Cooperative Multi-Agent Reinforcement Learning: Asynchronous Communication and Linear Function Approximation.
CoRR(2023)
摘要
We study multi-agent reinforcement learning in the setting of episodic Markov decision processes, where many agents cooperate via communication through a central server. We propose a provably efficient algorithm based on value iteration that can simultaneously allow asynchronous communication and guarantee the benefit of cooperation with low communication complexity. Under linear function approximation, we prove that our algorithm enjoys a $\tilde{\mathcal{O}}(d^{3/2}H^2\sqrt{K})$ regret upper bound with $\tilde{\mathcal{O}}(dHM^2)$ communication complexity, where $d$ is the feature dimension, $H$ is the horizon length, $M$ is the total number of agents, and $K$ is the total number of episodes. We also provide a lower bound showing that an $\Omega(dM)$ communication complexity is necessary to improve the performance through collaboration.
更多查看译文
关键词
reinforcement learning,communication,multi-agent
AI 理解论文
溯源树
样例
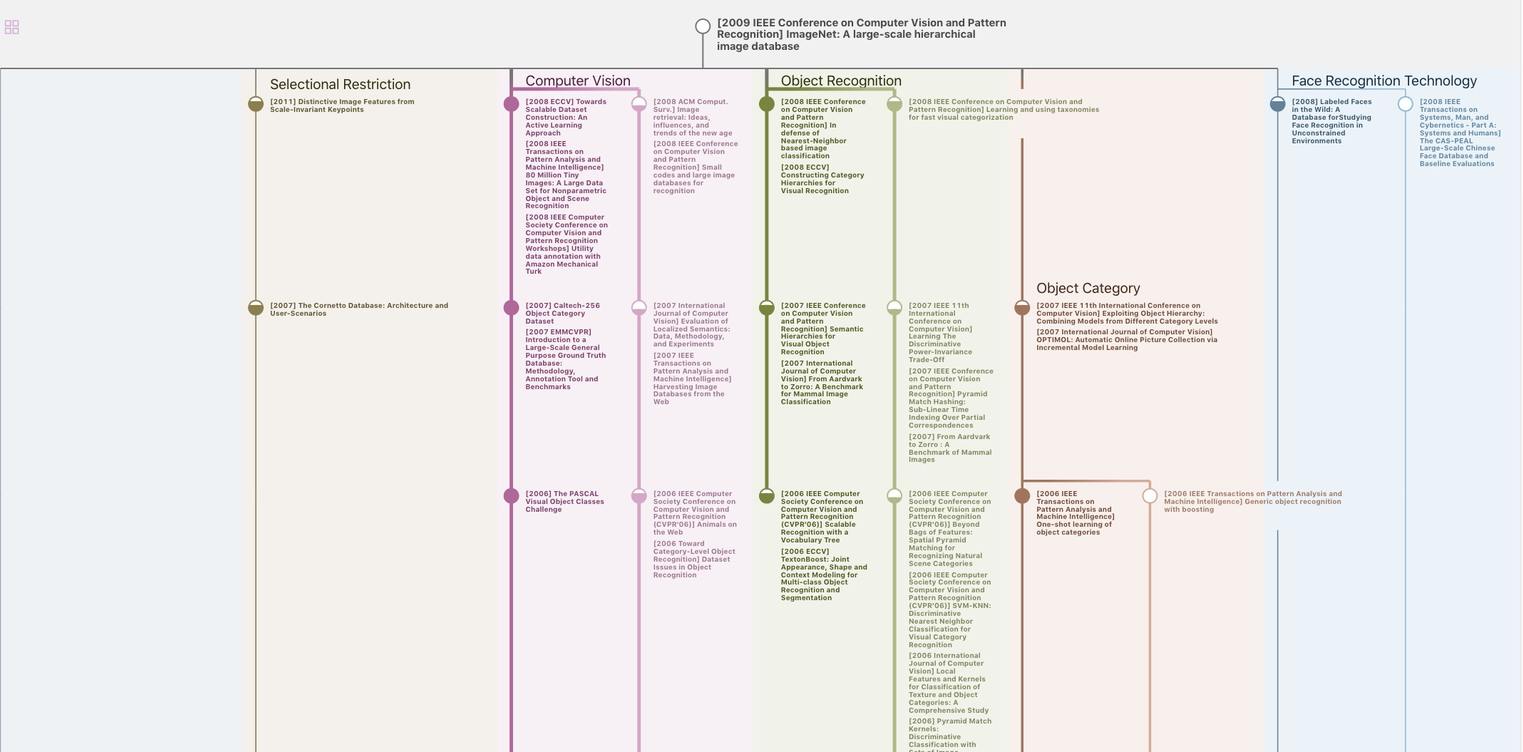
生成溯源树,研究论文发展脉络
Chat Paper
正在生成论文摘要