Using a Bayesian-Inference Approach to Calibrating Models for Simulation in Robotics
CoRR(2023)
摘要
In robotics, simulation has the potential to reduce design time and costs, and lead to a more robust engineered solution and a safer development process. However, the use of simulators is predicated on the availability of good models. This contribution is concerned with improving the quality of these models via calibration, which is cast herein in a Bayesian framework. First, we discuss the Bayesian machinery involved in model calibration. Then, we demonstrate it in one example: calibration of a vehicle dynamics model that has low degree-of-freedom (DOF) count and can be used for state estimation, model predictive control, or path planning. A high fidelity simulator is used to emulate the "experiments" and generate the data for the calibration. The merit of this work is not tied to a new Bayesian methodology for calibration, but to the demonstration of how the Bayesian machinery can establish connections among models in computational dynamics, even when the data in use is noisy. The software used to generate the results reported herein is available in a public repository for unfettered use and distribution.
更多查看译文
关键词
calibrating models,simulation,robotics,bayesian-inference
AI 理解论文
溯源树
样例
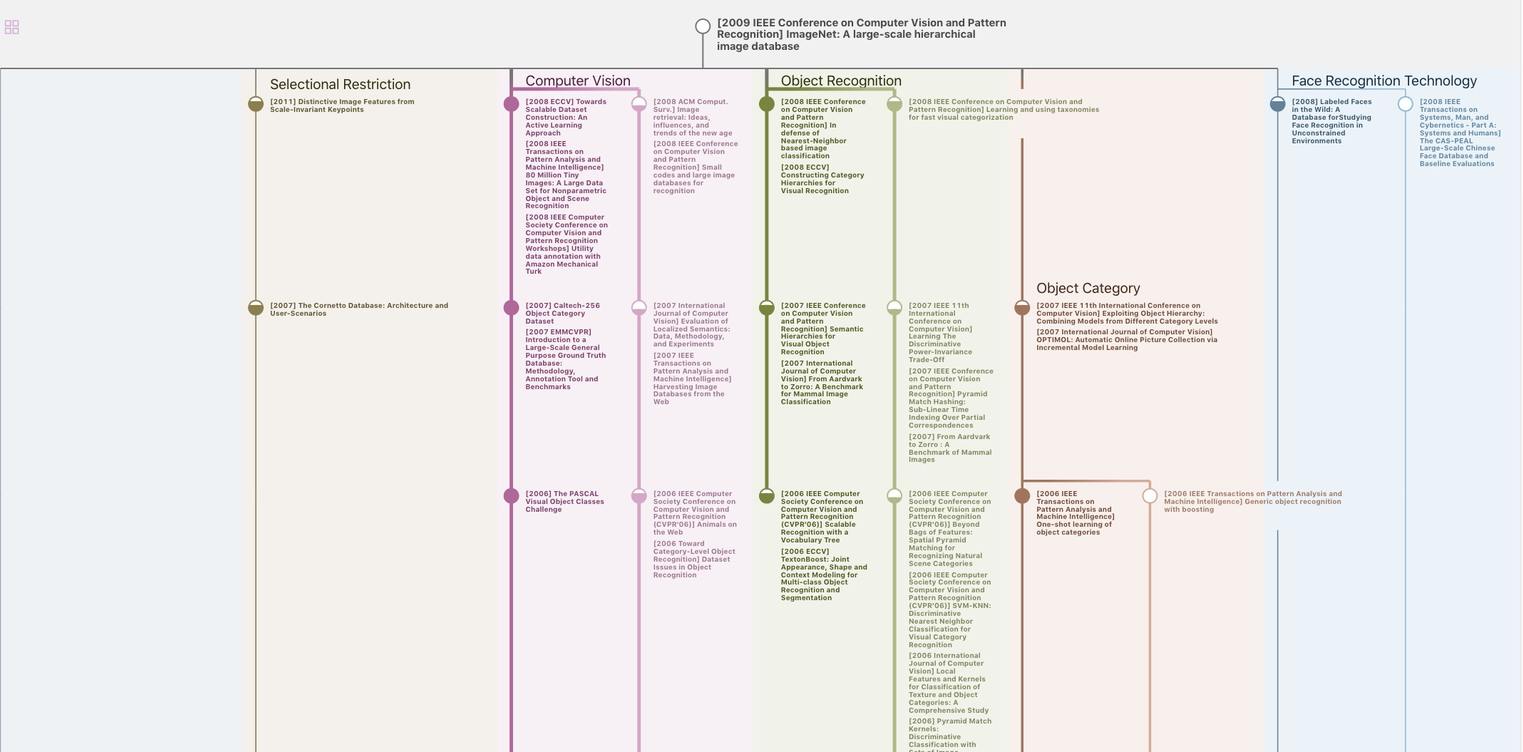
生成溯源树,研究论文发展脉络
Chat Paper
正在生成论文摘要