RF-BiLSTM Neural Network Incorporating Attention Mechanism for Online Ride-Hailing Demand Forecasting
SYMMETRY-BASEL(2023)
摘要
Accurately predicting online ride-hailing demand can help operators allocate vehicle resources on demand, avoid idle time, and improve traffic conditions. However, due to the randomness and complexity of online ride-hailing demand data, which are affected by many factors and mostly time-series in nature, it is difficult to forecast accurately and effectively based on traditional forecasting models. Therefore, this study proposes an online ride-hailing demand forecasting model based on the attention mechanism of a random forest (RF) combined with a symmetric bidirectional long short-term memory (BiLSTM) neural network (Att-RF-BiLSTM). The model optimizes the inputs and can use past and future data to forecast, improving the forecasting precision of online ride-hailing demand. The model utilizes a random forest to filter and optimize the input variables to reduce the neural network complexity, and then an attention mechanism was incorporated into the BiLSTM neural network to construct a demand forecasting model and validate it using actual Uber pickup data from New York City. Compared with other forecasting models (Att-XGBoost-BiLSTM, Att-BiLSTM, and pure LSTM), the results show that the proposed symmetrical Att-RF-BiLSTM online ride-hailing demand forecasting model has a higher forecasting precision and fitting degree, which indicates that the proposed model can be satisfactorily applied to the area of online ride-hailing demand.
更多查看译文
关键词
online ride-hailing,random forest,attention mechanism,BiLSTM,demand forecasting model
AI 理解论文
溯源树
样例
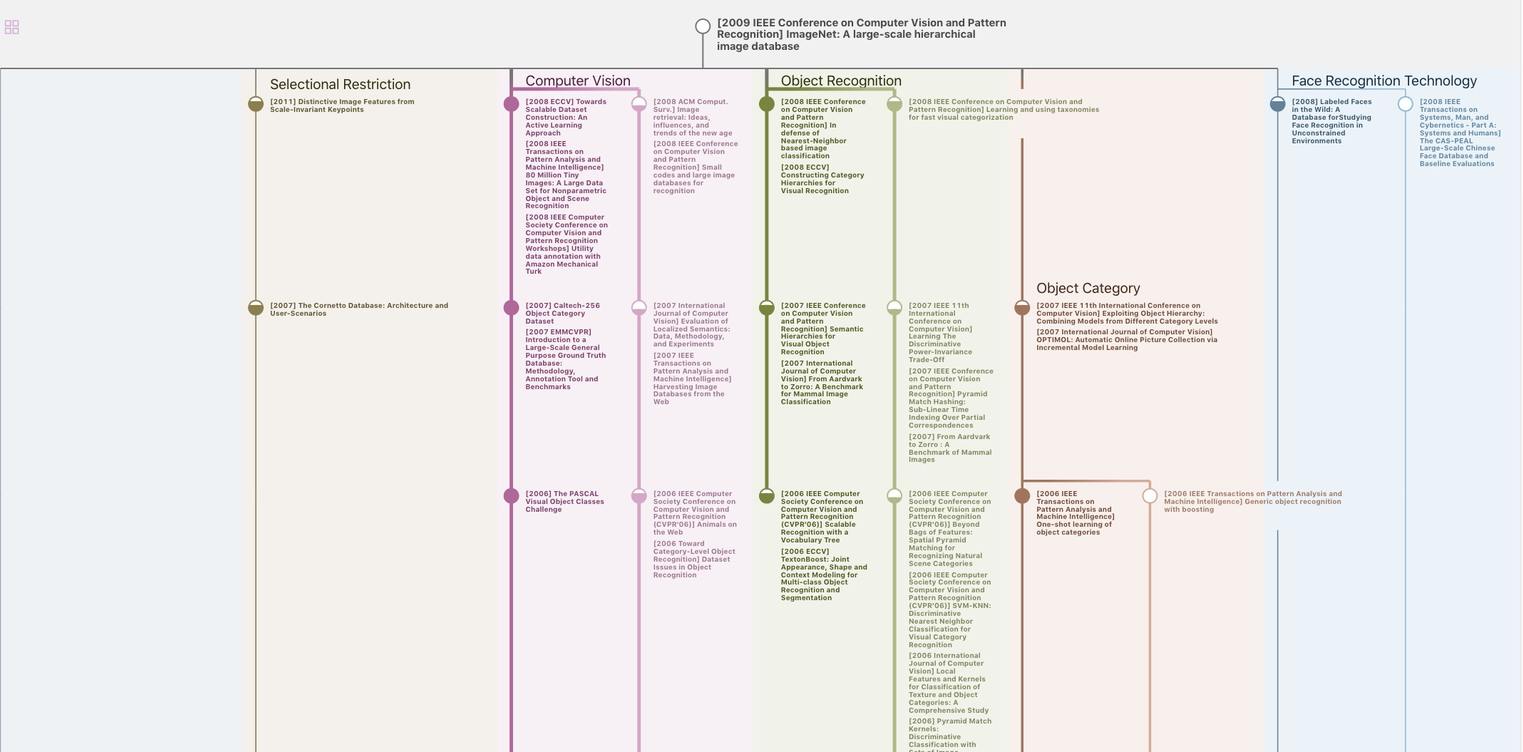
生成溯源树,研究论文发展脉络
Chat Paper
正在生成论文摘要