High Throughput Screening of Single Atomic Catalysts with Optimized Local Structures for the Electrochemical Oxygen Reduction by Machine Learning
Journal of Energy Chemistry/Journal of energy chemistry(2023)
摘要
Single atomic catalysts (SACs), especially metal-nitrogen doped carbon (M-NC) catalysts, have been extensively explored for the electrochemical oxygen reduction reaction (ORR), owing to their high activity and atomic utilization efficiency. However, there is still a lack of systematic screening and optimization of local structures surrounding active centers of SACs for ORR as the local coordination has an essential impact on their electronic structures and catalytic performance. Herein, we systematic study the ORR catalytic performance of M-NC SACs with different central metals and environmental atoms in the first and second coordination sphere by using density functional theory (DFT) calculation and machine learning (ML). The geometric and electronic informed overpotential model (GEIOM) based on random forest algorithm showed the highest accuracy, and its R2 and root mean square errors (RMSE) were 0.96 and 0.21, respectively. 30 potential high-performance catalysts were screened out by GEIOM, and the RMSE of the predicted result was only 0.12 V. This work not only helps us fast screen high-performance catalysts, but also provides a low-cost way to improve the accuracy of ML models.(c) 2023 Science Press and Dalian Institute of Chemical Physics, Chinese Academy of Sciences. Published by ELSEVIER B.V. and Science Press. All rights reserved.
更多查看译文
关键词
Single atomic catalysts,Coordination sphere,High throughput screening,Machine learning,Oxygen reduction reaction
AI 理解论文
溯源树
样例
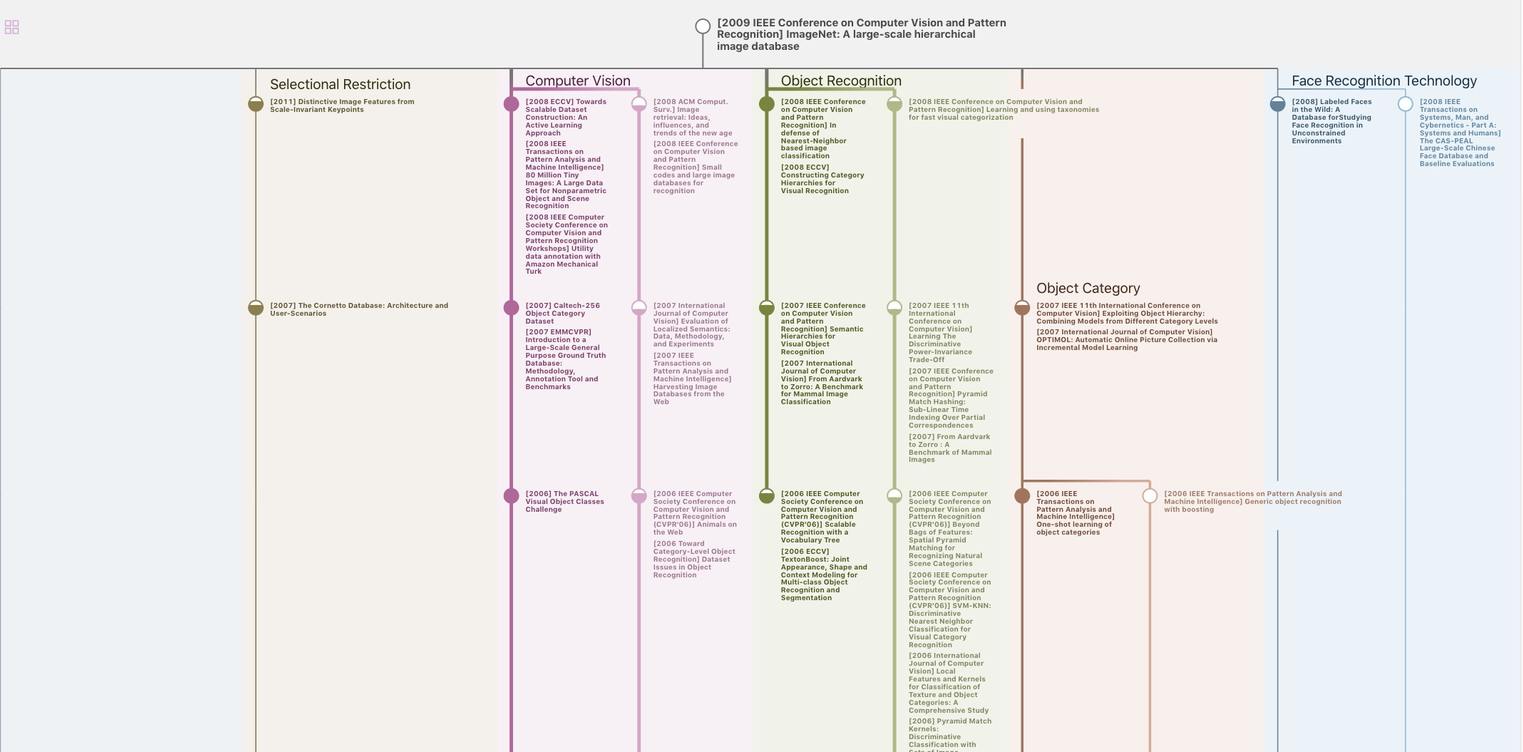
生成溯源树,研究论文发展脉络
Chat Paper
正在生成论文摘要