Brute-force analysis of insight of phase-resolved partial discharge using a CNN
ELECTRICAL ENGINEERING(2023)
摘要
This study analyzes phase-resolved partial discharge (PD) signals using a convolutional neural network (CNN) for different electrode systems and attempts to reveal their relationship with the brute-force method. PD measurement is a diagnostic technique used for electrical insulating systems, and machine learning is needed to improve processing efficiency. However, the relationship between human insight and the learning model is unclear. The generated PD signals are first accumulated by each phase angle through applying a high AC voltage to four types of electrodes with a polyethylene sheet. Data are then converted to scatter images and classified using a CNN, with an accuracy rate of 98%. After cutting the data, including PD typical distribution, the classification demonstrates a slightly lower score of 95%. Finally, we divide the cut data into nine parts and choose whether to mask each part, then observe how the accuracy is affected. Results demonstrate that the CNN algorithm emphasizes specific parts of images. For example, when significant parts are contained, accuracy increases, and vice versa. In addition, the effect of noise on the insight and accuracy is partially revealed. It is clear from the results that appropriate parts need to be selected from big data.
更多查看译文
关键词
Partial discharge,Phase-resolved partial discharge,Machine learning,Convolutional neural network
AI 理解论文
溯源树
样例
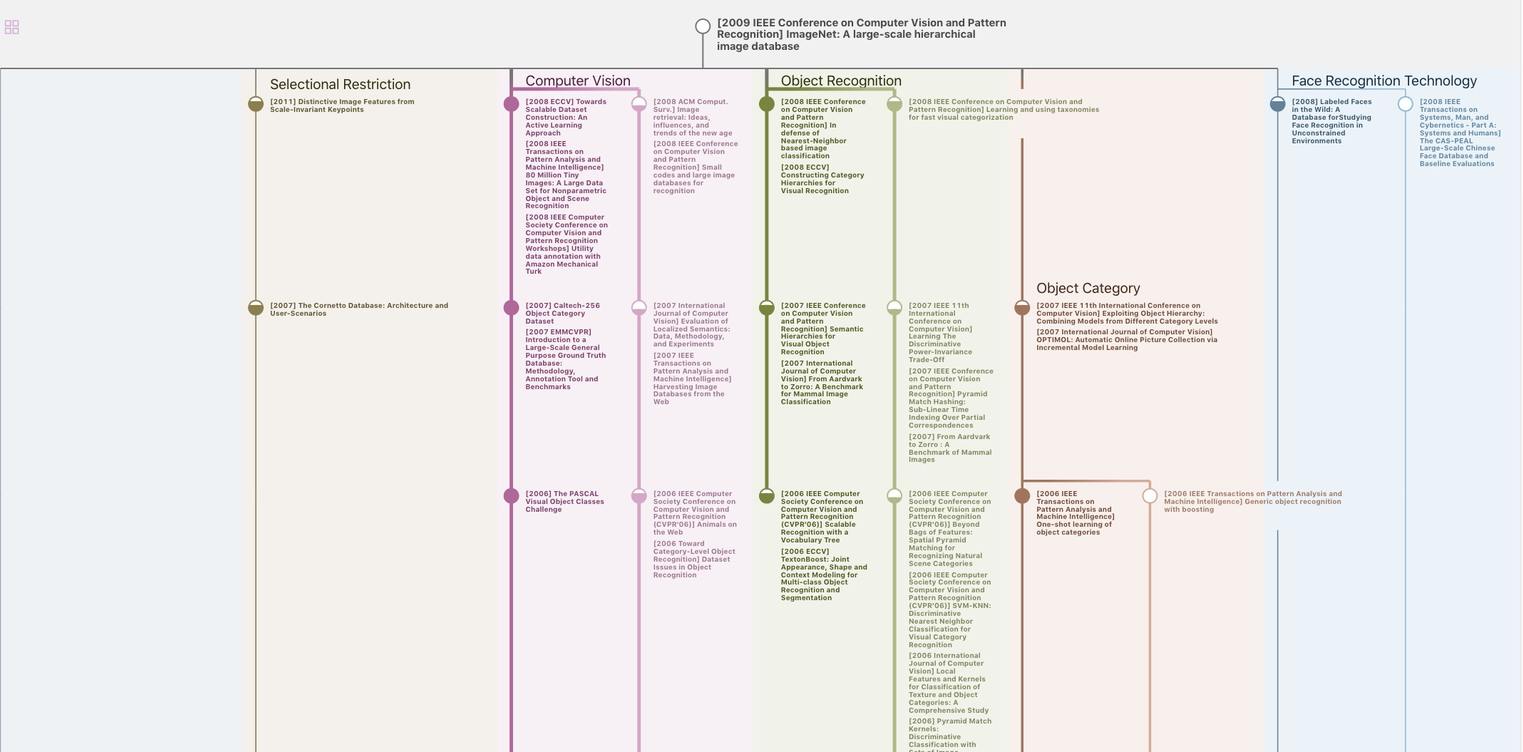
生成溯源树,研究论文发展脉络
Chat Paper
正在生成论文摘要