Enhancement-fusion feature pyramid network for object detection
JOURNAL OF ELECTRONIC IMAGING(2023)
摘要
Scale variation is one of the challenges of object detection. Most state-of-the-art object detectors depend on feature pyramid networks (FPN) for multiscale learning to deal with this problem, in which feature fusion is an essential operation. However, feature fusion does not sufficiently address the difficulty of the detection task. This paper presents an enhancement-fusion feature pyramid network (EFPN) to obtain reliable object representations for object detectors. Specifically, it contains a feature enhancement module (FEM) and a bottom-up path module (BPM). The FEM is used to eliminate the negative impact of the uneven distribution of object scales on the model performance. Then, a BPM is proposed to address the fusion inconsistency in the FPN. Additionally, an attention module (A(c)) is added to eliminate the information loss in the bottom-up aggregation process. EFPN is evaluated by combining it with state-of-the-art detection methods. Extensive experimental results on two datasets MS-COCO and VOC2007 demonstrate the effectiveness of the proposed method.
更多查看译文
关键词
object detection,enhancement-fusion
AI 理解论文
溯源树
样例
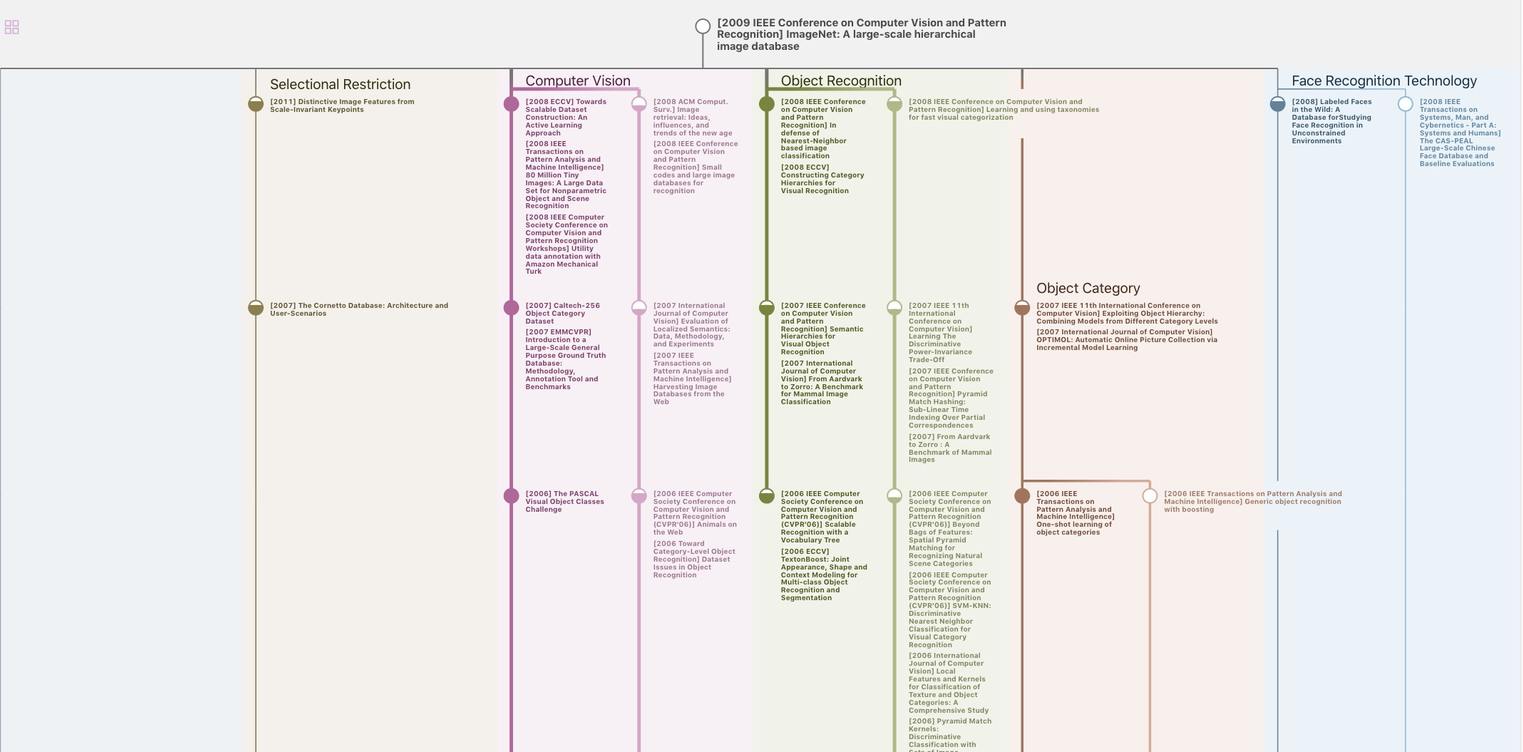
生成溯源树,研究论文发展脉络
Chat Paper
正在生成论文摘要