A CVAE-within-Gibbs sampler for Bayesian linear inverse problems with hyperparameters
COMPUTATIONAL & APPLIED MATHEMATICS(2023)
摘要
We propose a conditional variational auto-encoder within Gibbs sampling (CVAE-within-Gibbs) for Bayesian linear inverse problems where the prior or the likelihood function depends on ambiguous hyperparameters. The method builds on ideas from classical sampling theory and recent advances in deep generative models to approximate complicated probability distributions. Specifically, we use a CVAE model which is trained with a large amount of data to learn the conditional density of hyperparameters in the original Gibbs sampler. The learned property of the conditional posterior provides more flexibility than classical Gibbs sampling because it avoids manually or experimentally determining the hyperpriors and their hyperparameters. We demonstrate the performance of the proposed method for three linear inverse problems, i.e., image deblurring, signal denoising, and boundary heat flux identification in a heat conduction problem.
更多查看译文
关键词
Inverse problems,Bayesian inference,Gibbs sampling,Conditional variational auto-encoder,Hyperparameters
AI 理解论文
溯源树
样例
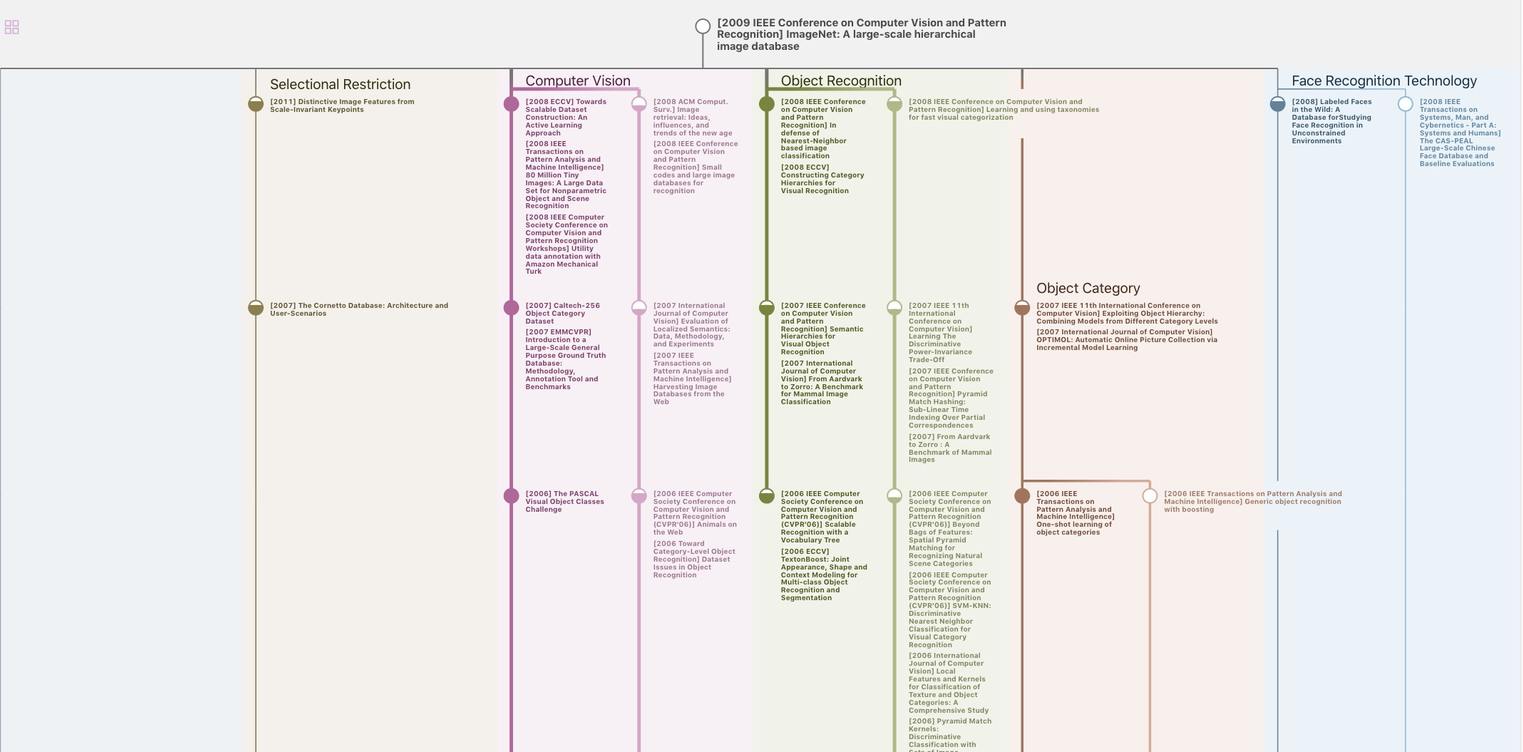
生成溯源树,研究论文发展脉络
Chat Paper
正在生成论文摘要