Hybrid modeling of hetero-agglomeration processes: a framework for model selection and arrangement
ENGINEERING WITH COMPUTERS(2024)
摘要
Modeling of hetero-agglomeration processes is invaluable for a variety of applications in particle technology. Traditionally, population balance equations (PBE) are employed; however, calculation of kinetic rates is challenging due to heterogeneous surface properties and insufficient material data. This study investigates how the integration of machine learning (ML) techniques-resulting in so-called hybrid models (HM)-can help to integrate experimental data and close this gap. A variety of ML algorithms can either be used to estimate kinetic rates for the PBE (serial HM) or to correct the PBE's output (parallel HM). As the optimal choice of the HM architecture is highly problem-dependent, we propose a general and objective framework for model selection and arrangement. A repeated nested cross-validation with integrated hyper-parameter optimization ensures a fair and meaningful comparison between different HMs. This framework was subsequently applied to experimental data of magnetic seeded filtration, where prediction errors of the pure PBE were reduced by applying the hybrid modeling approach. The framework helped to identify that for the given data set, serial outperforms parallel arrangement and that more advanced ML algorithms provide better interpolation ability. Additionally, it enables to draw inferences to general properties of the underlying PBE model and a statistical investigation of hyper-parameter optimization that paves the way for further improvements.
更多查看译文
关键词
Hetero-agglomeration,Population balance equations,Machine learning,Hybrid modeling,Hyper-parameter optimization,Repeated nested cross-validation
AI 理解论文
溯源树
样例
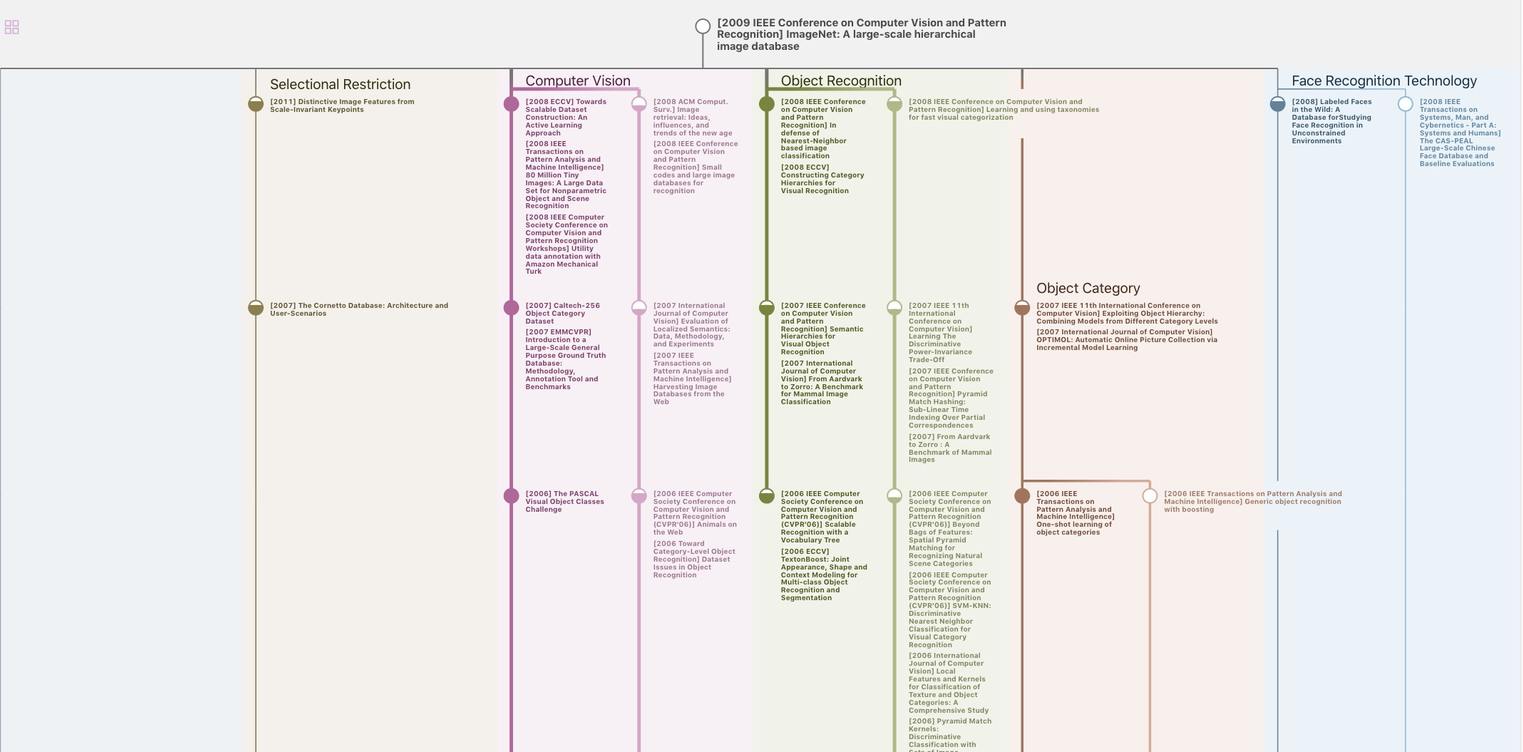
生成溯源树,研究论文发展脉络
Chat Paper
正在生成论文摘要