Synthetic data: the future of open-access health-care datasets?
LANCET(2023)
摘要
In modern health care, medical datasets are increasingly being used to improve patient care, including through population health analysis and the development of diagnostic machine learning algorithms. This trend has been a key driver for the development of open-access datasets, giving researchers access to local or national data shared by different institutions. Open-access data can be used to train machine learning algorithms on diverse datasets that have been carefully curated, or to test algorithms that have already been trained by researchers elsewhere to assess their performance when applied to new data. An increasing volume of patient data is being shared in the form of open-access datasets, with reasonable precautions being taken to anonymise the data. For example, numerous datasets are freely available online with thousands of retinal fundus photos, electrocardiograms, or electroencephalograms, which have been used to drive machine learning research in the corresponding specialties. Open-access datasets have largely been a positive force, enabling researchers to access datasets that would be infeasible to create locally. However, it is becoming increasingly apparent that machine learning algorithms can identify patient characteristics that are completely indiscernible to humans when presented with the same imaging. In ophthalmology, algorithms have identified age, sex, blood pressure, and smoking status from simple fundus photos;1Wagner SK Fu DJ Faes L et al.Insights into systemic disease through retinal imaging-based oculomics.Transl Vis Sci Technol. 2020; 9: 6Crossref PubMed Scopus (59) Google Scholar in cardiology, algorithms have identified age, sex, COVID-19 status, and diabetes status from electrocardiograms;2Topol EJ What's lurking in your electrocardiogram?.Lancet. 2021; 397: 785Summary Full Text Full Text PDF PubMed Scopus (1) Google Scholar and in neurology, algorithms have identified specific individuals from electroencephalograms.3Marcel S Millán JDR Person authentication using brainwaves (EEG) and maximum a posteriori model adaptation.IEEE Trans Pattern Anal Mach Intell. 2007; 29: 743-752Crossref PubMed Scopus (385) Google Scholar These tasks go beyond human abilities, which is why clinicians and researchers assumed that these forms of data were anonymous. For open-access datasets, very limited personally identifiable information should be released and demographic data associated with imaging are often withheld. However, the ability of modern machine learning algorithms to extract personally identifiable data from routine imaging raises questions as to whether existing datasets are truly anonymous. Few methods exist to address this issue, and perhaps the most promising one is the use of synthetic data. The generation of highly realistic synthetic data with artificial intelligence is an area of research that is still in its infancy; however, the quality of the generative algorithms has improved over time, and it is now increasingly possible to replace real datasets with synthetic data.4Chen RJ Lu MY Chen TY Williamson DFK Mahmood F Synthetic data in machine learning for medicine and healthcare.Nat Biomed Eng. 2021; 5: 493-497Crossref PubMed Scopus (101) Google Scholar, 5Arora A Arora A Generative adversarial networks and synthetic patient data: current challenges and future perspectives.Future Healthc J. 2022; 9: 190-193Crossref PubMed Google Scholar As the rate of releasing open-access datasets continues to accelerate, perhaps we should be rethinking these datasets. I have honorary research affiliations with NHS England and NHS Improvement and with Moorfields Eye Hospital. I am an academic panel and committee member of Health Data Research UK and the National Institute for Health and Care Research.
更多查看译文
AI 理解论文
溯源树
样例
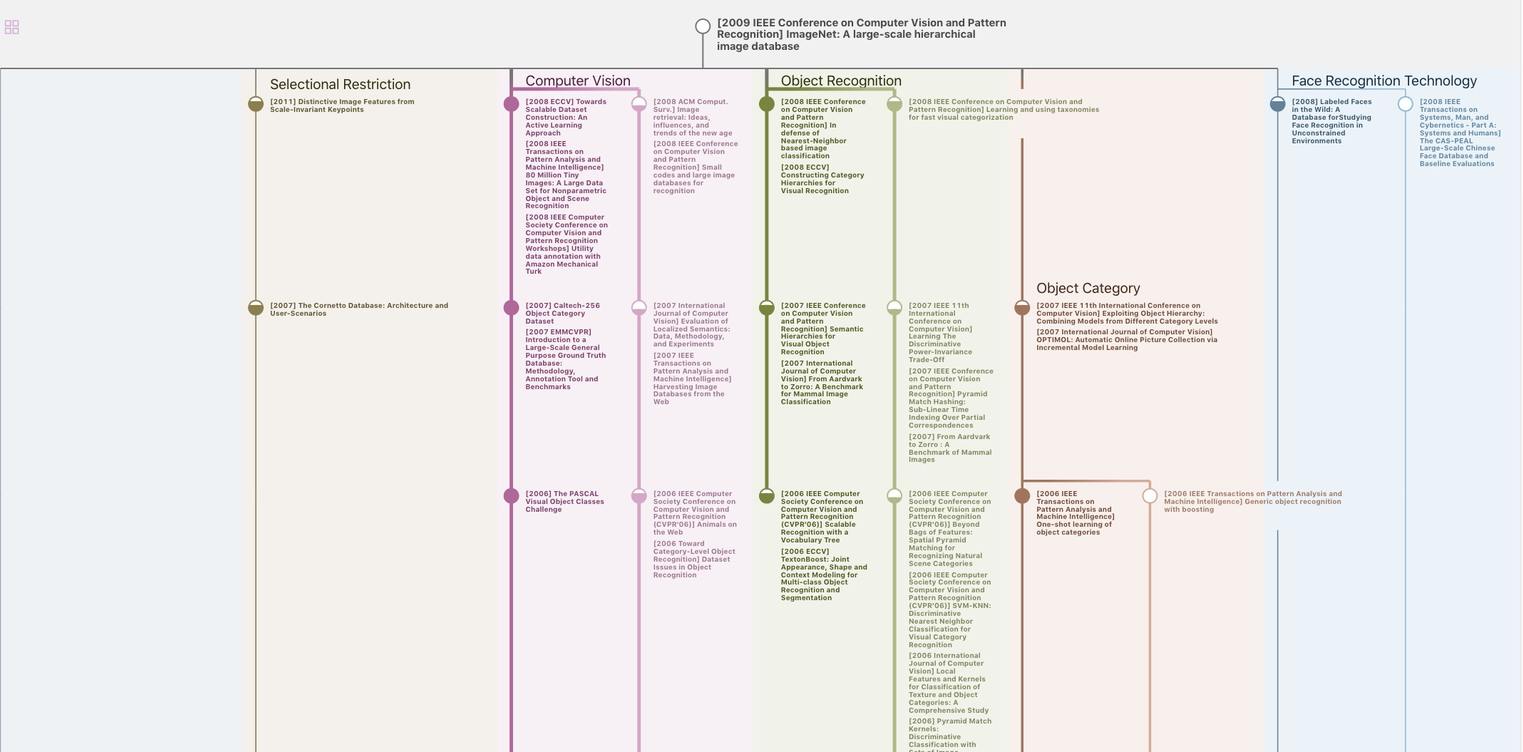
生成溯源树,研究论文发展脉络
Chat Paper
正在生成论文摘要