Comparison of geostatistical and deep-learning inversion methods for DNAPL source zone architecture characterization
HYDROGEOLOGY JOURNAL(2023)
摘要
High-resolution site characterization of hydraulic properties and source zone architecture (SZA) are essential for performing risk assessment and designing remediation strategies for dense nonaqueous phase liquid (DNAPL) contamination. DNAPL SZA characterization is challenging because of the highly correlated unknown states and parameters, namely the spatial distribution of DNAPL saturation ( S N ) and hydraulic conductivity ( K ). Two methods can be used for the inversion of highly correlated parameters, i.e., geostatistical inversion with and without parameter-state cross-correlation. In this study, numerical experiments considering weak and strong heterogeneity for S N and K are used to compare the performance of the two geostatistical inversion methods and the deep-learning-based correlation method. Results show that the inversion with combined parameter-state cross-correlation successfully estimated both the K and DNAPL SZA for a weakly heterogeneous K field, but fails to reproduce the overall morphology of the DNAPL SZA for a strongly heterogeneous K field. In comparison, the inversion without parameter-state cross-correlation can robustly capture but over-smooth the detailed features of the K and DNAPL SZA, due to the deviation of the highly correlated K – S N fields from the simplified prior cross-covariance. The deep-learning-based method consistently outperformed the inversion strategies with and without the parameter-state cross-correlation, in terms of computational efficiency and estimation accuracy for both the simple and the complex DNAPL SZA, since it can implicitly capture the K – S N interdependence and the physical patterns of the DNAPL SZA without explicitly coupling the multiphase model to account for the K – S N correlation.
更多查看译文
关键词
Geostatistics,Non-aqueous phase liquids (NAPLs),Deep learning-based inversion,Data assimilation,Highly correlated parameters inversion
AI 理解论文
溯源树
样例
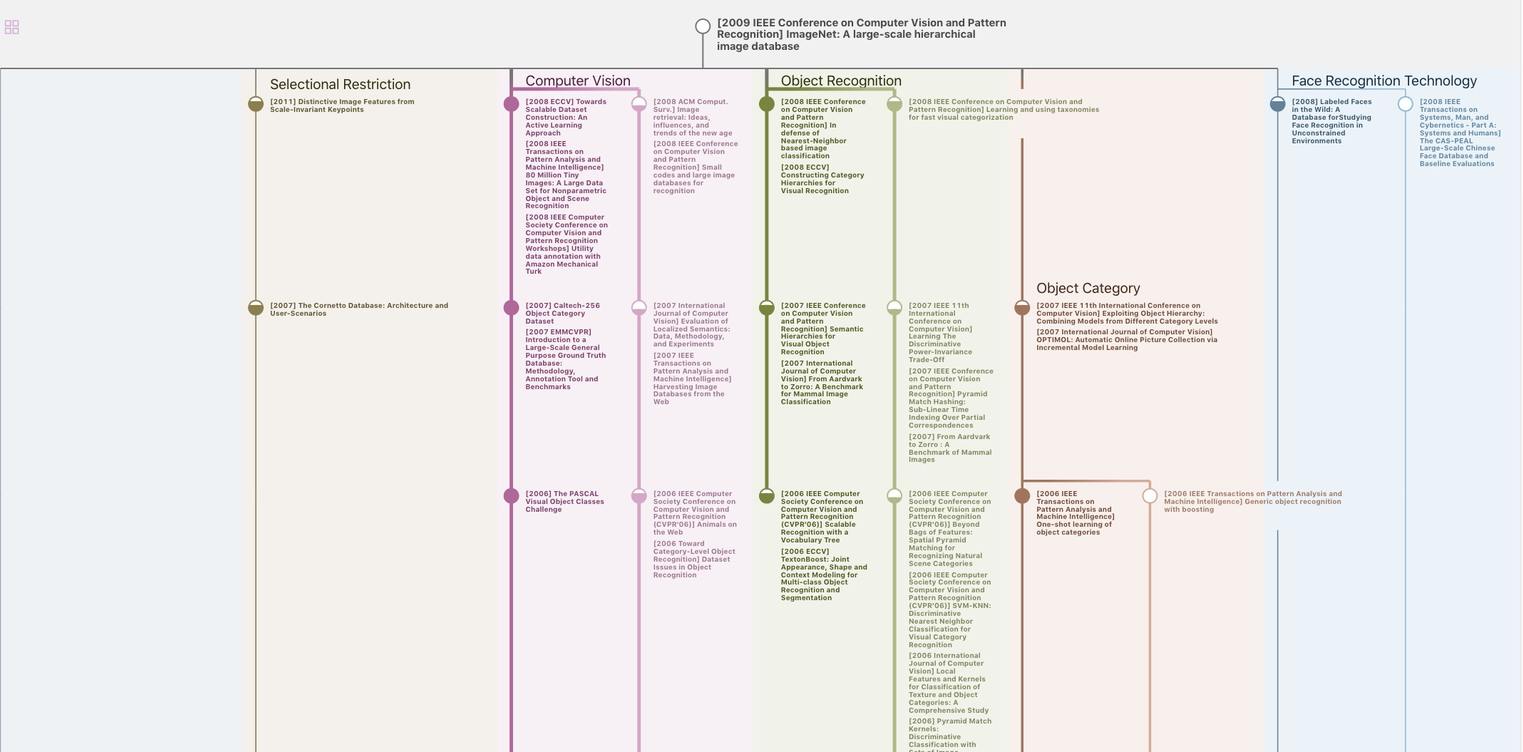
生成溯源树,研究论文发展脉络
Chat Paper
正在生成论文摘要