Dissipativity Analysis of Delayed Recurrent Neural Networks via a Flexible Negative-Definiteness Determination Method
IEEE SENSORS JOURNAL(2023)
摘要
The working condition of high-speed train wheelset bearings is complex and bad, which makes its vibration signal contain strong noise. To obtain more information of samples, signals are constructed into graphs and fit by graph convolution network (GCN). The existing GCN-based models still have some problems, such as insufficient theoretical basis of graph and the unstable and overfitting during training of models, which significantly limits the diagnosis accuracy. To address these issues, first, a weighted e-recurrence network is proposed to construct the recurrence graph (RG). The recurrence relationship between samples is analyzed by the recursive nature of vibration signals. Maximum mean discrepancy (MMD) is used to obtain the regenerated Hilbert spatial distribution distance between two samples to reduce the weight gap between different recursive pairs. Also, the proposed method has better ex ante interpretability. Second, a vertex mean normalization (MN) layer is proposed to normalize individual vertex features to introduce some spatial perturbations and reduce the variance of vertex features, which can improve training stability and reduce overfitting. In addition, multichannel MN-GCN is proposed to fit multiscale graph features. Analyze recurrence nature of samples at different scales through RGs with varying densities of connection to improve the antinoise ability. Experimental results show that the proposed model can obtain spatial topology information accurately and improve the wheelset-bearing system fault diagnosis performance.
更多查看译文
关键词
Vibrations, Fault diagnosis, Convolution, Transforms, Training, Time series analysis, Sensors, Graph convolution network (GCN), maximum mean discrepancy (MMD)-weighted epsilon-recurrence network (RN), multichannel mean normalization graph convolution network (MN-GCN), wheelset-bearing system fault diagnosis
AI 理解论文
溯源树
样例
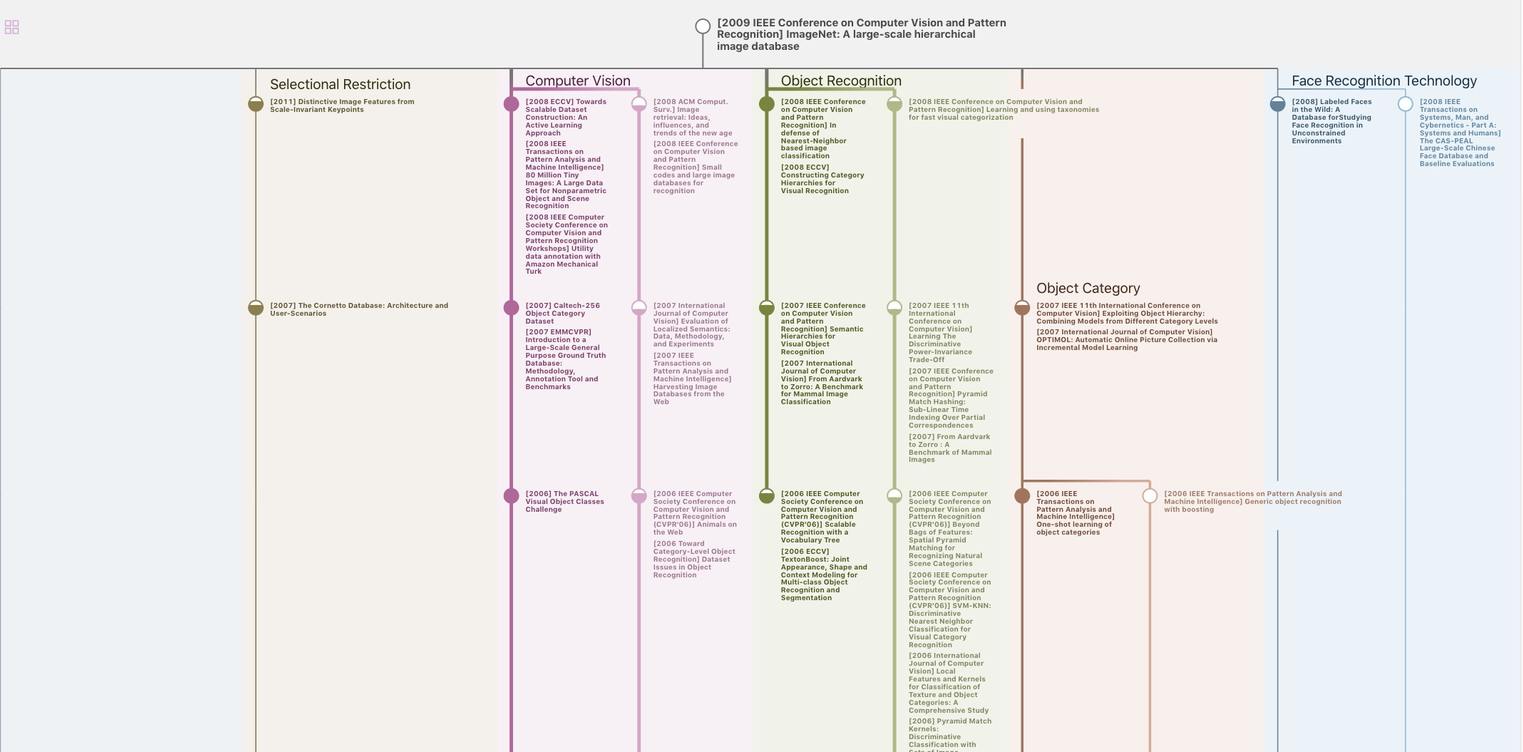
生成溯源树,研究论文发展脉络
Chat Paper
正在生成论文摘要