LogRegX: An Explainable Regression Network for Cross-Well Geophysical Logs Generation
IEEE TRANSACTIONS ON INSTRUMENTATION AND MEASUREMENT(2023)
摘要
Geophysical logging instruments continuously measure multiple geophysical properties of borehole rocks, thus providing a feasible way to fine borehole geology modeling. Since the missing problem of well logs is inevitable, it is essential to generate the missing logs by the available ones. Recently, a large body of interdisciplinary studies has demonstrated the effectiveness of applying machine learning to solve the missing logs generation problem, under which the training and testing datasets obey the independent and identical distribution (iid) assumption. This assumption, however, is not satisfied in the case of the cross-well missing logs generation task. A standard method to solve the non-iid issue is to map source and target data to a common feature space and then employ mean maximum discrepancy (MMD) to measure domain differences. However, this method suffers from high computational complexity and poor feature explainability when dealing with log generation tasks. To solve the above problems, we propose an explainable regression network for cross-well geophysical logs generation named LogRegX. LogRegX integrates single-well feature extraction, cross-well feature alignment, and missing logs prediction while maintaining the explainability of logging features. Specifically, LogRegX leverages the gating mechanism to fuse multiscale logging features to capture the response characteristics of well logs. The learned source and target feature representations are subject to domain discrepancy constraints, measured by random Fourier feature transform-induced MMD. Additionally, a target-domain information-retaining mechanism is introduced to maintain the structure of target data so that the transferred features are explainable. Experiments on real-world field data demonstrate the superiority and the explainability of LogRegX over the existing methods.
更多查看译文
关键词
Task analysis,Neural networks,Machine learning,Convolutional neural networks,Training,Feature extraction,Instruments,Discrepancy,domain adaptation,explainability,geophysical logs generation,unilateral alignment
AI 理解论文
溯源树
样例
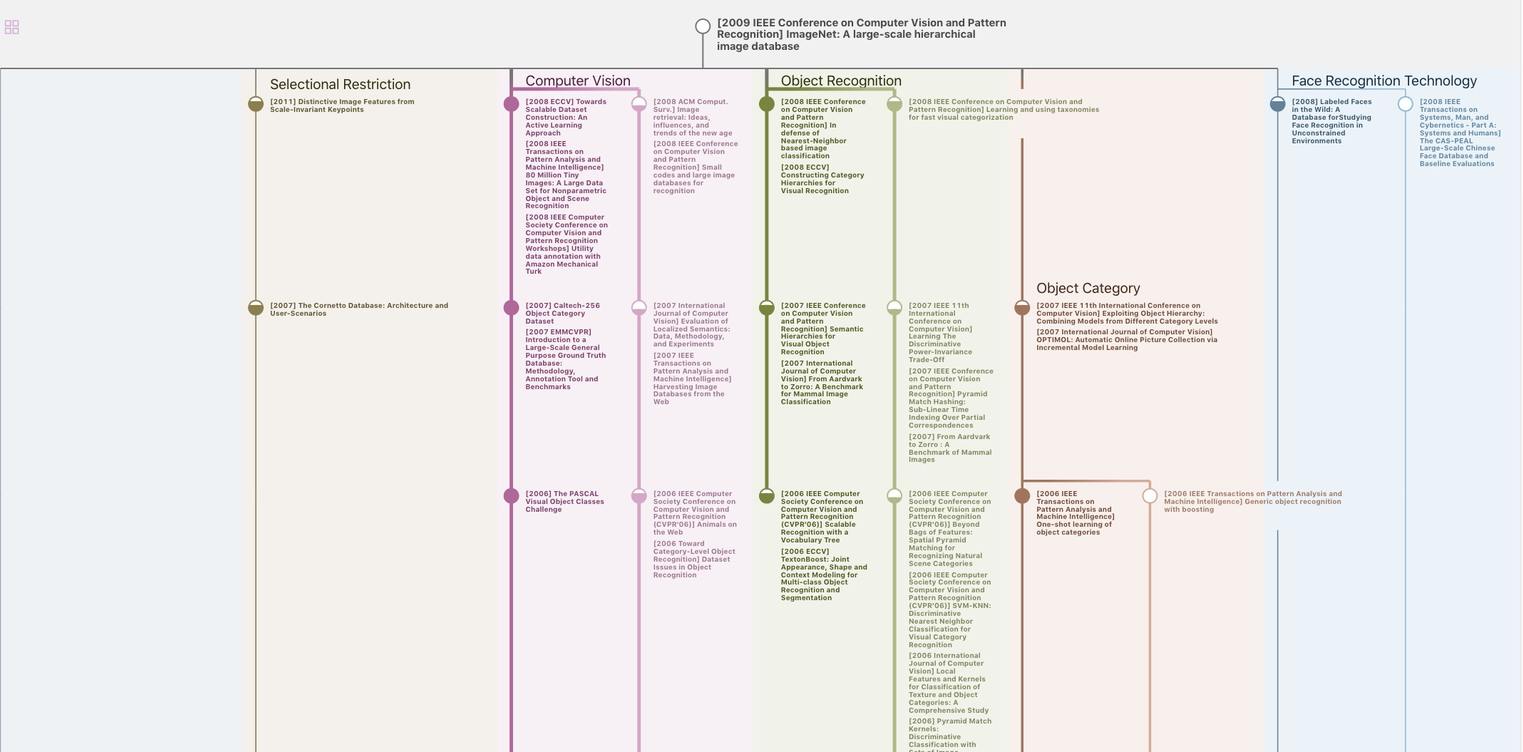
生成溯源树,研究论文发展脉络
Chat Paper
正在生成论文摘要