Physics-based Bayesian linear regression model for predicting length of mixed oil
GEOENERGY SCIENCE AND ENGINEERING(2023)
摘要
Precise estimation of the length of mixed oil is important to optimize the operation efficiency of products pipeline. The feasibility of physics models is limited by low accuracy or numerical simulation burden. The current frequentist predictive models require a sufficient number of historical samples, or they are easily encountered with over-fitting phenomenon. Bayesian linear regression model may alleviate this issue while predictive performance is also unsatisfactory without fusion of physical knowledge. To tackle such problems, this article proposes a new modeling method by employing the physics model to provide the nonlinear expression form and the underlying prior knowledge to the Bayesian linear regression model (BLR), and a physics-based Bayesian linear regression model (PBBLR) is developed. Based on the real product oil pipeline dataset, a per-formance comparison experiment with the Artificial Neural Network, the Gradient Boosting Decision Tree al-gorithm, two state-of-the-art predictive models, least squares estimation and pure Bayesian linear regression model is carried out, and the results verify the effectiveness of the proposed modeling method as the PBBLR shows optimal predictive performance in all scenarios. This research can help promote the predictive accuracy of mixed oil length of product oil pipelines and provide reference for the physics-data hybrid modeling method.
更多查看译文
关键词
Products pipeline,Mixed oil length,Physics -data,Hybrid modeling,Bayesian linear regression
AI 理解论文
溯源树
样例
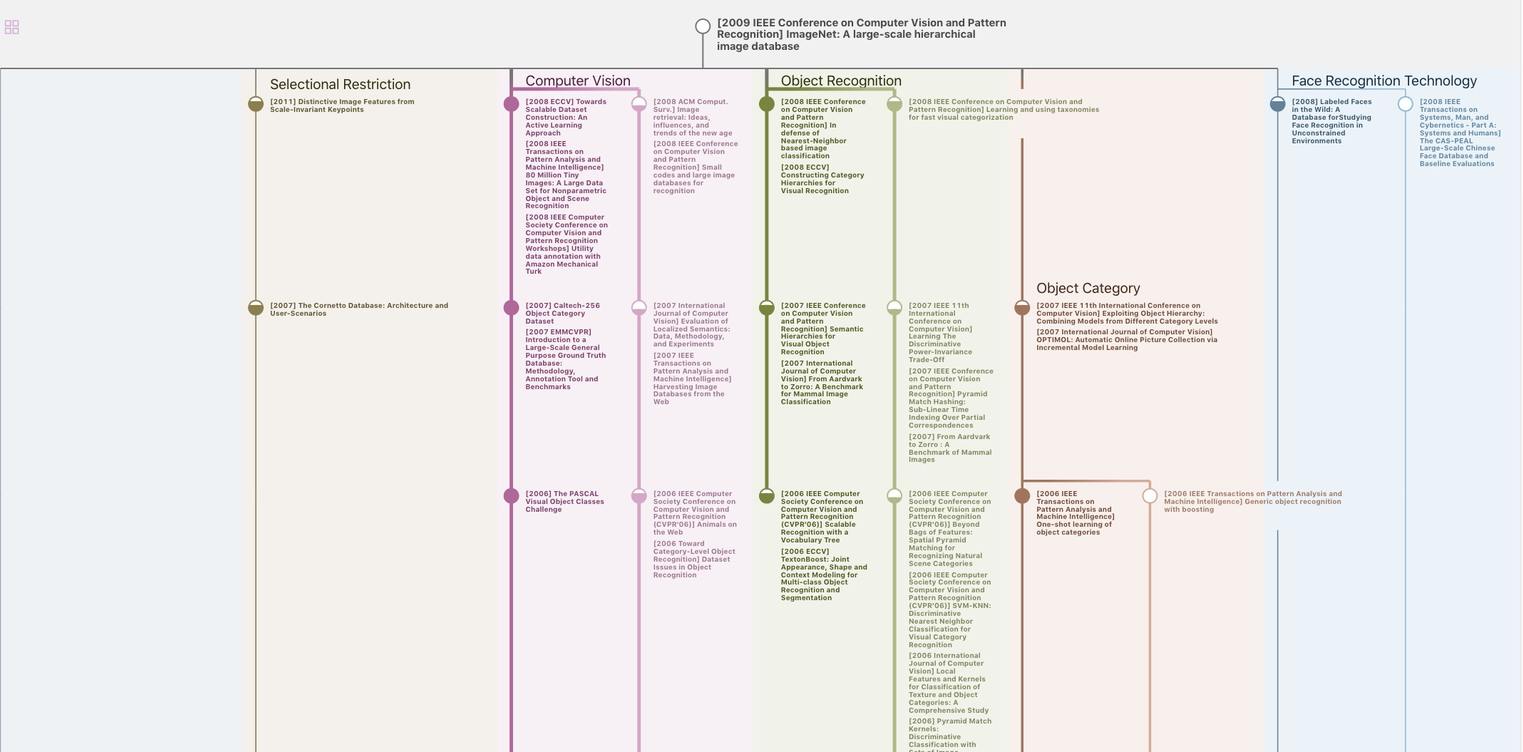
生成溯源树,研究论文发展脉络
Chat Paper
正在生成论文摘要