Predicting the HOMO-LUMO gap of benzenoid polycyclic hydrocarbons via interpretable machine learning
CHEMICAL PHYSICS LETTERS(2023)
摘要
While the experimental investigations and quantum chemistry methods have made significant progress on the exploration of electronic properties of benzenoid polycyclic hydrocarbons (BPHs), they are often time and labour consuming. Machine learning (ML) has recently been one of the most exciting tools that have entered the ma-terial science toolbox, providing an efficient way for property prediction and materials design at a much lower cost. In this work, we have applied the ML method to predict the HOMO-LUMO gaps of BPHs. Three datasets of BPHs are used, one was established by Gershoni-Poranne et al., and the other two were constructed by ourselves. The second dataset contains BPHs consisting of n benzene rings (n denotes the number of benzene rings, n < 10) but excluding the open-shell BPHs, whereas the third dataset encompasses the open-shell BPHs. We used different regression algorithms to build the ML models to obtain their structure-property relationship. After training, reasonable agreements between the model predictions and the reference values are obtained for the first and second datasets. Finally, we combined ML with the Shapley additive explanations method to explore important chemical features that affect the HOMO-LUMO gaps of BPHs. These results may provide insights to the rational design of BPHs as functional materials.
更多查看译文
关键词
Interpretable ML,Chemical informatics,Structure-property relationships,Polycyclic aromatic hydrocarbons,Benzenoid polycyclic hydrocarbons
AI 理解论文
溯源树
样例
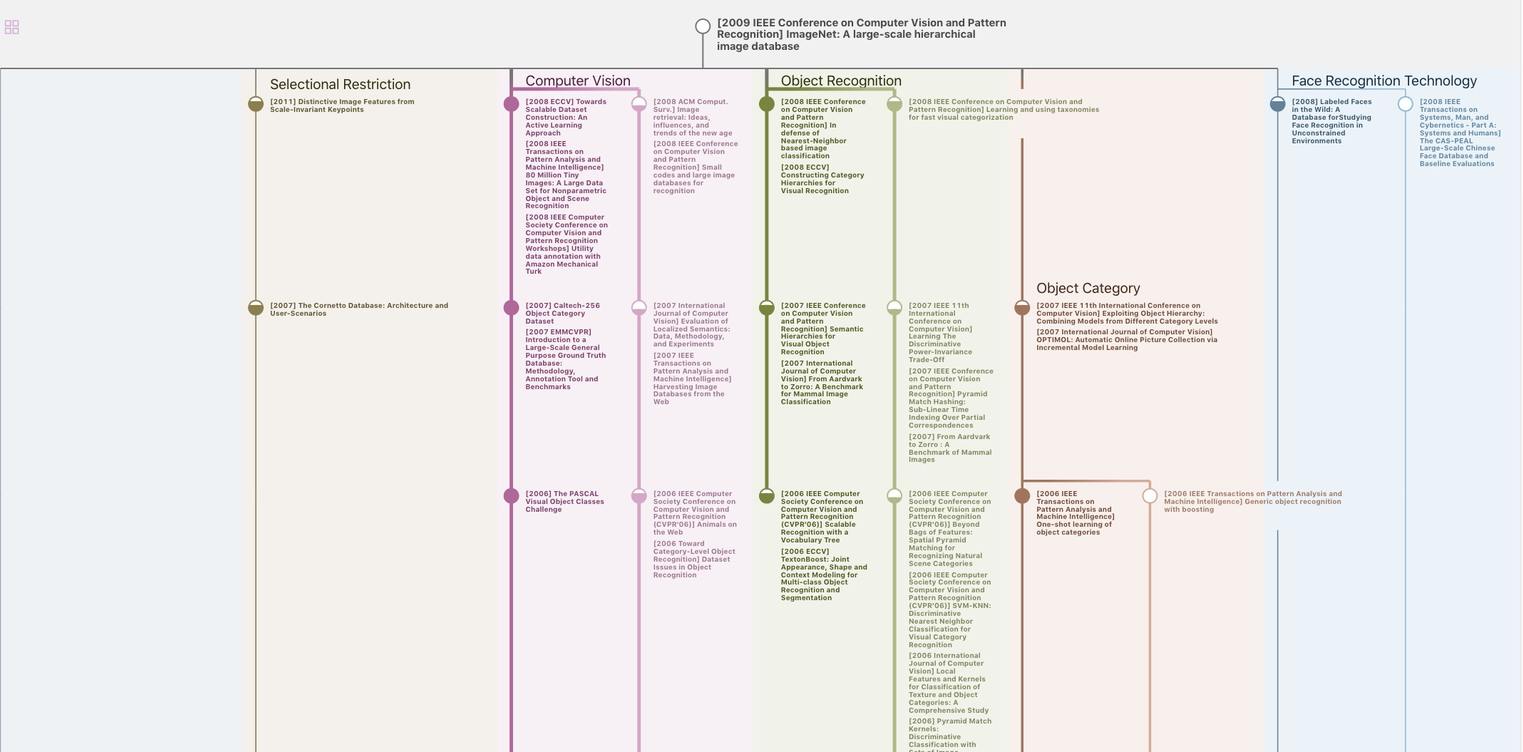
生成溯源树,研究论文发展脉络
Chat Paper
正在生成论文摘要