The amalgamation of wavelet packet information gain entropy tuned source and system parameters for improved speech emotion recognition
SPEECH COMMUNICATION(2023)
摘要
This paper proposes a three-stage feature selection algorithm by exploring the wavelet packet (WP) decompo-sition, statistical, and Information Gain (IG) feature ranking algorithm based on high IG entropy for the auto-matic classification of speech emotions (SE). Effective frame-level vocal tract systems and the excitation source parameters are initially extracted from a few significant WP sub-bands containing emotionally relevant infor-mation based on Eigenvalue decomposition. Further, Several intelligent amalgamations of the derived optimal feature vectors are formed for improved performance in a low-dimensional feature space. The fundamental argument is that in case the identification errors of any system subjected to individual feature streams transpire at separate points, there exists a probability that the inclusion of complementary information can nullify a few of these inaccuracies by increasing the available information. The models of the Cost-Sensitive-Decision Tree (CS -DT), Support Vector Machine (SVM), and Decision Tree (DT) have been validated and tested with the proposed setup for their efficacy. Results indicate the superiority of the proposed algorithms compared to other published articles cited in the literature with the CS-DT outperforming others. The proposed low-dimensional amalgam-ation vectors have witnessed more than 20% improvement in recognition performance with greater speed of response, savings in cost, and lower F-rank hence is a significant achievement in this direction.The link to compute the Hurst parameter for the feature amalgamation is available at (https://www.math works.com/matlabcentral/fileexchange/9842-hurst-exponent).
更多查看译文
关键词
Speech emotion recognition, Wavelet packet decomposition, Source and system parameters, Information gain, Cost savings, Friedman ranking
AI 理解论文
溯源树
样例
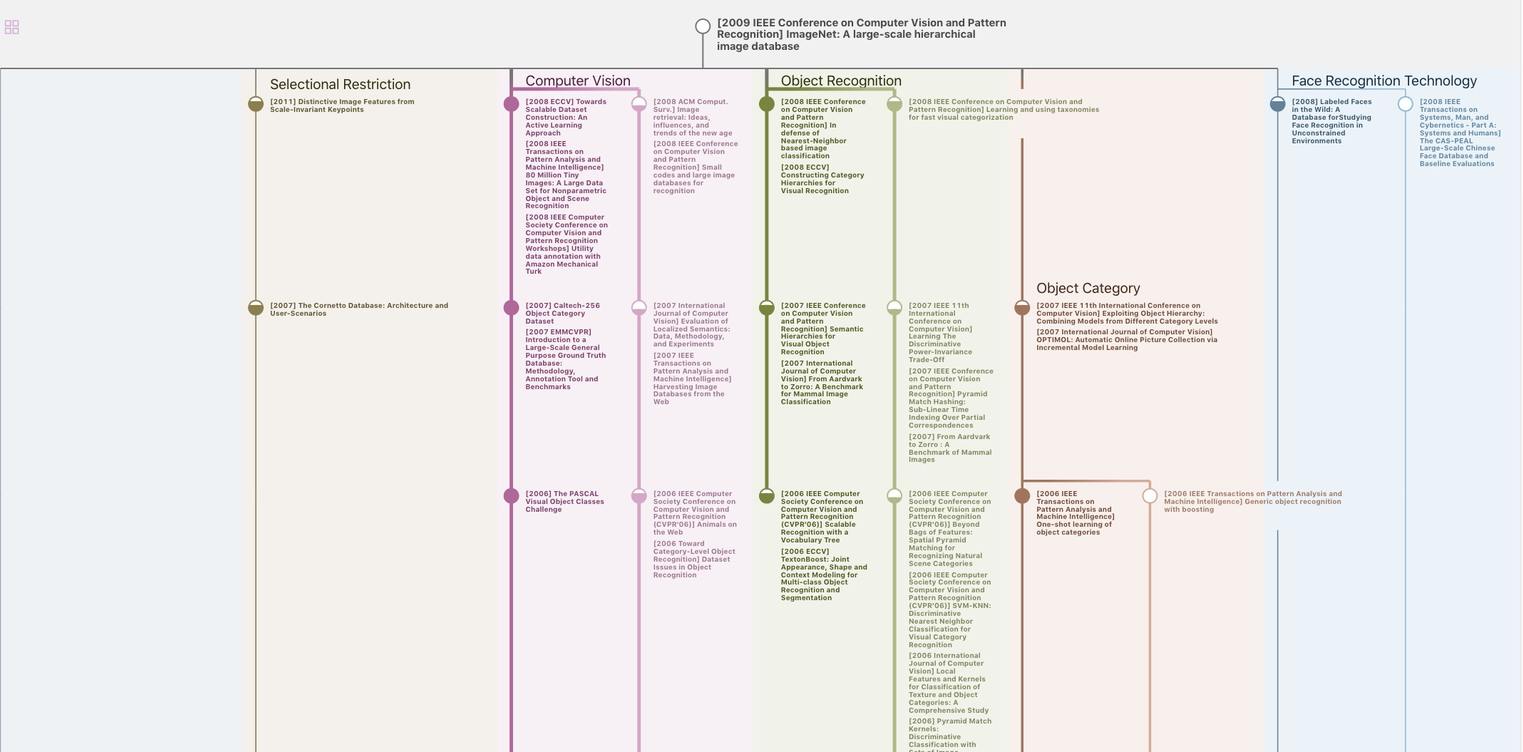
生成溯源树,研究论文发展脉络
Chat Paper
正在生成论文摘要