Examining the sensitivity of simulated EnMAP data for estimating chlorophyll-a and total suspended solids in inland waters
ECOLOGICAL INFORMATICS(2023)
摘要
Our study investigates the capability of the environmental mapping and analysis program (EnMAP) scenes simulated using the EnMAP end-to-end simulator software (EeteS) based on the AISA Eagle airborne data to predict chlorophyll-a (Chl-a) and total suspended solids (TSS) as two of the most crucial water quality indicators. Three machine learning (ML) approaches (principal component regression(PCR), partial least square regression (PLSR) and random forest (RF)) were employed to establish links between the simulated image spectra and the above-mentioned water attributes of the samples collected from several inland water reservoirs within the southern part of the Czech Republic. Airborne hyperspectral images were also used to develop a model to compare its performance with models developed based on the simulated EnMAP data. Adequate prediction accuracy was obtained for both Chl-a (R2 = 0.89, RMSE = 43.06 mu g/L, and Lin's concordance correlation co-efficient (LCCC) = 0.91) and TSS (R2 = 0.91, RMSE = 17.53 mg/L, and LCCC = 0.94), which were close enough to those obtained from the airborne hyperspectral images. Chl-a and TSS correlated with the wavelengths around 550 nm and 700 to 750 nm of the red and near-infrared (NIR) regions. In addition, the spatial distribution maps derived from the simulated EnMAP were comparable to those obtained from the AISA Eagle airborne data. Overall, it can be concluded that the simulated EnMAP image successfully and reliably predicted and spatially mapped the selected biophysical properties of the small inland water bodies.
更多查看译文
关键词
Biophysical properties,Hyperspectral imagery,Machine learning,Remote sensing,Satellite imagery,Water quality
AI 理解论文
溯源树
样例
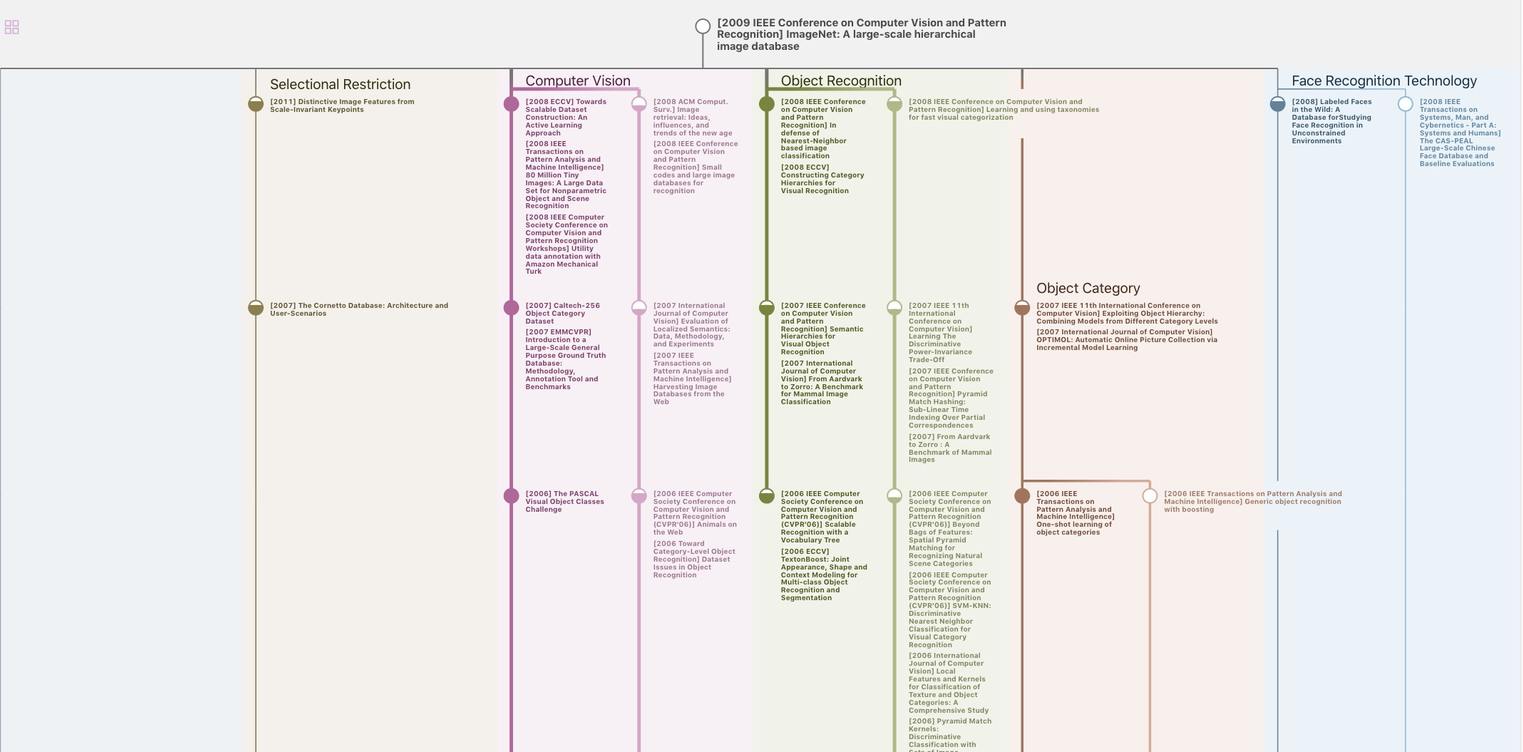
生成溯源树,研究论文发展脉络
Chat Paper
正在生成论文摘要