TALK: Tracking Activities by Linking Knowledge
ENGINEERING APPLICATIONS OF ARTIFICIAL INTELLIGENCE(2023)
摘要
Dependable and accurate monitoring of elderly at home becomes crucial to limit both the costs and human efforts of following up elderly for establishing a healthy care system. Human Activity Recognition (HAR) tools, based on sensors installed in smart homes, will become an important tool to provide useful information to the caregiver when something happens in the house of an elderly and care is required. The current available detection tools either exist out of interpretable knowledge-driven techniques or scalable data-driven ones. In this paper, a hybrid methodology that combines both approaches is designed and evaluated to Track Activities by Linking Knowledge (TALK). Both sensor data and their link to the relevant domain knowledge about where those sensors are installed, the performed activities that occur, and how the household is constructed, are generalized in a specific knowledge graph (KG) structure to represent continuous events. The interpretable knowledge graph embedding technique Instance Neighboring using Knowledge (INK) is then used to transform these events inside the KG to a tabular format, which can be used by any traditional machine learning classifier to create a HAR tool. The TALK methodology is evaluated on two HAR datasets and shows (a) that TALK outperforms both traditional automated data-driven as well as knowledge-driven techniques in terms of predictive performance, and (b) how TALK can be easily used in a more out of lab environment. All these results and the interpretable aspects show that TALK can become an important tool to monitor elderly in their homes efficiently, effectively and with less intrusive techniques.
更多查看译文
关键词
Human Activity Recognition (HAR),Hybrid Artificial Intelligence (AI),Knowledge Graph (KG) embedding,Ambient living,Smart monitoring,eHealth
AI 理解论文
溯源树
样例
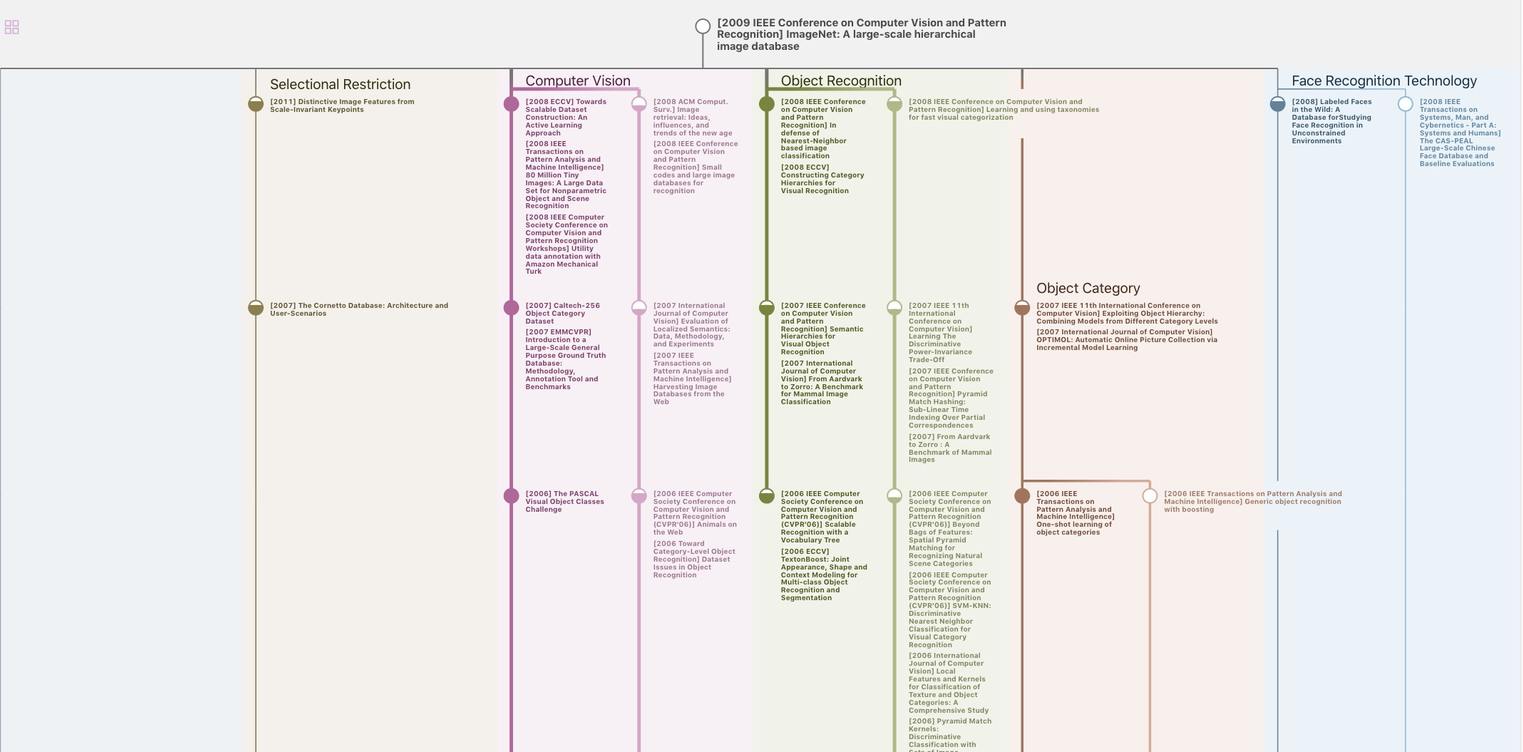
生成溯源树,研究论文发展脉络
Chat Paper
正在生成论文摘要