SWDPM: A Social Welfare-Optimized Data Pricing Mechanism
CoRR(2023)
摘要
Data trading has been hindered by privacy concerns associated with user-owned data and the infinite reproducibility of data, making it challenging for data owners to retain exclusive rights over their data once it has been disclosed. Traditional data pricing models relied on uniform pricing or subscription-based models. However, with the development of Privacy-Preserving Computing techniques, the market can now protect the privacy and complete transactions using progressively disclosed information, which creates a technical foundation for generating greater social welfare through data usage. In this study, we propose a novel approach to modeling multi-round data trading with progressively disclosed information using a matchmaking-based Markov Decision Process (MDP) and introduce a Social Welfare-optimized Data Pricing Mechanism (SWDPM) to find optimal pricing strategies. To the best of our knowledge, this is the first study to model multi-round data trading with progressively disclosed information. Numerical experiments demonstrate that the SWDPM can increase social welfare 3 times by up to 54\% in trading feasibility, 43\% in trading efficiency, and 25\% in trading fairness by encouraging better matching of demand and price negotiation among traders.
更多查看译文
关键词
Pricing Mechanism,Social Security,Markov Decision Process,Pricing Model,Pricing Strategy,Optimal Price,Match Demands,Price Negotiation,Uniform Price,Numerical Simulations,Time Step,Learning Rate,Collective Action,Optimal Function,Long Short-term Memory,Reward Function,Digital Era,Minimum Unit,Trade Data,Reasonable Price,Consideration Of Dynamics,Fine-tuning Strategy,Round Of Simulations,Pricing Problem,Action-value Function,Price Incentives
AI 理解论文
溯源树
样例
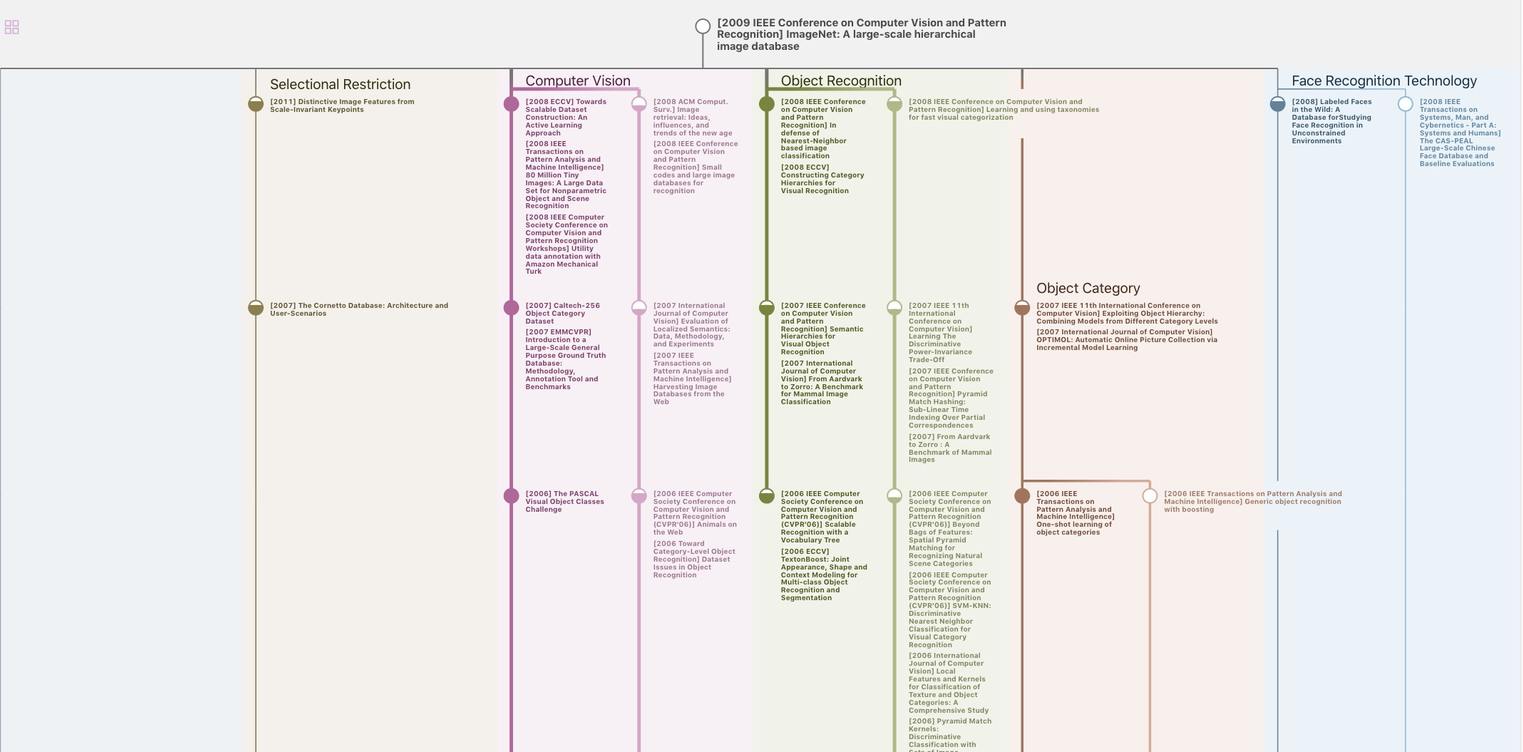
生成溯源树,研究论文发展脉络
Chat Paper
正在生成论文摘要