Fault detection in seismic data using graph convolutional network
JOURNAL OF SUPERCOMPUTING(2023)
摘要
Generally, seismic data have discontinuity or planar fracture anomalies in the volume of rocks, commonly known as seismic faults. A large number of methods exist to interpret seismic faults, nevertheless automating the process prevails to be a practical challenge. We propose a graph representation-based approach for interpreting faults in seismic data using the graph convolutional network (GCN). We extract 2D patches of data centered around seismic data points from the 3D seismic volumes for training. Then we represent these patches in the graph domain using the k-nearest neighbor graphs followed by the application of GCN. After training the patches in the networks, we classify the patches to identify the faults. We consider both synthetic and real data for training and testing. The seismic amplitude values, the seismic attribute values, and the successive difference values are used in the networks. We compare our implementation with the state-of-the-art method, the convolutional neural networks (CNN). The results show good accuracy when applied to both synthetic and real data. Additionally, the GCN method is more time efficient than that of CNN. We process the 3D seismic section by parallel processing the 2D patches.
更多查看译文
关键词
Graph convolutional network (GCN),Graph neural network (GNN),Seismic fault interpretation
AI 理解论文
溯源树
样例
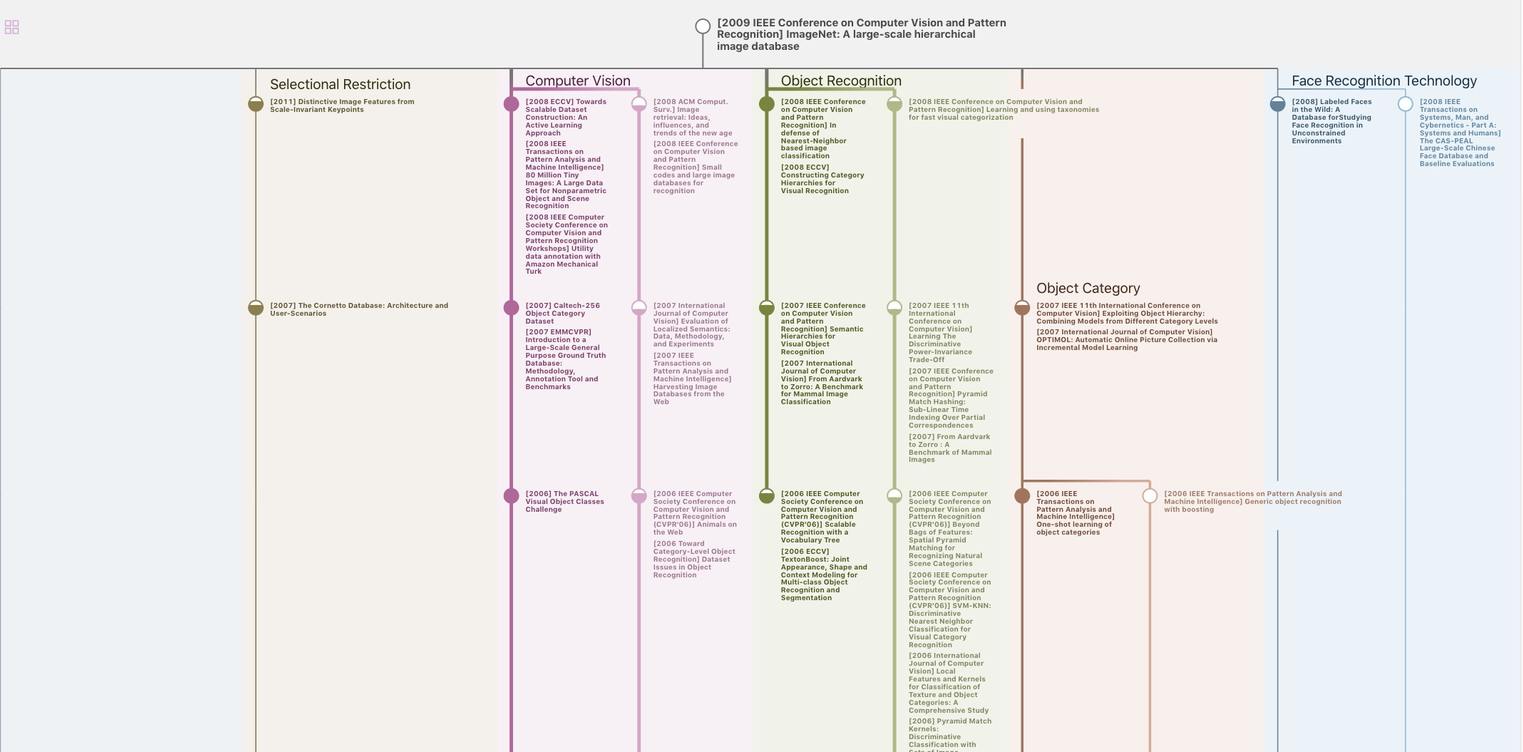
生成溯源树,研究论文发展脉络
Chat Paper
正在生成论文摘要