Evaluation of dual-weighted residual and machine learning error estimation for projection-based reduced-order models of steady partial differential equations
COMPUTER METHODS IN APPLIED MECHANICS AND ENGINEERING(2023)
摘要
Projection-based reduced-order models (pROMs) show great promise as a means to accelerate many-query applications such as forward error propagation, solving inverse problems, and design optimization. In order to deploy pROMs in the context of high-consequence decision making, accurate error estimates are required to determine the region(s) of applicability in the parameter space. The following paper considers the dual-weighted residual (DWR) error estimate for pROMs and compares it to another promising pROM error estimate, machine learned error models (MLEM). In this paper, we show how DWR can be applied to ROMs and then evaluate DWR on two partial differential equations (PDEs): a two-dimensional linear convection- reaction-diffusion equation, and a three-dimensional static hyper-elastic beam. It is shown that DWR is able to estimate errors for pROMs extrapolating outside of their training set while MLEM is best suited for pROMs used to interpolate within the pROM training set.(c) 2023 Elsevier B.V. All rights reserved.
更多查看译文
关键词
steady partial differential equations,error estimation,models,dual-weighted,projection-based,reduced-order
AI 理解论文
溯源树
样例
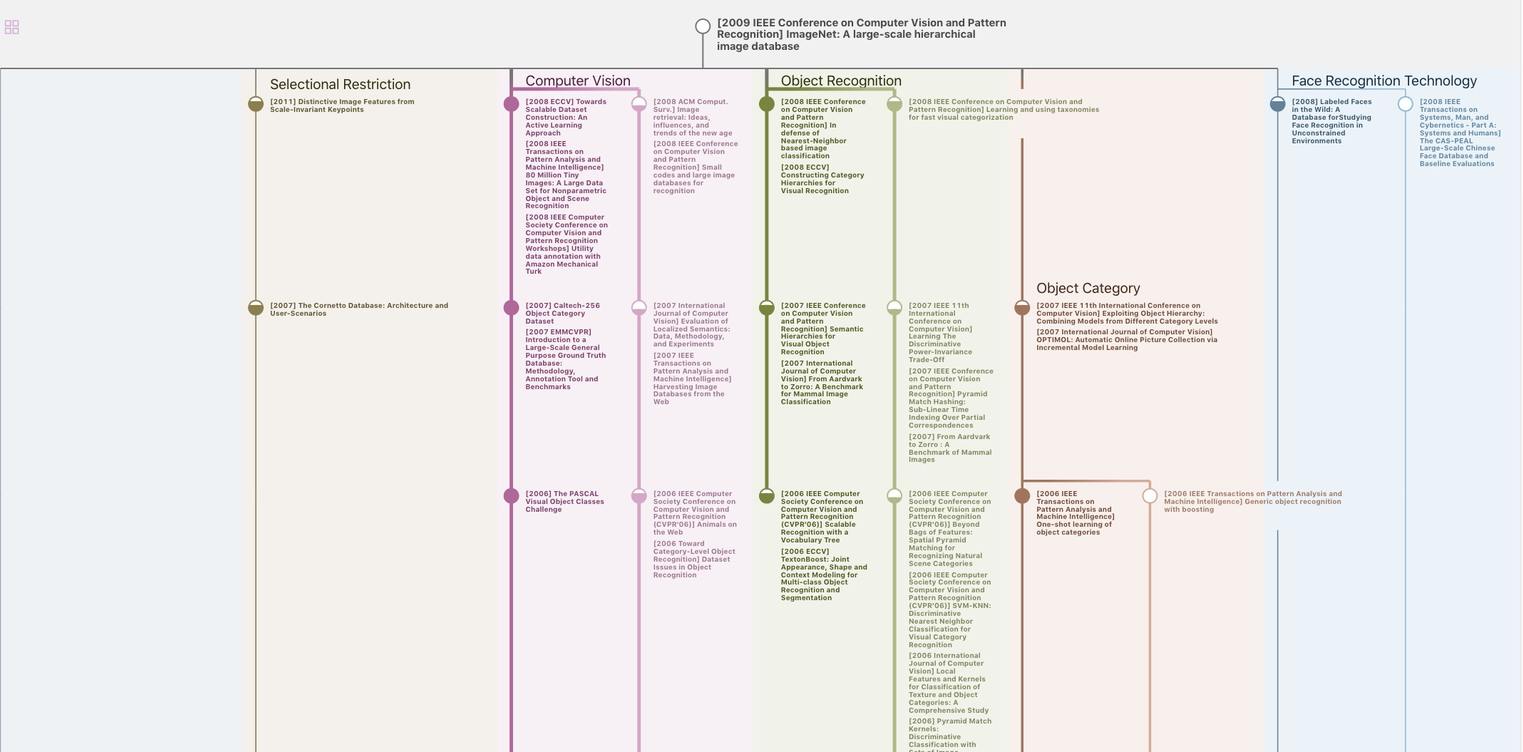
生成溯源树,研究论文发展脉络
Chat Paper
正在生成论文摘要