Fault detection of wind turbines using SCADA data and genetic algorithm-based ensemble learning
ENGINEERING FAILURE ANALYSIS(2023)
摘要
Due to global efforts to reduce the rise in the average global temperature by replacing fossil fuels, the amount of wind power installed worldwide is continuously increasing. The costs associated with running and maintaining wind farms make up a significant portion of the Levelized cost of wind energy. The main piece of equipment in a wind farm is a wind turbine (WT), and how well it functions safely and profitably depends heavily on its reliability. Early detection of WT faults can significantly lower the cost of wind farm maintenance. Fault detection is, therefore, essential for WTs to carry out. Supervisory control and data acquisition (SCADA) based approaches have significant advantages for developing condition monitoring systems for WTs. In this article, we have used genetic algorithm-based ensemble learning for anomaly detection of wind turbines using SCADA data. The proposed ensemble method consists of XGBoost, a random forest, and an extra tree model. XGBoost is used as a meta-model. A genetic algorithm is used for the selection of optimal features. A threshold is applied, and anomalies in the records are discovered where the RMSE is higher than the threshold. We have used several evaluation metrics to evaluate the performance of the proposed model. The proposed model's mean absolute percentage error (MAPE) is 0.83%. We have also compared our proposed model with state-of-the-art machine learning algorithms.
更多查看译文
关键词
Power generation,Wind turbines,SCADA data,Ensemble learning,Operation errors,Fault detection
AI 理解论文
溯源树
样例
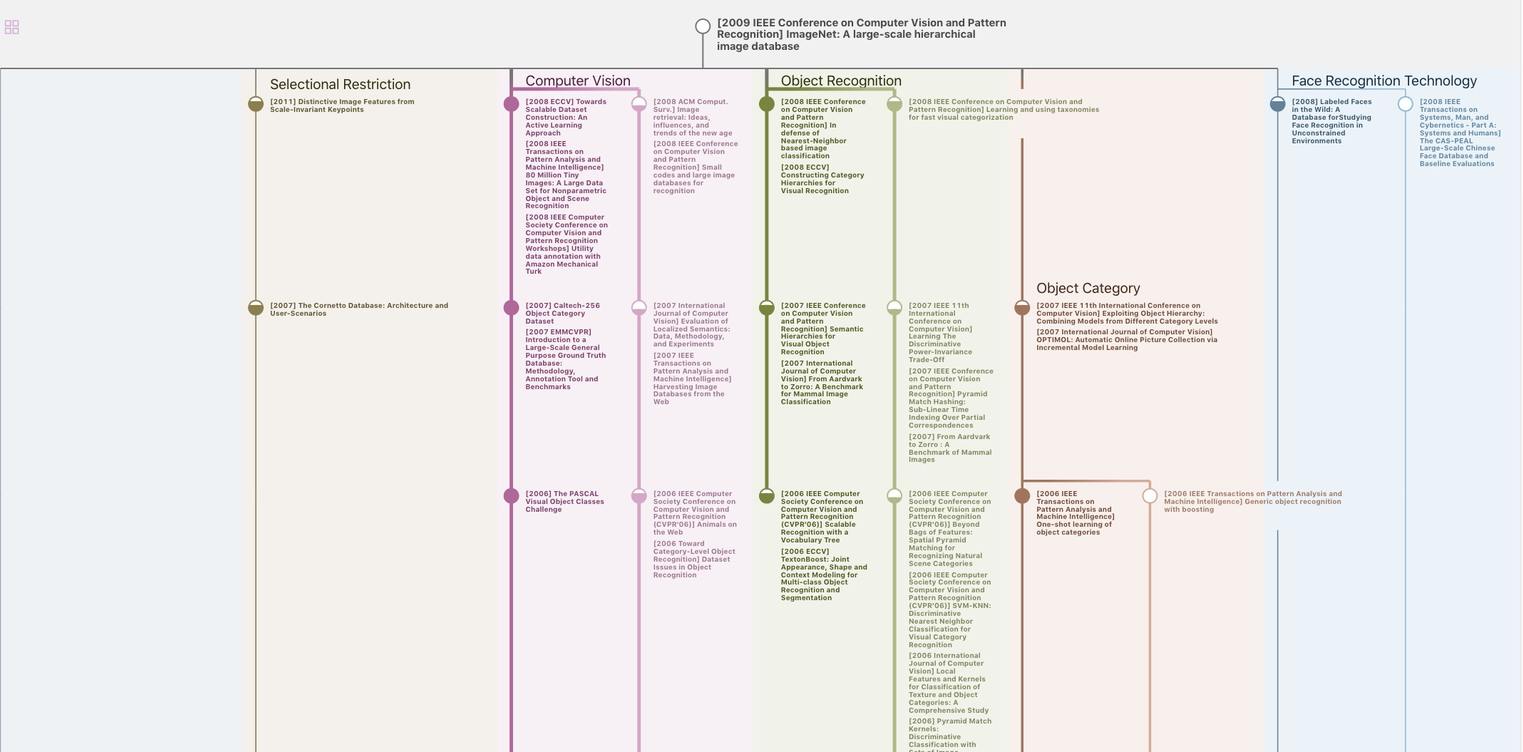
生成溯源树,研究论文发展脉络
Chat Paper
正在生成论文摘要