Automated machine learning-based framework of heating and cooling load prediction for quick residential building design
ENERGY(2023)
摘要
Reducing the heating and cooling load through energy-efficient building design can help decarbonize the building sector. Heating and cooling load prediction using machine learning (ML) techniques become increas-ingly important in the rapid assessment of building design variables at the early design stage. However, when applying the ML techniques, it still requires expert knowledge and manually frequent intervention to improve the prediction performance. Hence, this study proposed an automated machine learning (AutoML)-based framework to automatically generate the optimal ML pipelines for heating and cooling load prediction. An experimental dataset of residential buildings was used to evaluate the proposed framework. The proposed framework achieved the best performance with R2 of 0.9965 and RMSE of 0.602 kWh/m2 for heating load prediction, and R2 of 0.9899 and RMSE of 0.973 kWh/m2 for cooling load prediction. The prediction results showed that the proposed framework outperformed the other improved ML models from the representative studies in the last five years. Further, an explainable analysis of the ML models was explored to reveal the relationships between design variables and heating and cooling load. The proposed framework aims at promoting the AutoML-based frame-work to designers for building energy performance prediction without excessive ML knowledge and manually frequent intervention.
更多查看译文
关键词
cooling load prediction,heating,building,learning-based
AI 理解论文
溯源树
样例
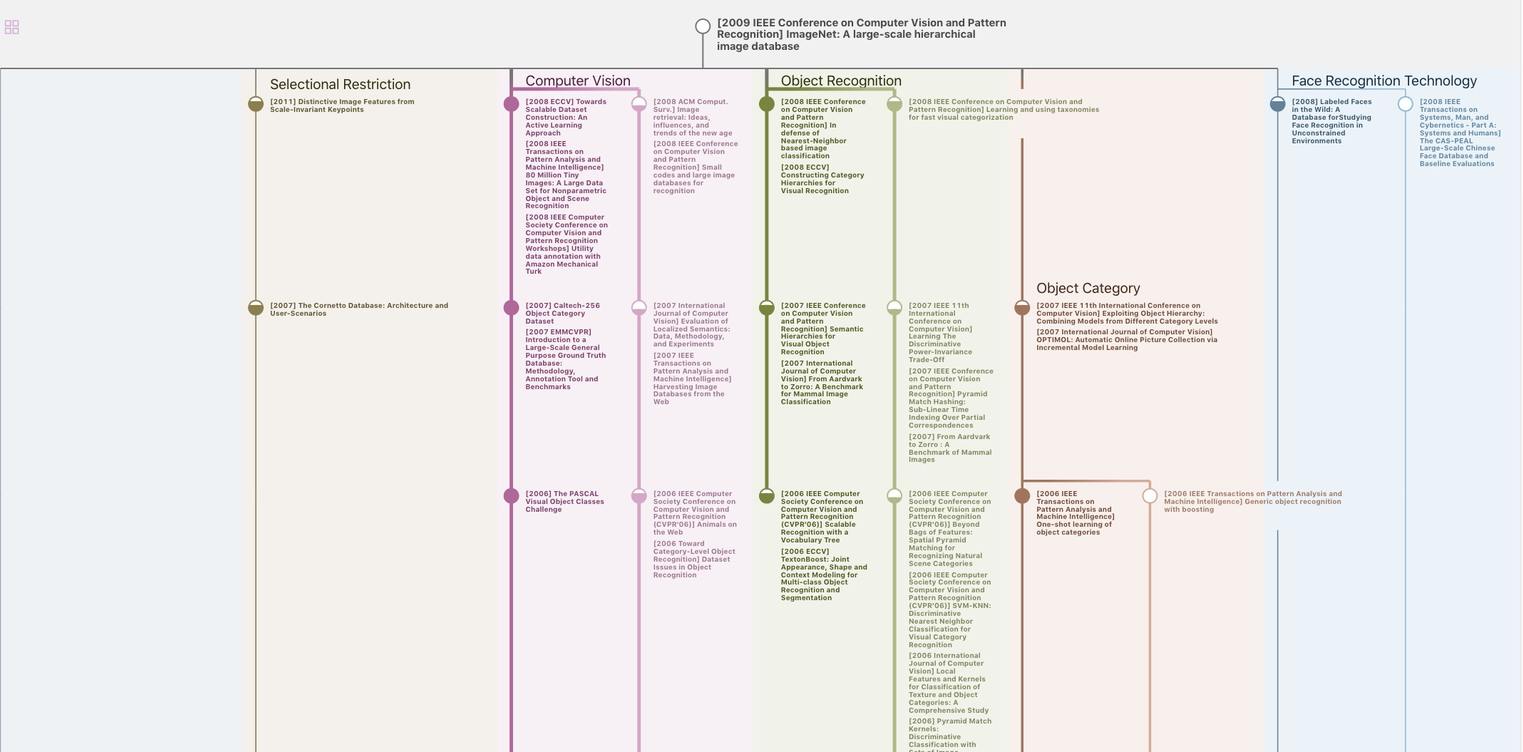
生成溯源树,研究论文发展脉络
Chat Paper
正在生成论文摘要