Machine Learning Model-Based Estimation of XCO2 with High Spatiotemporal Resolution in China
ATMOSPHERE(2023)
摘要
As the most abundant greenhouse gas in the atmosphere, CO2 has a significant impact on climate change. Therefore, the determination of the temporal and spatial distribution of CO2 is of great significance in climate research. However, existing CO2 monitoring methods have great limitations, and it is difficult to obtain large-scale monitoring data with high spatial resolution, thus limiting the effective monitoring of carbon sources and sinks. To obtain complete Chinese daily-scale CO2 information, we used OCO-2 XCO2 data, Carbon Tracker XCO2 data, and multivariate geographic data to build a model training data set, which was then combined with various machine learning models including Random Forest, Extreme Random Forest, XGBoost, LightGBM, and CatBoost. The results indicated that the Random Forest model presented the best performance, with a cross-validation R-2 of 0.878 and RMSE of 1.123 ppm. According to the final estimation results, in terms of spatial distribution, the highest multi-year average RF XCO2 value was in East China (406.94 +/- 0.65 ppm), while the lowest was in Northwest China (405.56 +/- 1.43 ppm). In terms of time, from 2016 to 2018, the annual XCO2 in China continued to increase, but the growth rate showed a downward trend. In terms of seasonal effects, the multi-year average XCO2 was highest in spring (407.76 +/- 1.72 ppm) and lowest in summer (403.15 +/- 3.36ppm). Compared with the Carbon-Tracker data, the XCO2 data set constructed in this study showed more detailed spatial changes, thus, can be effectively used to identify potentially important carbon sources and sinks.
更多查看译文
关键词
xco2,high spatiotemporal resolution,estimation,china,model-based
AI 理解论文
溯源树
样例
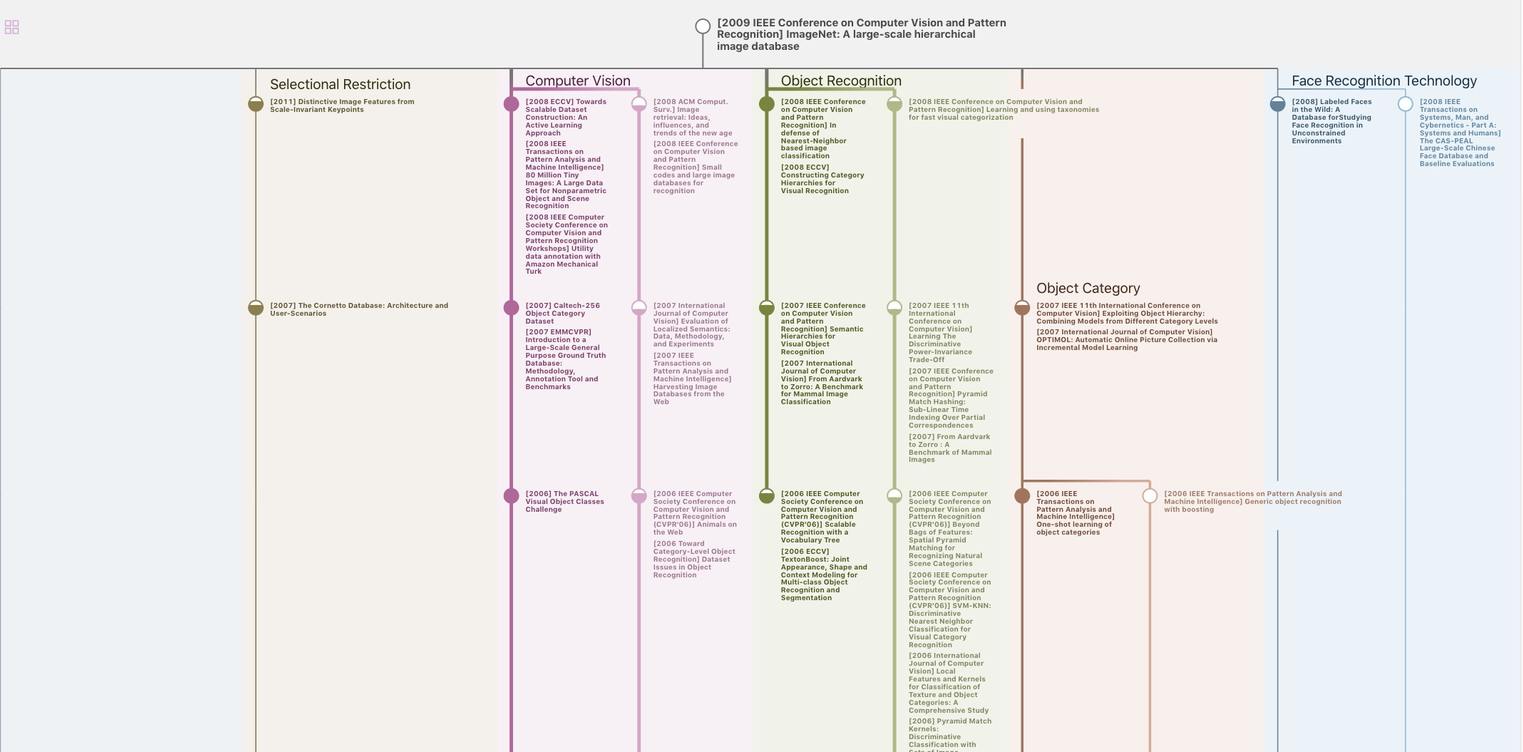
生成溯源树,研究论文发展脉络
Chat Paper
正在生成论文摘要