Error Graph Regularized Nonnegative Matrix Factorization for Data Representation
NEURAL PROCESSING LETTERS(2023)
摘要
Nonnegative matrix factorization (NMF) has been received much attention and widely applied to data mining by various researchers. It is believed that the non-negativity constraint makes NMF to learn a parts-based representation. Nevertheless, NMF fails to exploit the intrinsic manifold structure of the data. Therefore, many graph-based NMF methods have been proposed by incorporating a similarity graph. However, graph regularized NMF and its extensions do not consider the geometric structure of the given data is well preserved. In this paper, we propose an error graph regularized nonnegative matrix factorization (EGNMF) to perform the manifold learning. Our contribution is twofold: first, we introduce an error graph regularization term to maintain the geometric structures of the original data for each iterative update; second, we adopt a weight coefficient matrix to strengthen the important and weaken the non-important structures of the low-dimensional data. Experimental results on different benchmark datasets show that EGNMF is superior to competing methods.
更多查看译文
关键词
Error graph regularization,Nonnegative matrix factorization,Manifold learning,Low-dimensional representation learning
AI 理解论文
溯源树
样例
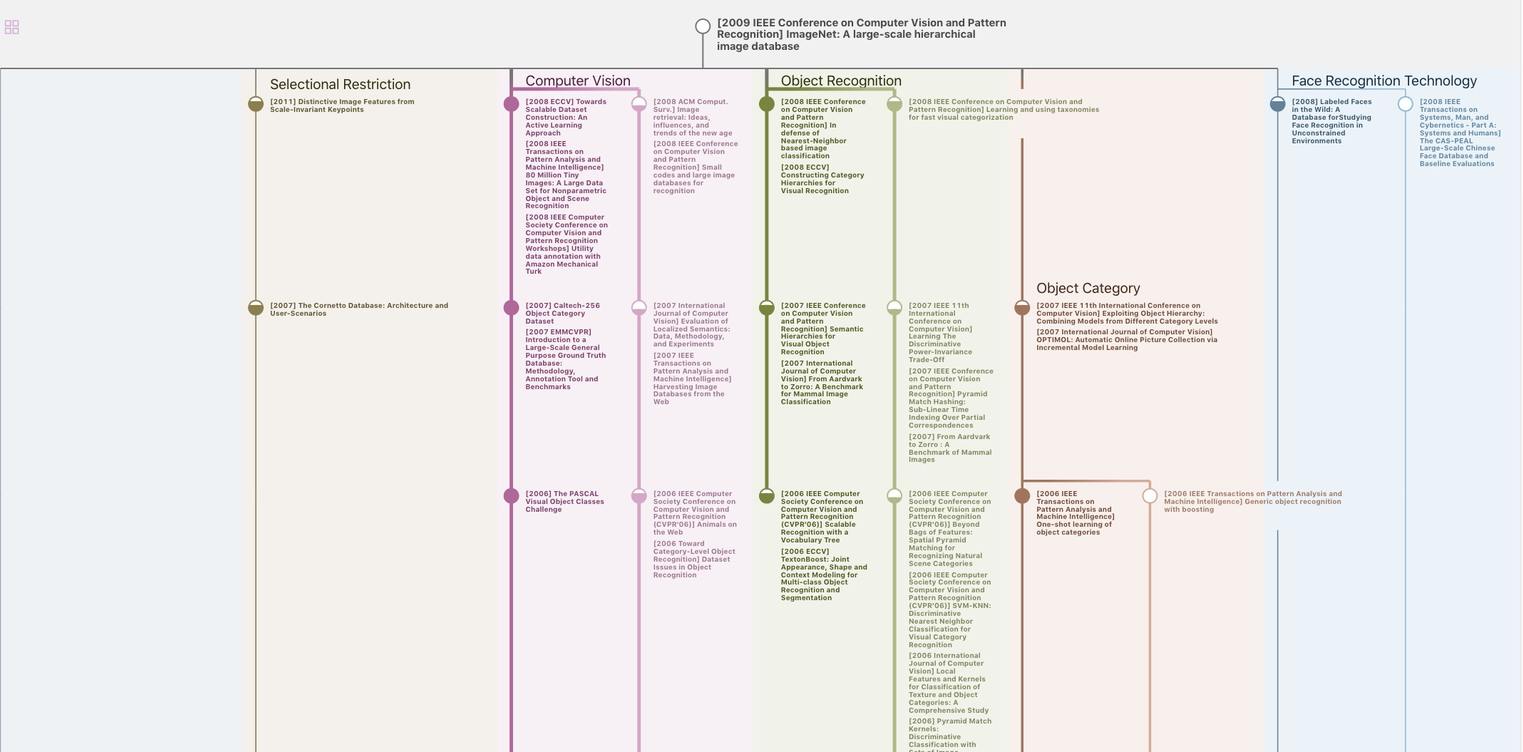
生成溯源树,研究论文发展脉络
Chat Paper
正在生成论文摘要