A multi-fault diagnostic method based on category-reinforced domain adaptation network for series-connected battery packs
JOURNAL OF ENERGY STORAGE(2023)
摘要
For the intelligent development and safe operation of electric vehicles, it is really of critical importance to quickly detect and accurately distinguish different types of faults in battery packs. However, the fault characteristics of lithium-ion battery packs with different battery types and different health conditions are difficult to discriminate, and domain-adapted neural networks perform well in this regard. Based on this, a multi-fault detection method based on Category-Reinforced Domain Adaptation Network for series-connected battery packs was proposed by integrating the characteristics of Attentional Mechanisms and Domain Adaptation Neural Network, which can diagnose several types of faults (i.e. voltage imbalance, the internal short circuit, sensor faults, Sensor drift voltage, and Random fluctuation). Through platform validation in real environment in three different working conditions, it is verified that the Domain Neural Network combined with the attention mechanism can effectively improve the generalization performance of the model in different battery packs and has an obvious effect on the diagnosis of multiple faults in battery packs.
更多查看译文
关键词
domain adaptation network,battery packs,multi-fault,category-reinforced,series-connected
AI 理解论文
溯源树
样例
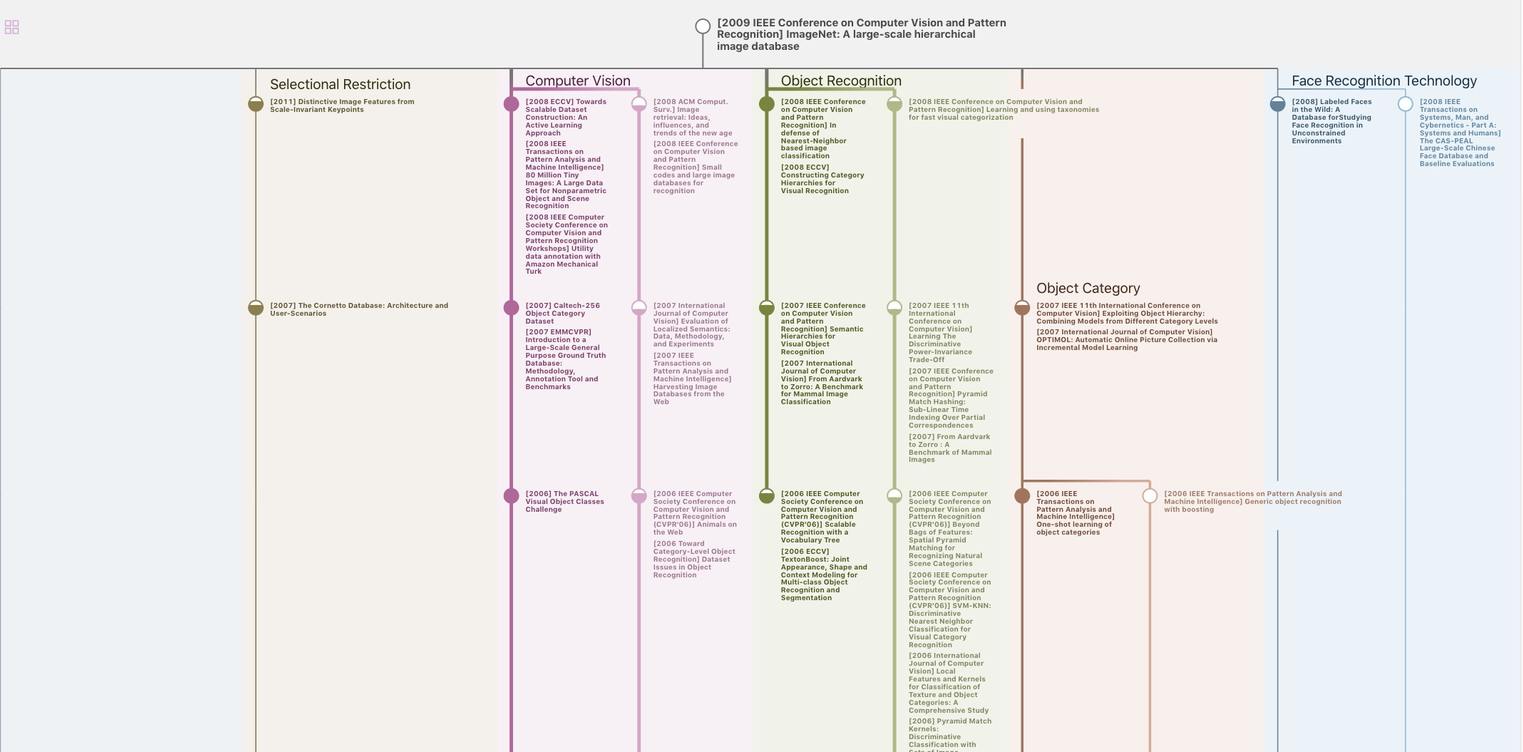
生成溯源树,研究论文发展脉络
Chat Paper
正在生成论文摘要