Probabilistic orthogonal-signal-corrected principal component analysis
KNOWLEDGE-BASED SYSTEMS(2023)
摘要
Much attention has been paid to reduce redundancy in data and extract discriminative features while preserving much of the relevant information. The technique of orthogonal signal correction (OSC) as a data processing tool removes directions of input features which are not correlated with the output variable. The PCA, on the other hand, has been successfully applied to problems in unsupervised dimensionality reduction of multivariate data. Application of PCA to orthogonal signal corrected data allows the resulting model to easily understand the relationships among variables and control the computational complexity. However, not being based upon a probability model, this procedure undergoes lack in statistical facilities to derive it such as maximum-likelihood estimation or model selection criteria. In this paper, we propose probabilistic orthogonal signal corrected principal component analysis (PO-PCA) which estimates the correct dimensionality based on a Bayesian approach. By formulating OSC and PCA as a probabilistic framework, we demonstrate how the whole procedure is determined by a maximum-likelihood solution of the density function. Similar to the Bayesian information criterion, we penalize the model for its complexity and therefore develop a model selection criterion for each of OSC and PCA, given enough observations. We present the experimental results of PO-PCA on simulation and real-life datasets, which illustrate the desired behavior of our probabilistic approach.(c) 2023 Elsevier B.V. All rights reserved.
更多查看译文
关键词
Orthogonal signal correction, Principal component analysis, Maximum-likelihood, Model selection, Near-infrared spectroscopy
AI 理解论文
溯源树
样例
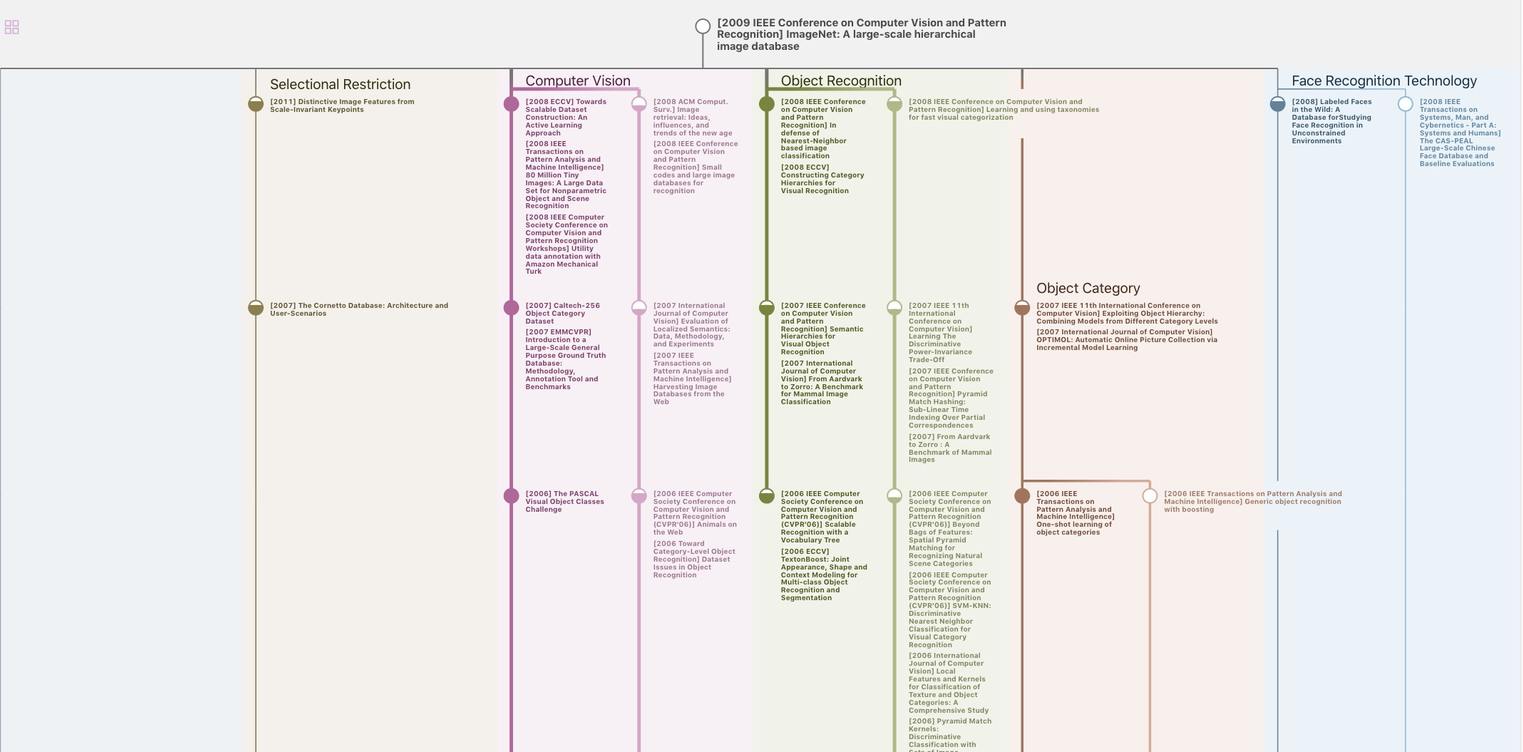
生成溯源树,研究论文发展脉络
Chat Paper
正在生成论文摘要