Few-shot News Recommendation via Cross-lingual Transfer
摘要
The cold-start problem has been commonly recognized in recommendation systems and studied by following a general idea to leverage the abundant interaction records of warm users to infer the preference of cold users. However, the performance of these solutions is limited by the amount of records available from warm users to use. Thus, building a recommendation system based on few interaction records from a few users still remains a challenging problem for unpopular or early-stage recommendation platforms. This paper focuses on solving the few-shot recommendation problem for news recommendation based on two observations. First, news at diferent platforms (even in diferent languages) may share similar topics.Second, the user preference over these topics is transferable across diferent platforms. Therefore, we propose to solve the few-shot news recommendation problem by transferring the user-news preference from a many-shot source domain to a few-shot target domain. To bridge two domainsthat are even in diferent languages and without any overlapping users and news, we propose a novel unsupervised cross-lingual transfer model as the news encoder that aligns semantically similar news in two domains. A user encoder is constructed on top of the aligned news encoding and transfers the user preference from the source to target domain. Experimental results on two real-world news recommendation datasets show the superior performance of our proposed method on addressing few-shot news recommendation, comparing to the baselines. The source code can be found at https://github.com/taichengguo/Few-shot-NewsRec .
更多查看译文
AI 理解论文
溯源树
样例
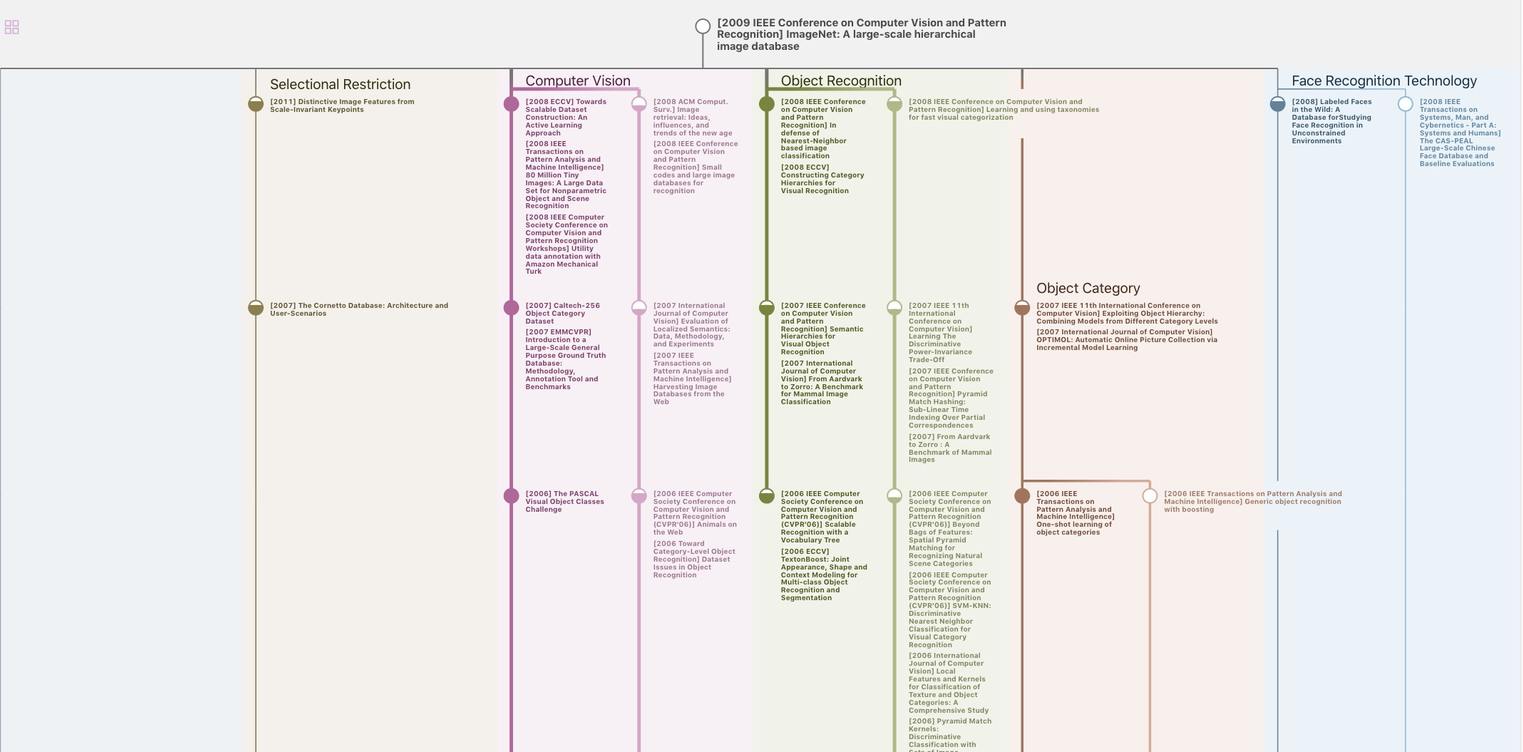
生成溯源树,研究论文发展脉络
Chat Paper
正在生成论文摘要