Transformer-based Local-Global Guidance for Image Captioning
EXPERT SYSTEMS WITH APPLICATIONS(2023)
摘要
Image captioning is a difficult problem for machine learning algorithms to compress huge amounts of images into descriptive languages. The recurrent models are popularly used as the decoder to extract the caption with significant performance, while these models have complicated and inherently sequential overtime issues. Recently, transformers provide modeling long dependencies and support parallel processing of sequences compared to recurrent models. However, recent transformer-based models assign attention weights to all candidate vectors based on the assumption that all vectors are relevant and ignore the intra-object relationships. Besides, the complex relationships between key and query vectors cannot be provided using a single attention mechanism. In this paper, a new transformer-based image captioning structure without recurrence and convolution is proposed to address these issues. To this end, a generator network and a selector network to generate textual descriptions collaboratively are designed. Our work contains three main steps: (1) Design a transformer-based generator network as word-level guidance to generate next words based on the current state. (2) Train a latent space to learn the mapping of captions and images into the same embedding space to learn the text-image relation. (3) Design a selector network as sentence-level guidance to evaluate next words by assigning fitness scores to the partial captions through the embedding space. Compared with the architecture of existing methods, the proposed approach contains an attention mechanism without the dependencies of time. It executes each state to select the next best word using local–global guidance. In addition, the proposed model maintains dependencies between the sequences, and can be trained in parallel. Several experiments on the COCO and Flickr datasets demonstrate that the proposed approach can outperform various state-of-the-art models over well-known evaluation measures.
更多查看译文
关键词
Attention,Transformer,Encoder-decoder,Image captioning,Deep learning
AI 理解论文
溯源树
样例
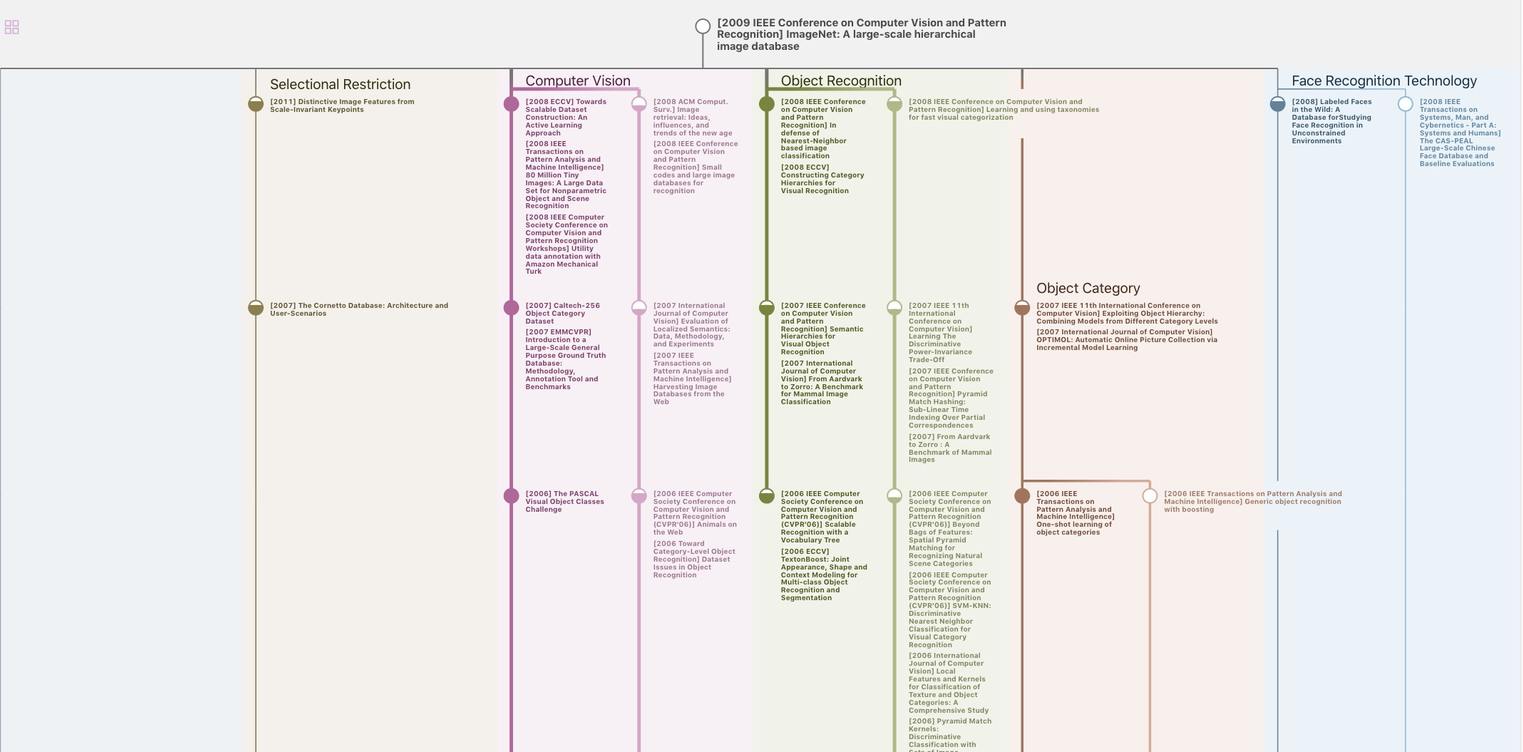
生成溯源树,研究论文发展脉络
Chat Paper
正在生成论文摘要