Automatic detection and characterization of porosities in cross-section images of metal parts produced by binder jetting using machine learning and image augmentation
JOURNAL OF INTELLIGENT MANUFACTURING(2024)
摘要
In binder jetting followed by sintering, the porosity characterization is critical to understand how the process affects the structure of the printed parts. Image-based porosity detection methods are widely used but the current solutions are limited to specific materials and conditions and require manual tuning that precludes real-time porosity detection. The application of machine learning for automating porosity detection has been also limited to specific materials and conditions and requires a large training dataset for successful implementation. However, large datasets are difficult to acquire experimentally in binder jetting due to prohibited material costs and experiment time. To bridge the knowledge gap, this paper investigates the application of machine learning on automated porosity detection using a small dataset consisting of highly varied cross-section images of metal parts produced by binder jetting followed by sintering. Stylegan3, a type of generative adversarial network, is used to increase the number of training images by image augmentation, and YOLOv5, a convolutional neural network specialized for object detection, is used to detect porosities. The resulting model achieves an F1 score of 88% and detection time of 3-15 ms per image. Generalized porosity detection is also assessed on a set of images containing highly varied materials, resolutions, magnifications, and pore densities. Furthermore, morphological information of the classified porosities such as the distribution of their orientations are automatically extracted using image processing algorithms.
更多查看译文
关键词
Porosity,Binder jetting,Sintering,Machine learning,CNN,Image augmentation
AI 理解论文
溯源树
样例
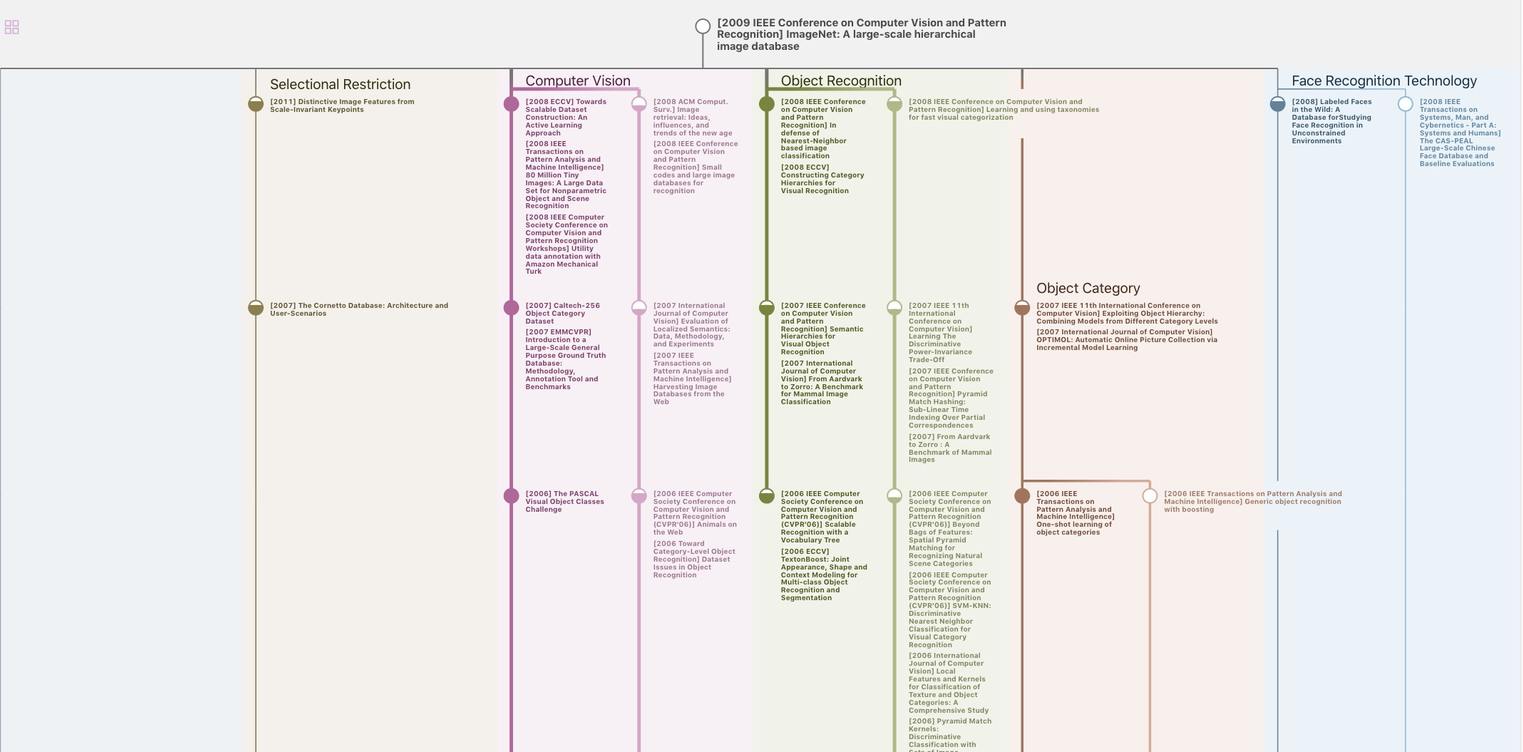
生成溯源树,研究论文发展脉络
Chat Paper
正在生成论文摘要