Semi-Supervised Active Learning for Anomaly Detection in Aviation
JOURNAL OF AEROSPACE INFORMATION SYSTEMS(2023)
摘要
Anomaly detection in commercial aviation is an extremely challenging yet crucial task. Accurately detecting operationally significant anomalies can save civilian lives and/or result in significant savings in maintenance cost. The current practice uses manually tuned rule-based mechanisms to flag exceedances from predefined safety boundaries. However, this system cannot identify unknown risks and emerging vulnerabilities. Recently, innovative approaches based on machine learning have been used to automate anomaly detection. However, there are limits to their applicability in the field of aviation due to several challenges: 1) Properly reviewed data are scarce in aviation and, as a result, supervised learning cannot reach optimal performance. 2) Operationally significant anomalies do not coincide with statistically significant ones and, as a result, unsupervised learning fails to provide reliable and robust performance. In this paper, we propose a semi-supervised active learning framework for anomaly detection (SALAD), which detects operationally significant anomalies in flight operational quality assurance data. The developed framework works with vast amounts of unlabeled data as well as a small quantity of labeled data reviewed by subject matter experts to reliably identify safety anomalies in flight operations. Moreover, the model's active learning strategy allows it to detect unknown anomalies that might emerge in the system. We validate the performance of the SALAD with a real-world case study of anomaly detection during the approach to landing of commercial aircraft. We specifically show that the proposed framework reaches reliable performance when only 1% of the data is labeled and can identify unknown anomalies effectively.
更多查看译文
AI 理解论文
溯源树
样例
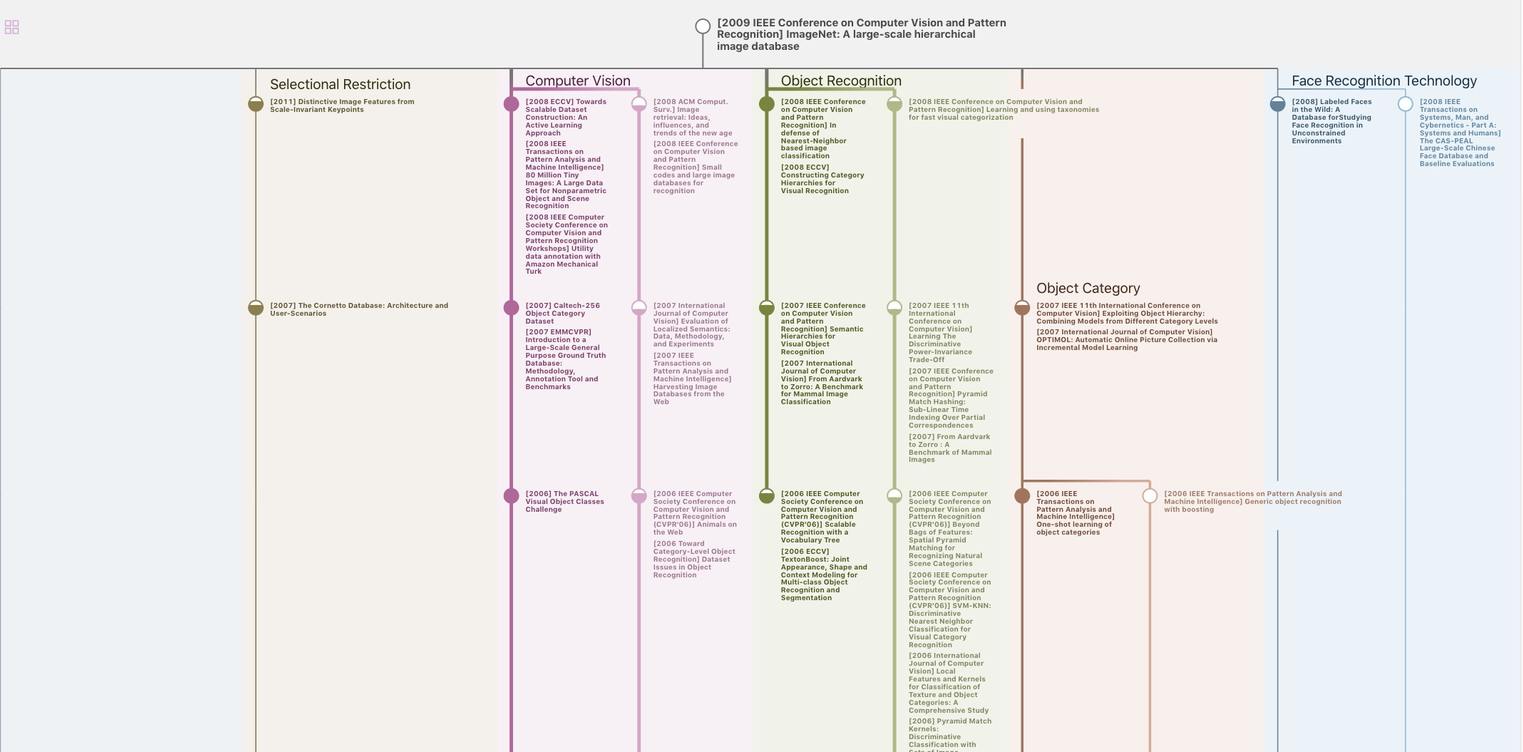
生成溯源树,研究论文发展脉络
Chat Paper
正在生成论文摘要