A comparative study of cellular traffic prediction mechanisms
WIRELESS NETWORKS(2023)
摘要
The rapid growth of cellular data traffic is creating a variety of challenges that will only be accentuated with the consolidation of 5G networks and the emergence of 6G networks. In order to address those challenges, prediction network management techniques, and in particular proactive network management and cellular traffic prediction will be fundamental. In this paper, we explore different research works in this area and provide a comprehensive survey. Moreover, we detail the principles of multiple statistical, machine learning, and deep learning mechanisms that can be utilized in order to address the cellular traffic prediction problem, and we evaluate their performance in the same dataset. The experimental results show that the Feedforward Neural Network model is capable of providing the best performance among the studied models for 1-step-ahead and 10-step-ahead predictions. In the case of longer-term predictions (i.e. 20-step-ahead), the performance of statistical models, such as Autoregression and Autoregression Moving Average, is shown to be superior to the other models.
更多查看译文
关键词
Statistical learning,Machine learning,Deep learning,Cellular traffic,Predictive network management
AI 理解论文
溯源树
样例
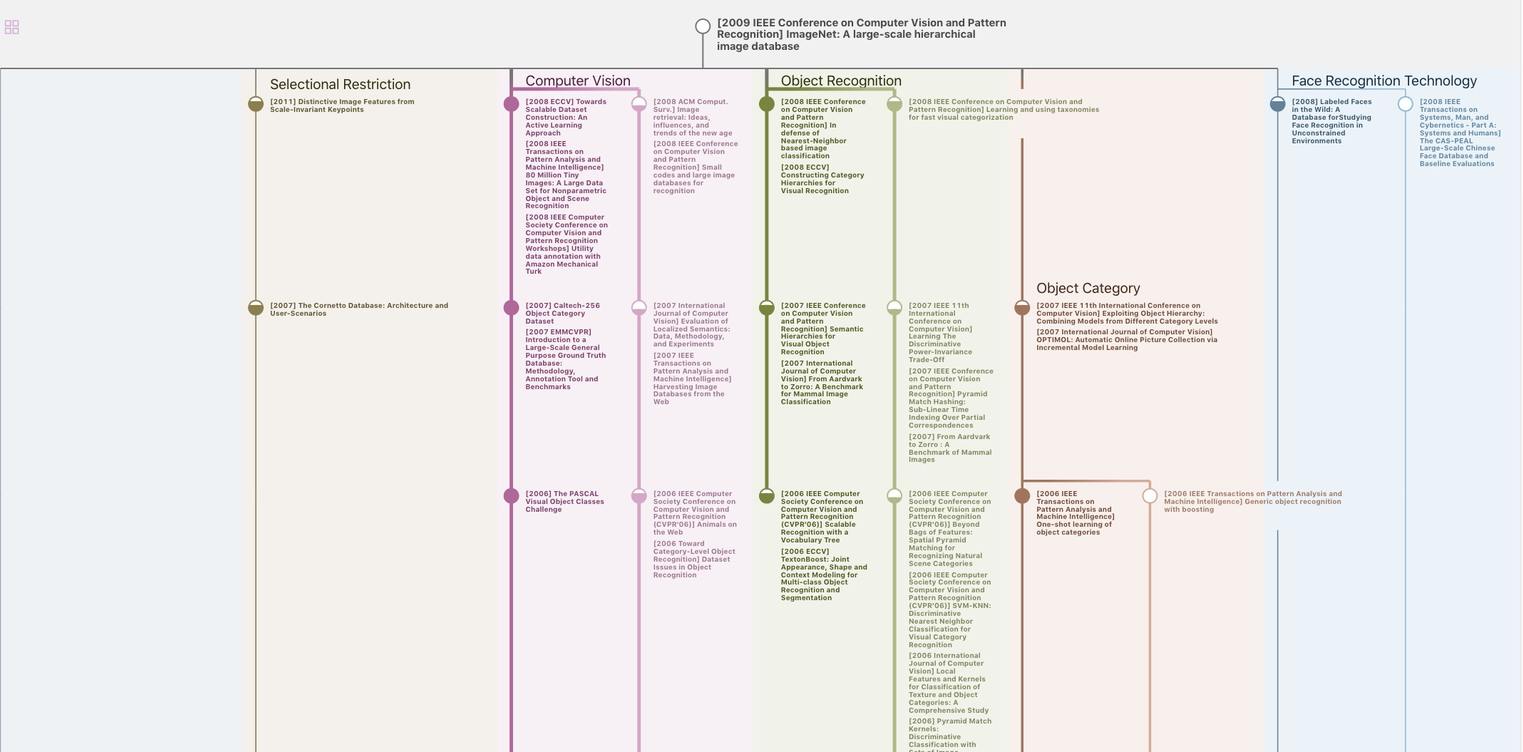
生成溯源树,研究论文发展脉络
Chat Paper
正在生成论文摘要