Towards Better Long-Tailed Oracle Character Recognition with Adversarial Data Augmentation.
Pattern recognition(2023)
摘要
Deciphering oracle bone script is of great significance to the study of ancient Chinese culture as well as archaeology. Although recent studies on oracle character recognition have made substantial progress, they still suffer from the long-tailed data situation that results in a noticeable performance drop on the tail classes. To mitigate this issue, we propose a generative adversarial framework to augment oracle characters in the problematic classes. In this framework, the generator produces synthetic data through convex combinations of all the available samples in the corresponding classes, and is further optimized through adversarial learning with the classifier and simultaneously the discriminator. Meanwhile, we in-troduce Repatch to generalize samples in the generator. Since tail classes do not have sufficient data for convex combinations, we propose the TailMix mechanism to generate suitable tail class samples from other classes. Experimental results show that our proposed algorithm obtains remarkable performance in oracle character recognition and achieves new state-of-the-art average (total) accuracy with 86.03% (89.46%), 86.54% (93.86%), 95.22% (96.17%) on the three datasets Oracle-AYNU, OBC306 and Oracle-20K, respectively.(c) 2023 Elsevier Ltd. All rights reserved.
更多查看译文
关键词
Oracle character recognition,Long tail,Data imbalance,Data augmentation,Mixup strategy,Generative adversarial networks
AI 理解论文
溯源树
样例
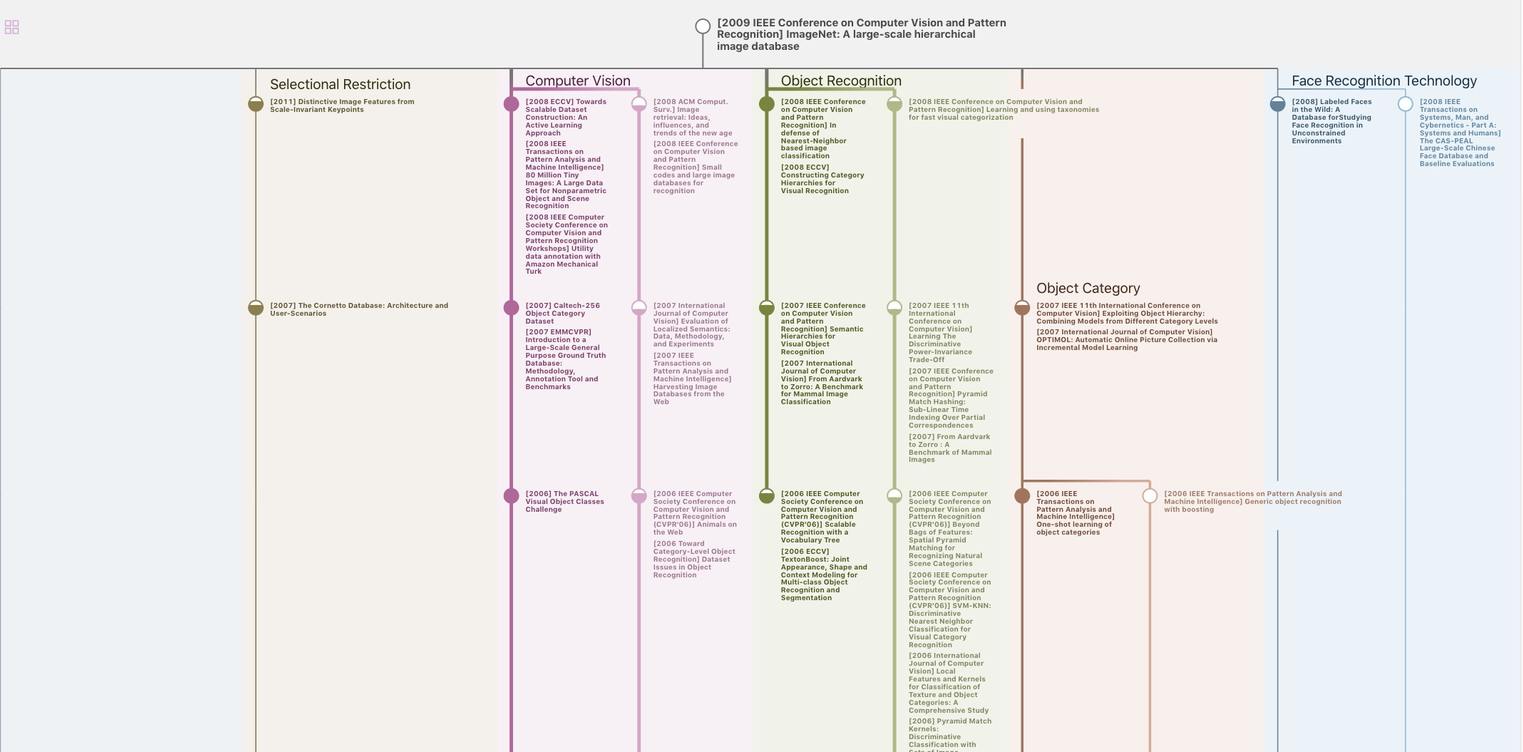
生成溯源树,研究论文发展脉络
Chat Paper
正在生成论文摘要