How fast do we forget our past social interactions? Understanding memory retention with parametric decays in relational event models
NETWORK SCIENCE(2023)
摘要
In relational event networks, endogenous statistics are used to summarize the past activity between actors. Typically, it is assumed that past events have equal weight on the social interaction rate in the (near) future regardless of the time that has transpired since observing them. Generally, it is unrealistic to assume that recently past events affect the current event rate to an equal degree as long-past events. Alternatively one may consider using a prespecified decay function with a prespecified rate of decay. A problem then is that the chosen decay function could be misspecified yielding biased results and incorrect conclusions. In this paper, we introduce three parametric weight decay functions (exponential, linear, and one-step) that can be embedded in a relational event model. A statistical method is presented to decide which memory decay function and memory parameter best fit the observed sequence of events. We present simulation studies that show the presence of bias in the estimates of effects of the statistics whenever the decay, as well as the memory parameter, are not properly estimated, and the ability to test different memory models against each other using the Bayes factor. Finally, we apply the methodology to two empirical case studies.
更多查看译文
关键词
relational event model,social network analysis,network dynamics,memory decay,memory retention process,profile likelihood,Bayes factor
AI 理解论文
溯源树
样例
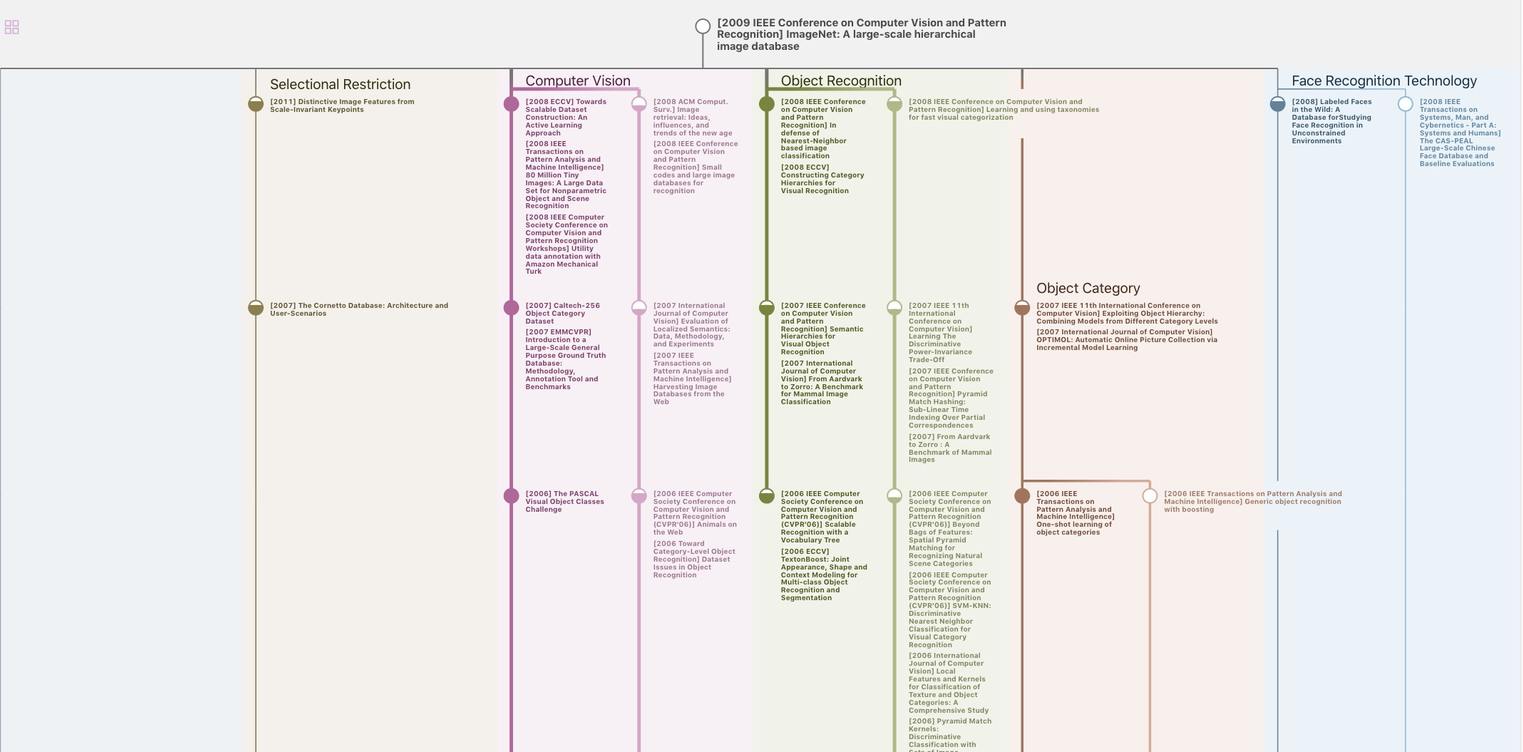
生成溯源树,研究论文发展脉络
Chat Paper
正在生成论文摘要