Numerical Analysis of Low-Cost Optimization Measures for Improving Energy Efficiency in Residential Buildings
Energy(2023)
摘要
Low-cost optimization measures often show an energy efficiency potential that, if correctly exploited, can reduce the implementation of more invasive and costly retrofitting interventions. However, they are rarely systematized due to the lack of a dedicated data collection process together with the absence of optimal implementation strategies from an energy efficiency perspective. In this work, a quantitative analysis was conducted using a unique dataset containing 92 residential buildings in order to identify the set of actions leading to the highest energy savings. An Artificial Neural Network is trained over a dedicated dataset and the complex relation “Optimization measures-Energy Saving” is modeled as black-box. Sensitivity indices are computed to analyze and quantify the influence of each measure on the variability of the energy consumption, while metamodel-based samples are employed to identify the most probable intervals of energy savings and the probability of success associated with each optimization measure. As results, the most effective optimization measures accounting for the inherent uncertainty in the model and weather conditions are identified. In particular, the best LICOMs can achieve a median monthly energy saving of about 2%, which is four times the median of all the monthly energy saving events for the analyzed building stock. The adopted metamodel-based approach is suitable for modeling each input–output relation and it can be efficiently employed to perform advanced sensitivity analyses by creating synthetic data. The possibility of quantifying expected energy savings of an optimization measure as well as its chances of success is of strategic importance for targeted decision making.
更多查看译文
关键词
Low-cost energy optimization measures,Energy efficiency,Surrogate model,Building energy optimization
AI 理解论文
溯源树
样例
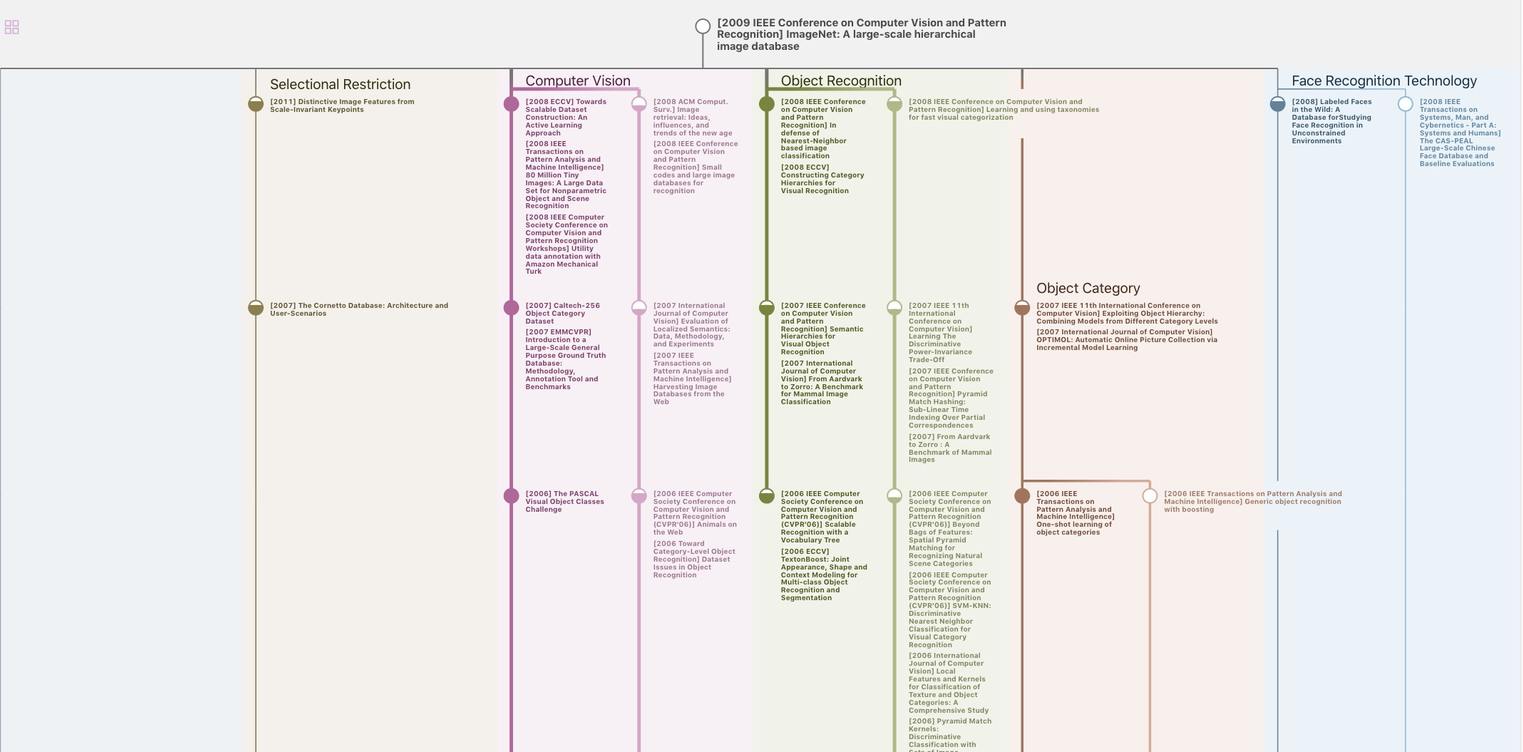
生成溯源树,研究论文发展脉络
Chat Paper
正在生成论文摘要