a transfer learning technique employed for seizure detection.
MoreTranslated text
Key words
Transfer learning,Deep neural networks,Deep learning,Neonatal seizures,Electroencephalography
AI Read Science
Must-Reading Tree
Example
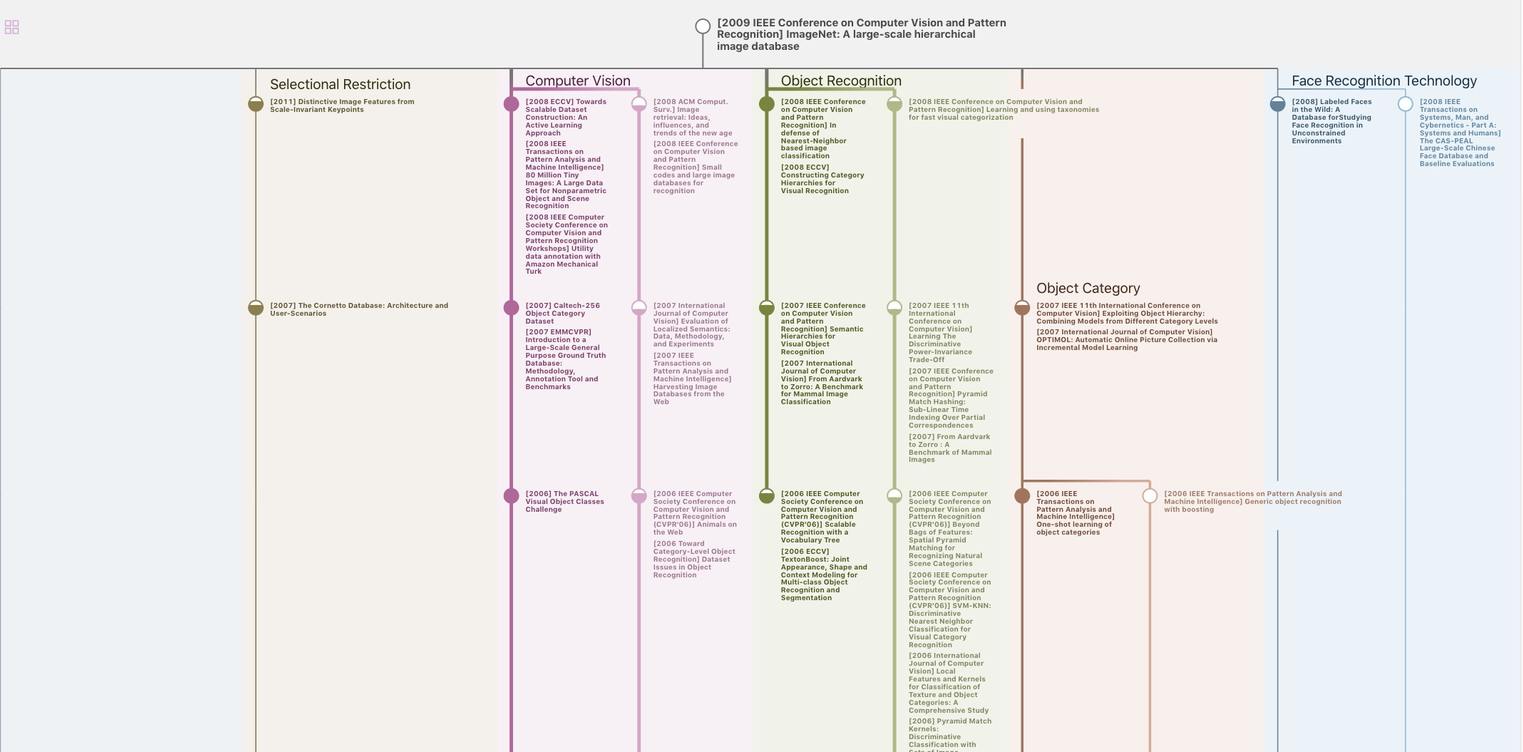
Generate MRT to find the research sequence of this paper
Chat Paper
Summary is being generated by the instructions you defined