State of health prediction for li-ion batteries with end-to-end deep learning
JOURNAL OF ENERGY STORAGE(2023)
摘要
State of health prediction for li-ion batteries is one of the hottest research topics of electric vehicles. The complexity of the capacity degradation mechanism makes it possible for deep learning methods to replace modelling and experimental methods. An end-to-end model based on a convolutional autoencoder and bidirectional LSTM combined with data interpolation and augmentation is proposed. First, key fused timing features, such as voltage, current, and temperature, are extracted directly from raw data through a convolutional autoencoder. Then, the fused features are used as the input of the bi-directional LSTM network with dropout technology and a fully connected layer to map to the battery state of health. Finally, the approach is demonstrated on the public NASA and Oxford battery datasets, and the experimental results show that the proposed method predicts capacity degradation well and that the mean absolute error is within 2 %, even when the capacity fluctuates greatly, thus proving its effectiveness.
更多查看译文
关键词
Li-ion batteries,State of health,Convolutional autoencoder,Bi-directional LSTM,Data interpolation and augmentation
AI 理解论文
溯源树
样例
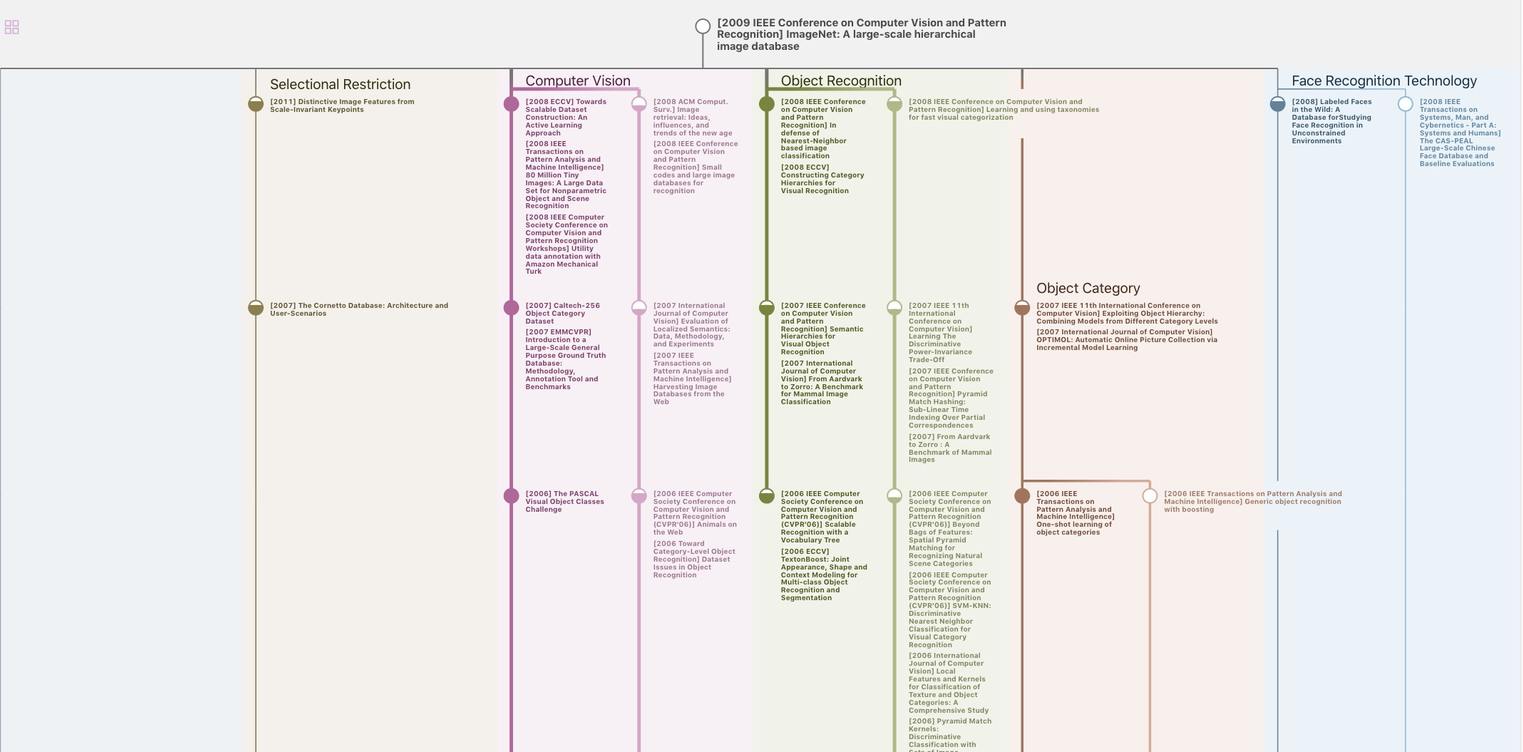
生成溯源树,研究论文发展脉络
Chat Paper
正在生成论文摘要