Approximately linear INGARCH models for spatio-temporal counts
JOURNAL OF THE ROYAL STATISTICAL SOCIETY SERIES C-APPLIED STATISTICS(2023)
摘要
Existing integer-valued generalised autoregressive conditional heteroskedasticity (INGARCH) models for spatio-temporal counts do not allow for negative parameter and autocorrelation values. Using approximately linear INGARCH models, the unified and flexible spatio-temporal (B)INGARCH framework for modelling unbounded (bounded) counts is proposed. These models combine negative dependencies with kinds of a long memory. They are easily adapted to special marginal features or cross-dependencies: When modelling precipitation data (counts of rainy hours), we account for zero-inflation, while for cloud-coverage data (counts of okta), we deal with missing data and additional cross-correlation. A copula related to the spatial error model shows an appealing performance.
更多查看译文
关键词
Gaussian copula, INGARCH models, soft clipping, softplus, spatial error model, spatio-temporal counts
AI 理解论文
溯源树
样例
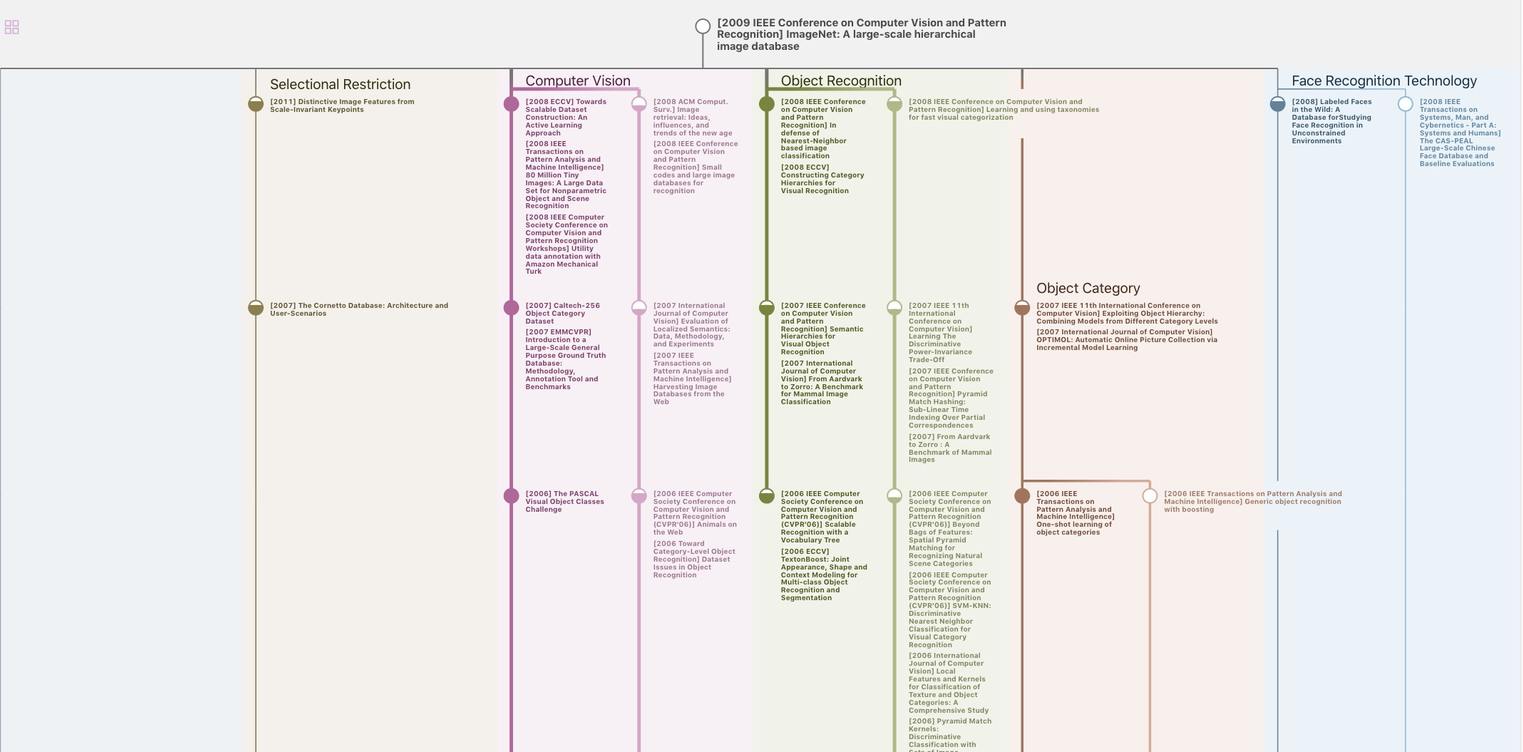
生成溯源树,研究论文发展脉络
Chat Paper
正在生成论文摘要