ACTNet: A Dual-Attention Adapter with a CNN-Transformer Network for the Semantic Segmentation of Remote Sensing Imagery
Remote sensing(2023)
摘要
In recent years, the application of semantic segmentation methods based on the remote sensing of images has become increasingly prevalent across a diverse range of domains, including but not limited to forest detection, water body detection, urban rail transportation planning, and building extraction. With the incorporation of the Transformer model into computer vision, the efficacy and accuracy of these algorithms have been significantly enhanced. Nevertheless, the Transformer model’s high computational complexity and dependence on a pre-training weight of large datasets leads to a slow convergence during the training for remote sensing segmentation tasks. Motivated by the success of the adapter module in the field of natural language processing, this paper presents a novel adapter module (ResAttn) for improving the model training speed for remote sensing segmentation. The ResAttn adopts a dual-attention structure in order to capture the interdependencies between sets of features, thereby improving its global modeling capabilities, and introduces a Swin Transformer-like down-sampling method to reduce information loss and retain the original architecture while reducing the resolution. In addition, the existing Transformer model is limited in its ability to capture local high-frequency information, which can lead to an inadequate extraction of edge and texture features. To address these issues, this paper proposes a Local Feature Extractor (LFE) module, which is based on a convolutional neural network (CNN), and incorporates multi-scale feature extraction and residual structure to effectively overcome this limitation. Further, a mask-based segmentation method is employed and a residual-enhanced deformable attention block (Deformer Block) is incorporated to improve the small target segmentation accuracy. Finally, a sufficient number of experiments were performed on the ISPRS Potsdam datasets. The experimental results demonstrate the superior performance of the model described in this paper.
更多查看译文
关键词
remote sensing,semantic segmentation,transformer,adapter
AI 理解论文
溯源树
样例
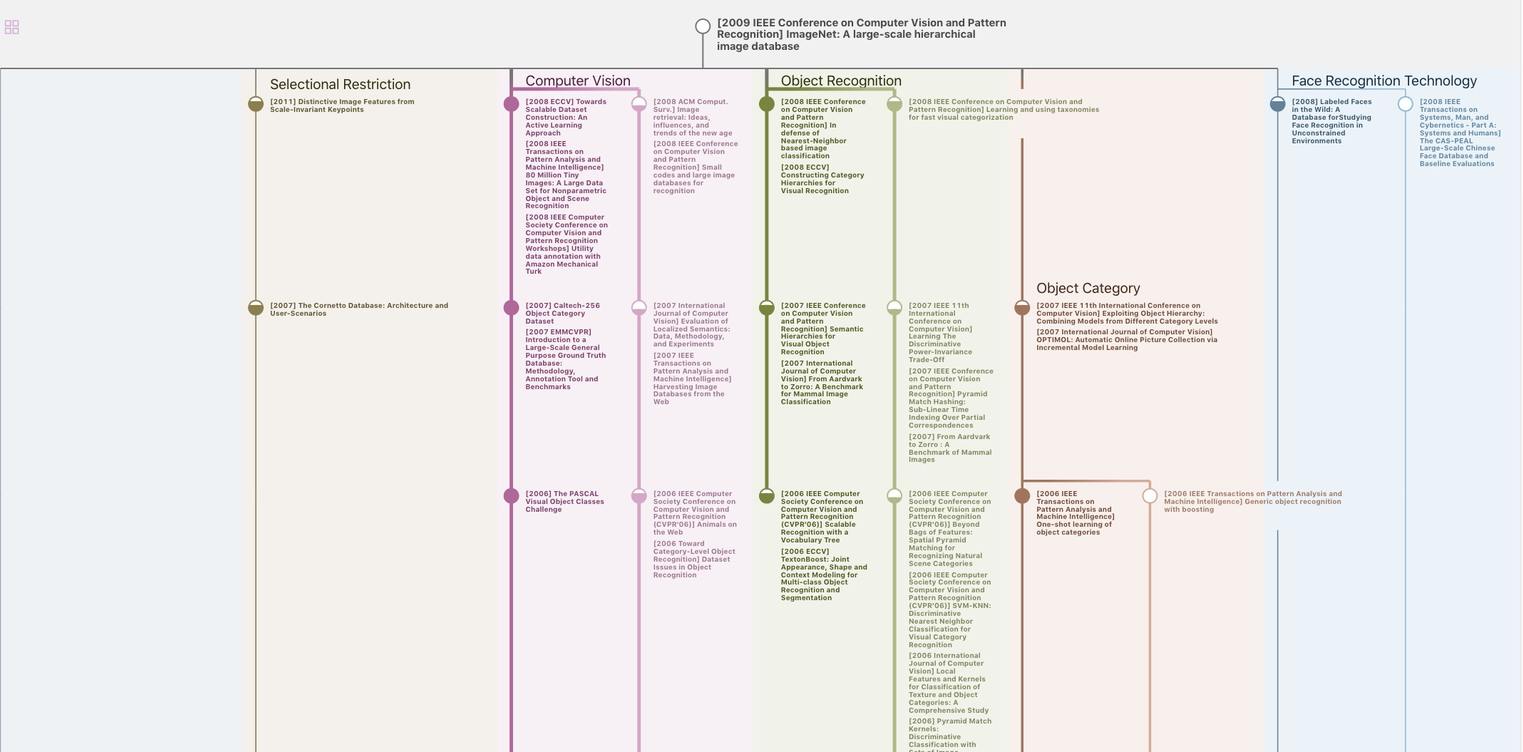
生成溯源树,研究论文发展脉络
Chat Paper
正在生成论文摘要