Emerging Memory Devices Beyond Conventional Data Storage: Paving the Path for Energy-Efficient Brain-Inspired Computing
ELECTROCHEMICAL SOCIETY INTERFACE(2023)
摘要
The current state of neuromorphic computing broadly encompasses domain-specific computing architectures designed to accelerate machine learning (ML) and artificial intelligence (AI) algorithms. As is well known, AI/ML algorithms are limited by memory bandwidth. Novel computing architectures are necessary to overcome this limitation. There are several options that are currently under investigation using both mature and emerging memory technologies. For example, mature memory technologies such as high-bandwidth memories (HBMs) are integrated with logic units on the same die to bring memory closer to the computing units. There are also research efforts where in-memory computing architectures have been implemented using DRAMs or flash memory technologies. However, DRAMs suffer from scaling limitations, while flash memory devices suffer from endurance issues. Additionally, in spite of this significant progress, the massive energy consumption needed in neuromorphic processors while meeting the required training and inferencing performance for AI/ML algorithms for future applications needs to be addressed. On the AI/ML algorithm side, there are several pending issues such as life-long learning, explainability, context-based decision making, multimodal association of data, adaptation to address personalized responses, and resiliency. These unresolved challenges in AI/ML have led researchers to explore brain-inspired computing architectures and paradigms.
更多查看译文
关键词
memory devices,conventional data storage,energy-efficient,brain-inspired
AI 理解论文
溯源树
样例
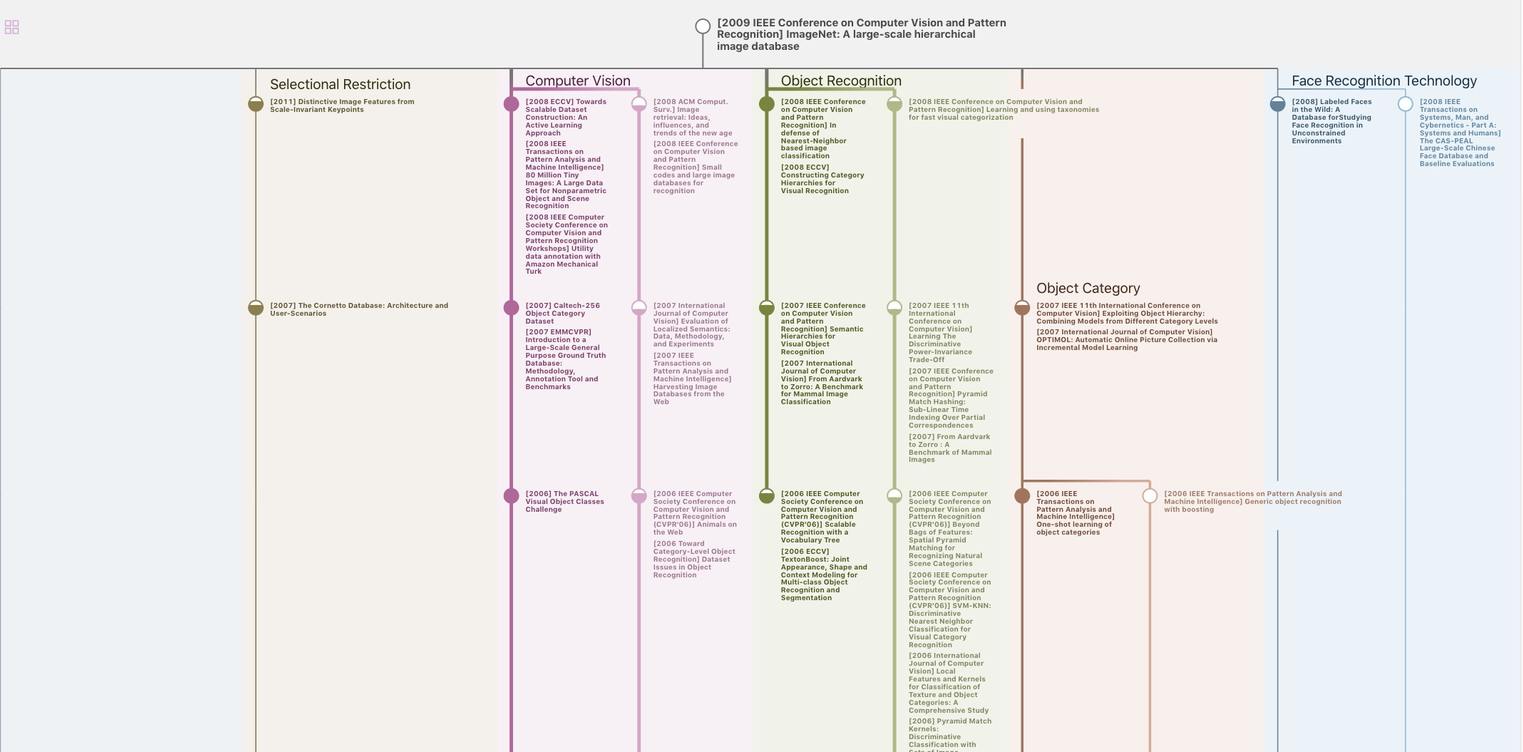
生成溯源树,研究论文发展脉络
Chat Paper
正在生成论文摘要