Strategically switching metaheuristics for effective parameter estimation of electrochemical lithium-ion battery models
JOURNAL OF ENERGY STORAGE(2023)
摘要
Electrochemical lithium-ion battery models have drawn considerable research attention as alternative exper-imental approaches to elucidate the internal mechanisms and physical properties of lithium-ion batteries. To obtain such useful models, accurate parameter estimation is an essential prerequisite, although it is challenging because of their complexity. Metaheuristics-based parameter estimation methods have been suggested individ-ually in this regard. To achieve more accurate parameter estimation, this study provides a systematic guide for strategically switching to an appropriate metaheuristic. First, we identify objective functions that are beneficial to each metaheuristic. Thus, ten baseline functions suitable for specific metaheuristics are selected, and learned by a convolutional neural network (CNN). From the sample particles collected up to present, the designed CNN provides the most similar baseline function and then recommends the corresponding proper metaheuristic. To escape from local optima and deliver a better solution, particle resampling and metaheuristic suitability tests are also performed if the current metaheuristic does not significantly improve within the prescribed iteration. Compared with average results over five runs of each metaheuristic, experimental data demonstrate that the proposed strategically switched metaheuristics (SSM) reduces the difference between experimental and simulated voltage responses by more than half. Additionally, the SSM estimates the external short circuit resistance with a 3 % error using the internal short circuit (ISC) built-in model.
更多查看译文
关键词
metaheuristics,effective parameter estimation,lithium-ion
AI 理解论文
溯源树
样例
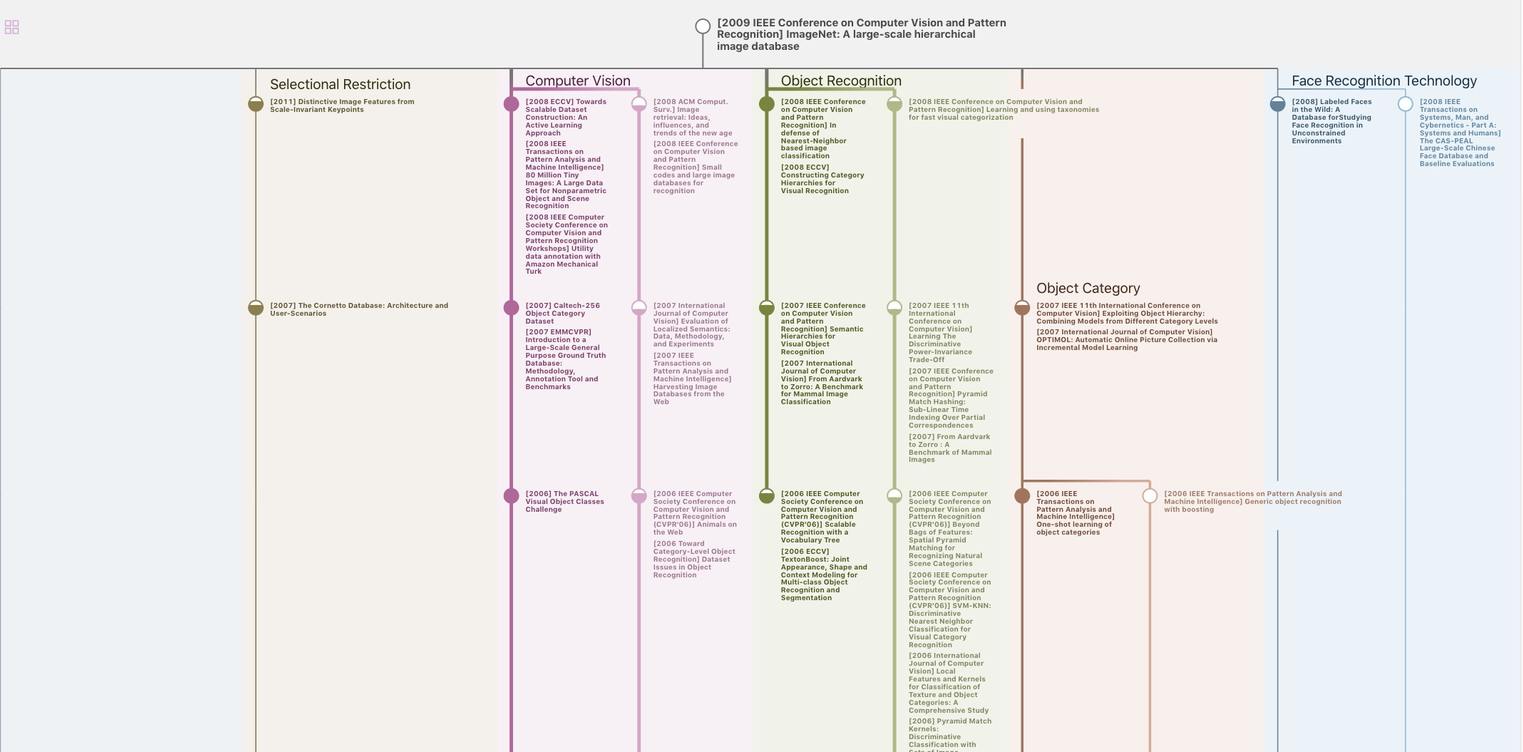
生成溯源树,研究论文发展脉络
Chat Paper
正在生成论文摘要