Optimal Identification of Unknown Parameters of Photovoltaic Models Using Dual-Population Gaining-Sharing Knowledge-Based Algorithm
INTERNATIONAL JOURNAL OF INTELLIGENT SYSTEMS(2023)
摘要
Establishing an accurate equivalent model is a critical foundation to describe the energy conversion characteristics of a photovoltaic system, which can support the research of fault analysis, output power prediction, and performance analysis of the photovoltaic system. However, the widely used equivalent models are highly nonlinear and have many unknown parameters, making it difficult to identify these parameters accurately. Our previous work found that the gaining-sharing knowledge-based algorithm (GSK) shows promising performance in solving this problem. But its efficacy is not enough to achieve accurate parameters within a relatively limited computing resource. In this context, a dual-population GSK algorithm (DPGSK), which introduces a dual-population evolution strategy for more excellent searchability, is proposed to address this issue. In each iteration, the population splits equally and randomly into two subpopulations, one of which performs the junior gaining-sharing phase while the other performs the senior gaining-sharing phase. Then two updated subpopulations merge to form a new population. This allows for a grand reconciliation of convergence speed and population diversity, giving DPGSK powerful optimization performance. Afterward, DPGSK is applied to five photovoltaic models and validated for performance against other advanced metaheuristics. Besides, the impact of different components on DPGSK is also investigated. Results and comparisons show that either component is indispensable to DPGSK, and DPGSK strengthens the convergence and achieves accurate and reliable results, demonstrating its superiority over other algorithms in solving this studied problem.
更多查看译文
关键词
photovoltaic models,optimal identification,dual-population,gaining-sharing,knowledge-based
AI 理解论文
溯源树
样例
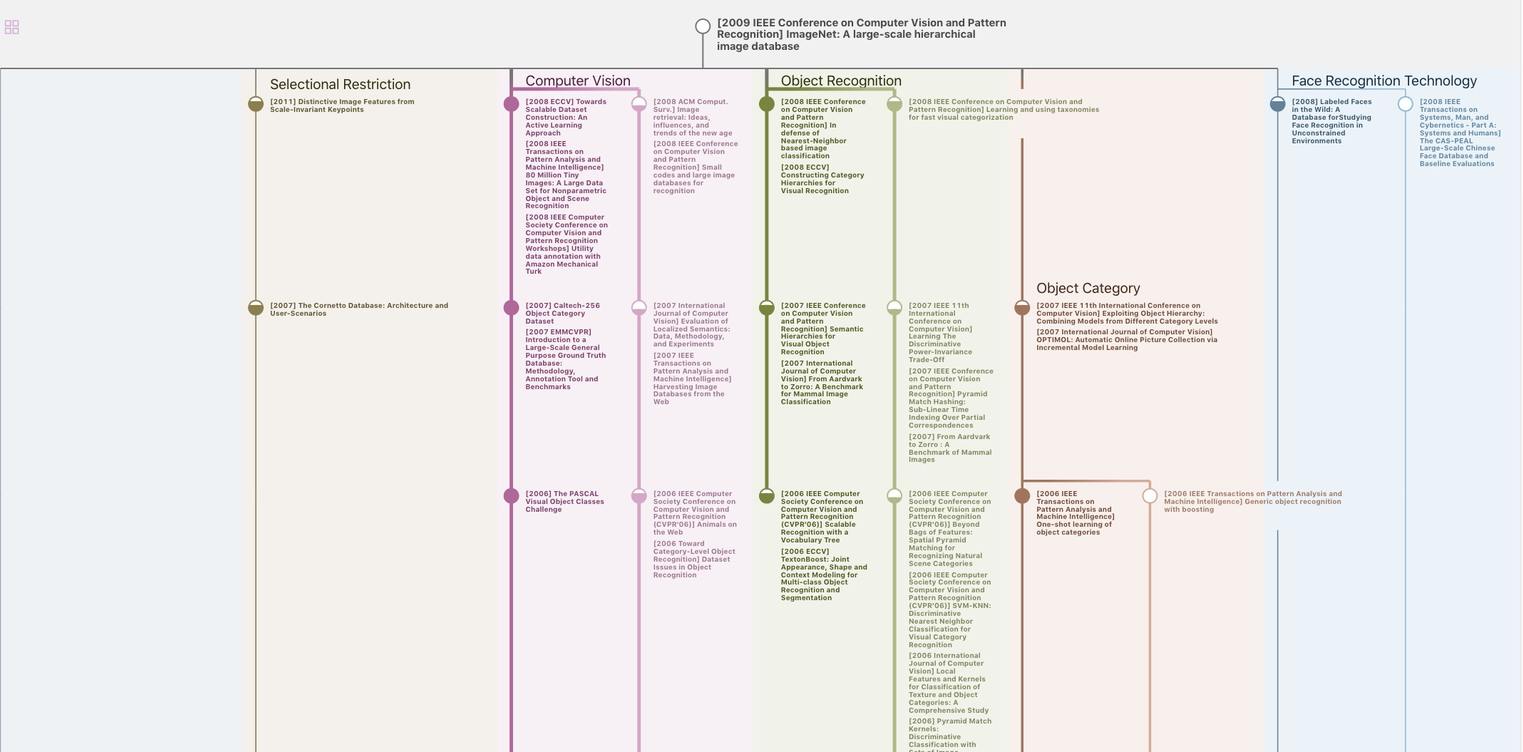
生成溯源树,研究论文发展脉络
Chat Paper
正在生成论文摘要