Deep Q-Network-Based Efficient Driving Strategy for Mixed Traffic Flow with Connected and Autonomous Vehicles on Urban Expressways
TRANSPORTATION RESEARCH RECORD(2023)
摘要
With the increase in the number of automated vehicles, roads will contain a mix of automated vehicles and human-driven vehicles. At present, rule-based driving strategy control of automated vehicles in mixed traffic flow makes it difficult to obtain optimal control. Therefore, this study proposes a learning-based driving strategy for connected and autonomous vehicles under mixed traffic flow. The proposed method differs from other driving strategies in two respects. First, both the lane-change and car-following policies are included, and the Deep Q-network algorithm is utilized to train the two policies in a mixed traffic-flow environment. Second, the proposed driving strategy considers both traffic efficiency and safety when designing the reward function. Through simulation experiments, the differences in traffic efficiency and safety of this method and the rule-based method were compared and analyzed under different traffic densities and penetration rates of connected and autonomous vehicles. Simulation results show that the driving strategy improves the average velocity (by 7.02 km/h) and traffic safety (especially in high-density traffic), compared with traditional rule-based driving strategy.
更多查看译文
关键词
mixed traffic flow,efficient driving strategy,urban expressways,autonomous vehicles,q-network-based
AI 理解论文
溯源树
样例
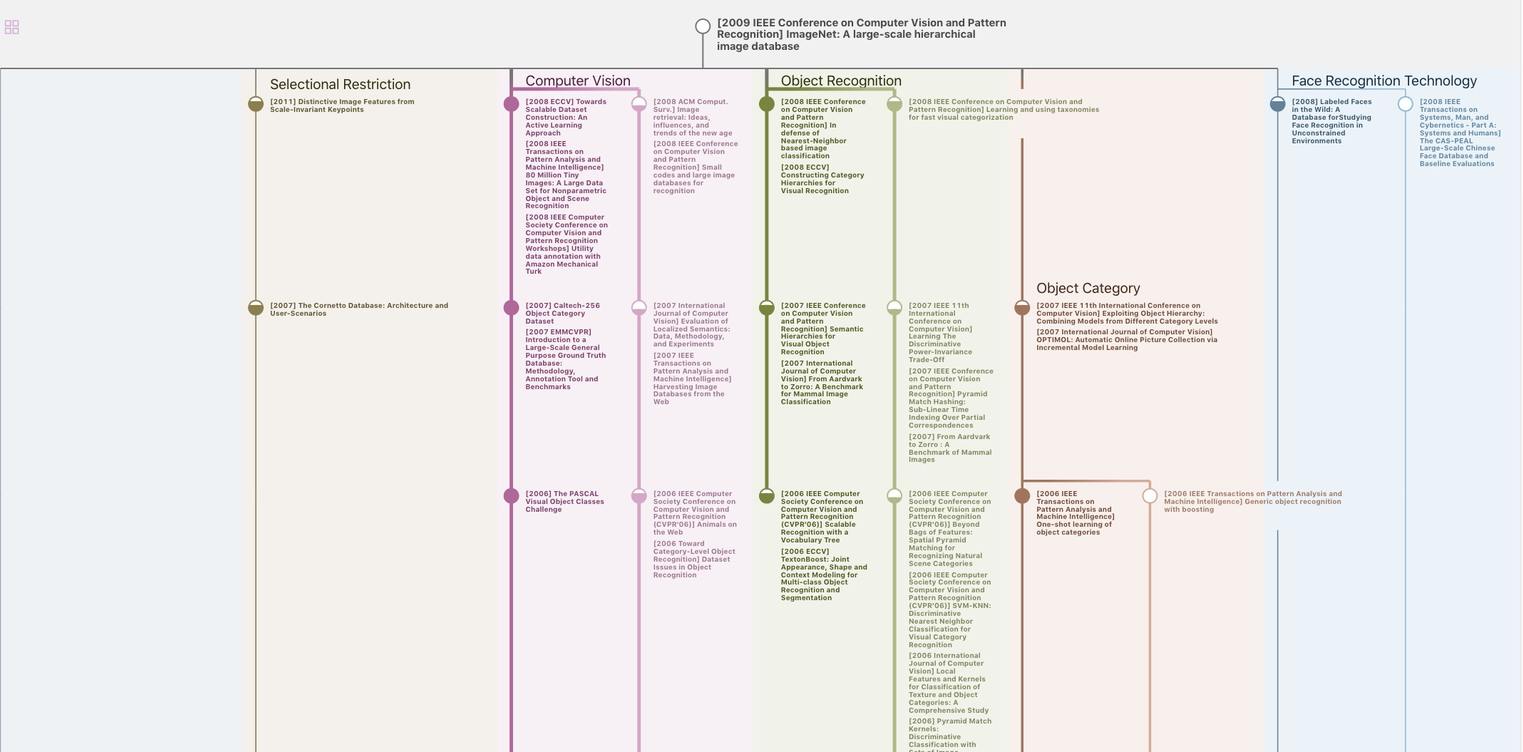
生成溯源树,研究论文发展脉络
Chat Paper
正在生成论文摘要